All published articles of this journal are available on ScienceDirect.
Integrated Bioinformatics Approach for Disclosing Autophagy Pathway as a Therapeutic Target in Advanced KRAS Mutated/Positive Lung Adenocarcinoma
Abstract
Background:
Lung cancer is the leading cause of cancer-related deaths, accounting for 1.8 million deaths (18%). Nearly 80%-85% of lung cancer cases are non-small cell lung cancers (NSCLC). One of the most frequent genetic mutations in NSCLC is the Kirsten Rat Sarcoma Oncogene Homolog (KRAS) gene mutation. In recent years, autophagy has drawn substantial attention as a potential pathway that can be targeted in cancer driven by KRAS gene mutation to efficiently improve the therapeutic profile of different treatments.
Methods:
In this study, we have investigated the potential of targeting the autophagy pathway as a treatment approach in advanced KRAS-mutated lung adenocarcinoma using gene expression data from The Cancer Genome Atlas Lung Adenocarcinoma (TCGA-LUAD) project.
Results:
Compared to KRAS wild-type lung adenocarcinoma, there were found 11 differentially expressed autophagy-related genes (DEARGs), with 5 upregulated and 6 downregulated DEARGs (threshold of adjusted p-value <0.05).
Conclusion:
These DEARGs can be investigated as potential genes that can be targeted by different autophagy inhibitors.
1. INTRODUCTION
Among non-small cell lung cancer (NSCLC) alterations, Kirsten Rat Sarcoma Oncogene Homolog (KRAS) gene mutations are the most prevalent [1]. The KRAS gene belongs to the rat sarcoma (RAS) gene family and encodes a small enzyme that hydrolyzes guanosine triphosphate (GTPases) that activates signaling pathways, including RAF-MEK-ERK, PI3K-AKT-mTOR, and RALGDS-RA. Mutated RAS isoforms vary among different types of tumors. In lung, pancreatic, and colon cancer, KRAS is most commonly mutated, while NRAS is more prevalent in melanoma and HRAS in bladder cancer. A mutation in RAS typically entails a single base substitution that stabilizes GTP binding and activates the ERK signaling pathway. Mutations in the KRAS gene affect how the KRAS protein switches between active and inactive states. This leads to uncontrolled cell division as well as an increase in resistance to chemotherapy and biotherapies. As a distinct subset of oncogene-derived tumors, KRAS mutant tumors represent a heterogeneous group of diseases that are different both from a biological and clinical perspective (response to treatment). In most cases, amino acid substitutions detected are from Gly12 to Ala, Cys, Asp, or Val. Through modulation of oncogenic downstream effectors, aberrantly mutated KRAS has been proven to play a crucial role in the initiation and maintenance of cancer [2]. Additionally, KRAS has been demonstrated to be a negative indicator of lung cancer prognosis [3]. As a result of KRAS’s high affinity for GTP and its lack of clear binding pockets for inhibitors, it was previously thought to be an “undruggable” target. Considering the difficulty of directly targeting KRAS oncoproteins, alternative therapeutic approaches have been explored, such as targeting KRAS expression, processing, upstream regulators, or downstream effectors. Over the course of the last few years, two covalent target inhibitors of KRAS-G12C protein have been approved (adagrasib and sotorasib); however, as with other kinase inhibitors, they are limited by the emergence of resistance. Moreover, the KRAS-G12C mutation encompasses only 40-50% of patients with KRAS mutations, which means that almost 50% of patients with other KRAS mutations remain untreated [4].
Despite KRAS being the most prevalent oncogene in NSCLC, to date, no targeted therapies have been approved for first-line treatment of metastatic NSCLC. The most recent European and American guidelines [5, 6] recommend immunotherapy or platinum doublet therapy with immune checkpoint inhibitors (ICIs) as first-line treatments. There is, however, a small subset of patients who respond to immunotherapy, and few are able to fully recover. Furthermore, the efficacy of platinum-based anticancer drugs and drugs targeting KRAS downstream signaling molecules (the RAS/MEK/ERK pathway) is also limited by the emergence of resistance mechanisms. Possible resistance mechanisms include activating cell survival pathways, such as autophagy.
Depending on the stage and tissue type of the tumor, autophagy has both pro- and anti-tumor functions. As a quality control mechanism, autophagy has a tumor-suppressive function in early carcinogenesis. This enables it to protect the cell by sequestering and eliminating defective cellular components, such as damaged mitochondria, and by maintaining cellular homeostasis. At advanced stages of cancer, autophagy, on the other hand, can promote tumor growth [7]. Stressful conditions, such as starvation, organelle damage, loss of proteostasis, and hypoxia, induce pro-survival autophagy. It is also possible for some anti-cancer treatments to induce pro-survival autophagy.
Even in the fed state, tumors and tumor cells, especially those driven by oncogenes, such as KRAS, upregulate autophagy and rely on it for survival and malignancy [8]. It is, therefore, possible to suppress tumors by inhibiting increased autophagy in these tumors. Several preclinical studies [9-11] have demonstrated that KRAS mutant lung cancer has a high level of basal autophagy, making autophagy inhibition a therapeutic option in KRAS-driven tumors.
A growing body of clinical evidence points toward a renewed interest in inhibiting autophagy in KRAS-driven cancer as an efficient way to improve MEK or ERK inhibitors’ therapeutic profiles. According to this evidence, KRAS-mutant tumors rely on autophagy for survival when the MAPK pathway is inhibited, and blocking autophagy in conjunction with MEK or ERK kinase may have therapeutic benefits in patients with KRAS-mutated pancreatic ductal adenocarcinoma, NRAS-mutated melanoma, or BRAF-mutated colorectal cancer [12-14].
A number of research groups have attempted to investigate the role of autophagy-related genes (ARGs)/autophagy biomarkers (based on gene expression signatures) in cancers, including lung adenocarcinomas, using various bioinformatics approaches [15-22]. To the best of our knowledge, no bioinformatics analysis has been conducted to probe the role of the ARGs in advanced KRAS mutant lung adenocarcinomas.
Research is needed on the role of autophagy in cancer growth, prevention, survival, anticancer treatments, and drug resistance in different cancers, including NSCLC. We aimed to explore the potential of targeting the autophagy pathway as a treatment for advanced KRAS-mutated NSCLC.
2. METHODS
2.1. Acquisition of Autophagy-related Gene Sets
ARGs were obtained from the Human Autophagy Database (HADb) (http://autophagy. lu/autophagy.html), REACTOME and GO biological process in the Molecular Signatures Database (MSigDB) (https://www.gsea-msigdb .org/gsea/msigdb/). The three gene sets were combined and incorporated into a list of ARGs after overlapping genes were removed. The ARGs list ultimately included 703 genes when it was constructed.
2.2. Acquisition of Gene Expression, Clinical, and Somatic Mutation Data
Our analysis was based on data retrieved from The Cancer Genome Atlas Lung Adenocarcinoma (TCGA-LUAD) project [23] using the TCGAbiolinks R/Bioconductor package [24], which can access the National Cancer Institute (NCI) Genomic Data Commons (GDC) through its GDC Application Programming Interface (API). Gene expression data were retrieved as an R object using three main functions: GDCquery (search for the needed data), GDCdownload (download the needed data), and GDCprepare (transform the needed data into a summarizedExperiment object). The three functions were used sequentially. Arguments (screening/search factors) used in GDCquery included a GDC project (TCGA-LUAD), data.category (Transcriptome Profiling), data.type (Gene Expression Quantification), workflow.type (STAR – Counts), experimental.strategy (RNA-Seq), and sample.type (Primary Tumor). Gene expression data in the SummarizedExperiment object were accessed using the assay accessor. There are many individual files in the downloaded gene expression data, including one for each tissue sample. A single file containing gene expression profiles for all the tissue samples was generated by extracting the relevant information from these individual files. Each row of this file contains gene expression information for a particular gene in all samples, and each column contains gene expression data for a particular tissue sample. Gene names are listed in the first column, and TCGA sample IDs are listed in the first row. The data were annotated in a human hg38 gene standard track reference transcript.
Clinicopathological features, such as information about demographics, exposures, diagnosis, and treatment, were obtained using GDCquery_clinical. Patients with advanced stages (stage IIIA, IIIB, and IV), according to the American Joint Committee on Cancer (AJCC) pathologic tumor staging, were only included.
Maftools Bioconductor package [25] was used to summarize, analyze, and visualize the public Mutation Annotation Format (MAF) file, which contains somatic mutation information. As an exclusion criterion, mutations appearing in different samples belonging to the same patient were not included in our study. Samples with advanced stages that were previously filtered based on the AJCC staging were also divided into two cohorts according to KRAS mutation status (mutated or wild-type).
2.3. Identification of Differential Expression of the Autophagy-related Gene Set and Enrichment Analysis
We used the DESeq2 package of R [26] to identify the differentially expressed genes (DEGs) between KRAS+ & KRAS wild-type in the TCGA-LUAD. It uses the raw counts and models the normalization inside the Generalized Linear Model (GLM). The standard differential expression analysis was performed using the function DESeq. The Benjamini-Hochberg method was applied to adjust p-values, and we considered adjusted p-value <0.05 as the cutoff criterion for DEGs identification. The intersection of DEGs from the TCGA-LUAD and ARGs was considered the set of significant differentially expressed autophagy-related genes (DEARGs) for further analysis. The MA plot and volcano plot, as well as the heatmap for the DEGs in KRAS+ and KRAS wild-type tissues, were used for graphical representation. Visualization of the volcano plot and heatmap of the DEGs were implemented using ggplot2 [27], while the MA plot was implemented using the plotMA function in DESeq2.
2.4. Application of Gene Set Enrichment Analysis (GSEA)
The Gene Ontology (GO) (including molecular function (MF), cellular component (CC), and biological process (BP)) and Kyoto Encyclopedia of Genes and Genomes (KEGG) enrichment analyses were performed on the DEARGs and all DEGs using the “clusterProfiler” R package [28] to reveal significantly enriched signaling pathways in order to better explore the biological interpretation of the DEARGs.
2.5. Construction of Protein-protein Interactions (PPI)
All DEARGs were analyzed using the STRING (Search Tool for the Retrieval of Interacting Genes) online tool [29] to construct a PPI with a cut-off criterion of combined score ≥ 0.4 (medium confidence), and visualized using Cytoscape (v3.9.1) [30].
3. RESULTS
3.1. TCGA Gene Expression Data
We explored the LUAD open access data set, which consists of 60660 genes and 585 samples. These samples include 59 normal tissue samples, 2 recurrent tumors, as well as 537 primary tumors. Each tissue sample is represented by an individual file in the downloaded data set. Using all the individual files, we compiled gene expression profiles for all tissues into one single file. After that, we removed all samples related to pathological stages other than advanced ones. The final file included only 108 samples.
The downloaded MAF file included 616 samples with 16642 genes. From the MAF file, we extracted samples with missense KRAS mutations and divided the 108 samples into two groups according to their mutation status (KRAS+ vs. KRAS wild-type). Of the 108 samples that were included in our analysis, 29 samples were KRAS+.
3.2. Identification, Enrichment Analysis, and PPI Network Construction of the DEARGs
The expression data of 23275 genes were obtained using DESeq2; of which, 730 DEGs met our selection criteria (adjusted p-value <0.05, including 236 up-regulated and 494 down-regulated genes). The MA, volcano plots, and heatmap for the DEGs are displayed in Fig. (1). The TCGA sample IDs included in the heatmap are provided in the Supplemental file.
ARGs that were differentially expressed between KRAS+ and KRAS wild-type tissues were evaluated, leading to the identification of 11 DEARGs, including 5 up-regulated DEARGs and 6 down-regulated DEARGs, as presented in Table 1.
Gene Symbol | Protein Name | Log-2 Fold Change | padj |
---|---|---|---|
PEX3 | Peroxisomal Biogenesis Factor 3 | -0.4234596 | 1.809798e-02 |
LEPR | Leptin Receptor | 0.8461635 | 4.695786e-02 |
FOXO3 | Forkhead Box O3 | -0.4741128 | 1.882466e-02 |
HTR2B | 5-Hydroxytryptamine Receptor 2B | -1.5210204 | 1.363774e-02 |
GATA4 | GATA Binding Protein 4 | -4.1864786 | 6.485733e-06 |
UFC1 | Ubiquitin-Fold Modifier Conjugating Enzyme 1 | 0.4146483 | 2.223836e-02 |
MCL1 | MCL1 Apoptosis Regulator | 0.4845785 | 7.926286e-03 |
CHMP7 | Charged Multivesicular Body Protein 7 | 0.3696904 | 2.207912e-02 |
RAB39B | Ras-related Protein Rab-39B | -1.0831825 | 1.607209e-02 |
TMEM74 | Transmembrane Protein 74 | -1.5126451 | 2.678979e-02 |
TUBA4B | Tubulin Alpha 4b | 1.6730556 | 2.372014e-02 |
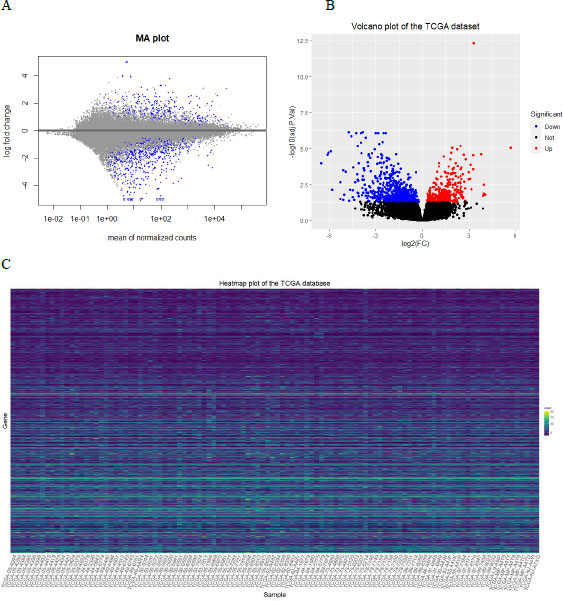
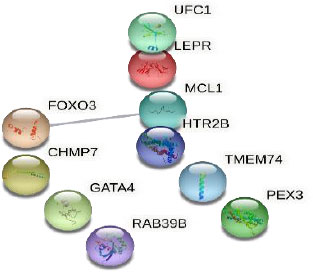
Term ID | Term Description | Observed Gene Count | Background Gene Count | FDR | Matching Proteins |
---|---|---|---|---|---|
GO:0010506 | Regulation of autophagy | 6 | 340 | 7.42e-05 | HTR2B, LEPR, GATA4, MCL1, RAB39B, FOXO3 |
GO:0010507 | Negative regulation of autophagy | 4 | 82 | 0.00046 | HTR2B, LEPR, GATA4, MCL1 |
GO:1903201 | Regulation of oxidative stress-induced cell death | 3 | 67 | 0.0133 | GATA4, MCL1, FOXO3 |
KEGG analysis and GO annotations were also performed for the 11 DEARGs to have an idea about the biological pathways in which the DEARGs are enriched. As expected, we did not get insights from these analyses due to the small number of DEARGs. However, analyzing the PPIs network constructed from the 11 genes, using the STRING database (http://string-db.org), indicated 3 GO-terms to be significantly enriched: regulation of autophagy, negative regulation of autophagy, and regulation of oxidative stress-induced cell death. Table 2 summarizes the three enriched terms.
The established network involved 10 nodes and one edge (Fig. 2). The single edge was between MCL1 Apoptosis Regulator (MCL1) and Forkhead Box O3 (FOXO3), with an interaction score of 0.556 (medium confidence). The two genes were found to have opposite actions: MCL1 inhibits apoptosis, while FOXO3 promotes apoptosis.
4. DISCUSSION
This study used the TCGA-LUAD database to examine associations between ARGs and KRAS+ phenotype for the purpose of identifying genes that can be targeted by autophagy inhibitors in advanced stages. First, we screened TCGA-LUAD for differentially expressed genes. Based on the adjusted p-value of 0.05, 730 DEGs were identified. In addition, we constructed a list of 703 ARGs derived from the HADb and the MSigDB. 11 DEARGs were found to be common between the ARGs and the DEGs, including Peroxisomal Biogenesis Factor 3 (PEX3), Leptin Receptor (LEPR), FOXO3, 5-Hydroxytryptamine Receptor 2B (HTR2B), GATA Binding Protein 4 (GATA4), Ubiquitin-Fold Modifier Conjugating Enzyme 1 (UFC1), MCL1, Charged Multivesicular Body Protein 7 (CHMP7), Ras-related Protein Rab-39B (RAB39B), Transmembrane Protein 74 (TMEM74), and Tubulin Alpha 4b (TUBA4B). The down-regulated genes included PEX3, FOXO3, HTR2B, GATA4, RAB39B, and TMEM74, while the up-regulated genes included LEPR, UFC1, MCL1, CHMP7, and TUBA4B. Using the STRING database, the 11 genes were mostly enriched in the regulation of autophagy, negative regulation of autophagy, and regulation of oxidative stress-induced cell death. Duan et al. reported, among 366 ARGs in lung adenocarcinoma, 83 DEARGs to have more than 1.5 expression fold difference, with 33 upregulated and 50 downregulated DEARGs. From the DEARGs, 10 genes were significantly associated with overall survival (OS) in LUAD patients, and were identified as an autophagy-related signature [15].
Literature was searched to explore what other researchers reported regarding the DEARGs in lung cancer. High expression levels of LEPR, UFC1, and MCL1 were found to be associated with tumor progression in lung cancer [31-33]. Higher expression of leptin and LEPR was observed in tissues of NSCLC than in normal lung tissue [34]. While low expression of FOXO3 and GATA4 was associated with poor prognosis [35, 36]. Xq28 chromosome contains the RAB39B gene, which encodes the RAB39B protein that belongs to the Rab protein superfamily [37]. There are two types of Rab proteins: those that promote or suppress tumor growth and development. In lung cancer, Rab3d and Rab5 are overexpressed and are associated with cell migration and invasion [38, 39]. On the contrary, RAB37 showed anti-metastatic activity against NSCLC [40]. RAB39B was found to be up-regulated in gastric carcinoma tissues as compared to its non-cancerous counterparts [41]. No information regarding the role of RAB39B in lung cancer was found. However, RAB39A (RAB39B isoform) was shown to promote cancer stemness and tumorigenesis [42]. Interestingly, our study identified the downregulation of RAB39B in KRAS+ NSCLC, which has not been previously described in the literature.
Although our current study provides insights into the potential role the identified DEARGs might play in facilitating the discovery of potential therapeutic targets in the studied population, it has certain limitations that should be mentioned. First, the identified DEARGs were not validated using external datasets. Secondly, in vitro and in vivo studies are needed to investigate the activity of different autophagy inhibitors in targeting these genes in advanced KRAS-mutated lung adenocarcinomas. One of the autophagy inhibitors that we are particularly interested in is hydroxychloroquine (HCQ). When combined with either antiangiogenic agents or cytotoxic agents, HCQ may increase antitumor activity significantly. HCQ was shown to be effective at inhibiting autophagy in multiple trials involving anticancer therapies, including chemotherapy and radiation therapy [8, 43-46].
CONCLUSION
The current study determined 11 DEARGs in KRAS mutated lung adenocarcinoma, which can be targeted by different autophagy inhibitors.
LIST OF ABBREVIATIONS
NSCLC | = Non-small Cell Lung Cancer |
KRAS | = Kirsten Rat Sarcoma Oncogene Homolog |
RAS | = Rat Sarcoma |
ICIs | = Immune Checkpoint Inhibitors |
ARGs | = Autophagy-Related Genes |
HADb | = Human Autophagy Database |
MSigDB | = Molecular Signatures Database |
TCGA-LUAD | = The Cancer Genome Atlas Lung Adenocarcinoma |
NCI | = National Cancer Institute |
GDC | = Genomic Data Commons |
API | = Application Programming Interface |
AJCC | = American Joint Committee on Cancer |
MAF | = Mutation Annotation Format |
DEGs | = Differentially Expressed Genes |
GLM | = Generalized Linear Model |
DEARGs | = Differentially Expressed Autophagy-Related Genes |
GSEA | = Gene Set Enrichment Analysis |
GO | = Gene Ontology |
MF | = Molecular Function |
CC | = Cellular Component |
BP | = Biological Process |
KEGG | = Koyoto Encyclopedia of Genes and Genomes |
PPI | = Protein-protein Interactions |
STRING | = Search Tool for the Retrieval of Interacting Genes |
MCL1 | = MCL1 Apoptosis Regulator |
FOXO3 | = Forkhead Box O3 |
PEX3 | = Peroxisomal Biogenesis Factor 3 |
LEPR | = Leptin Receptor |
HTR2B | = 5-Hydroxytryptamine Receptor 2B |
GATA4 | = GATA Binding Protein 4 |
UFC1 | Ubiquitin-Fold Modifier Conjugating Enzyme 1 |
CHMP7 | = Charged Multivesicular Body Protein 7 |
RAB39B | = Ras-related Protein Rab-39B |
TMEM74 | = Transmembrane Protein 74 |
TUBA4B | = Tubulin Alpha 4b |
OS | = Overall survival |
HCQ | = Hydroxychloroquine |
ETHICS APPROVAL AND CONSENT TO PARTICIPATE
Not applicable.
HUMAN AND ANIMAL RIGHTS
No humans or animals were used in the studies that are the basis of this research.
CONSENT FOR PUBLICATION
Not applicable.
AVAILABILITY OF DATA AND MATERIALS
All the data and supportive information are provided within the article.
FUNDING
None.
CONFLICT OF INTEREST
The author declares no potential conflicts of interest with respect to the research, authorship, and/or publication of this article.
ACKNOWLEDGEMENTS
The author acknowledges the role of the office of Scientific Affairs and Research (OSAR) at King Hussein Cancer Center in supporting and facilitating this project.