All published articles of this journal are available on ScienceDirect.
Investigation of Drought and Salinity Tolerance Related Genes and their Regulatory Mechanisms in Arabidopsis (Arabidopsis thaliana)
Abstract
Background:
The development of genome microarrays of the model plant; Arabidopsis thaliana, with increasing repositories of publicly available data and high-throughput data analysis tools, has opened new avenues to genome-wide systemic analysis of plant responses to environmental stresses.
Objective:
To identify differentially expressed genes and their regulatory networks in Arabidopsis thaliana under harsh environmental condition.
Methods:
Two replications of eight microarray data sets were derived from two different tissues (root and shoot) and two different time courses (control and 24 hours after the beginning of stress occurrence) for comparative data analysis through various bioinformatics tools.
Results:
Under drought stress, 2558 gene accessions in root and 3691 in shoot tissues had significantly differential expression with respect to control condition. Likewise, under salinity stress 9078 gene accessions in root and 5785 in shoot tissues were discriminated between stressed and non-stressed conditions. Furthermore, the transcription regulatory activity of differentially expressed genes was mainly due to hormone, light, circadian and stress responsive cis-acting regulatory elements among which ABRE, ERE, P-box, TATC-box, CGTCA-motif, GARE-motif, TGACG-motif, GAG-motif, GA-motif, GATA- motif, TCT-motif, GT1-motif, Box 4, G-Box, I-box, LAMP-element, Sp1, MBS, TC-rich repeats, TCA-element and HSE were the most important elements in the identified up-regulated genes.
Conclusion:
The results of the high-throughput comparative analyses in this study provide more options for plant breeders and give an insight into genes and cis-acting regulatory elements involved in plant response to drought and salinity stresses in strategic crops such as cereals.
1. INTRODUCTION
Plants respond to abiotic stresses in various ways to survive under harsh environmental conditions. Plants have acquired various stress tolerance mechanisms including physiological and biochemical changes allowing adaptation to changeable environmental conditions [1]. Recent advances in genome-wide analyses have revealed complex regulatory networks that control global gene expression, protein modification, and metabolite compositions. Genetic regulation and epigenetic regulation, including changes in nucleosome distribution, histone modification, DNA methylation, and npcRNAs (non-protein-coding RNA) play important roles in abiotic stress related gene networks [1]. Transcriptomics, metabolomics, bioinformatics, high-through-put DNA sequencing and high-density microarrays have enabled precise assessment of the regulatory networks contributing to abiotic stress tolerance. Such analyses have markedly increased our understanding of global plant systems in response to stressed conditions [1].
DNA microarray -a recent high-throughput gene expression technology- has been extensively used in gene expression analysis in crop plants [2-5]. Accordingly, several genes being responsive to different abiotic stresses at the transcriptional level have been studied [6-13]. The products of the stress inducible genes have been classified into two groups: one that directly protects plants against environmental stresses and another that regulates gene expression and signal transduction. Stress-inducible genes have been used to improve the stress tolerance of plants by gene transfer [6-8, 12-16]. Irrespective availability of such information about abiotic stresses responsible genes and various genomic tools, no satisfactory experimental crop plant model system is available to explore mechanisms behind stress tolerance at a molecular and systems level [17]. These targets necessitate investigation for the complete genomic sequence in the public domain, availability of easy transformation protocols, Expressed Sequence Tags (EST), microarray and proteomics data, and ideally a large set of well-characterized mutants. The availability of aforementioned information in Arabidopsis and the considerable body of physiological data on ion homeostasis [18], have made it the system of choice for molecular and system-wide plant studies of abiotic stress.
In Arabidopsis, the AtGenExpress project (http://www.arabidopsis.org/portals/expression/microarray/ATGenExpr ess.jsp) has collected thousands of transcript profiles on the basis of the Affymetrix ATH1 GeneChip that are now publicly available. This contribution has enabled the discovery of candidate genes on the basis of expression profiles in various tissues, developmental stages, and environmental conditions. Transcriptome analysis technologies have advanced to the point where high-through-put DNA sequencers and high-density microarrays such as tiling arrays are readily available [1]. The results of several studies indicated the usefulness of the microarray technology to the analysis of expression profiles in response to abiotic stresses, such as drought, cold and high-salinity [19-24].
In silico, identification and characterization of the genes in various organisms under different conditions are important due to growing data in the databases [25]. According to the importance of bioinformatic tools to analyze current mass biology data to identify key genes and their regulatory pattern to achieve abiotic stresses tolerance mechanism, herein we assessed the most important and common genes contributing to the key factors in response to abiotic stress in model plant Arabidopsis thaliana that can be used in practical plant breeding programs for generated new abiotic stress tolerant staple food crops. Another important aim of this study was to characterize the regulatory mode of abiotic tolerance responsible genes.
2. METHODS
2.1. Microarray Data Mining
The comparative analysis of the microarray data from different tissues of Arabidopsis under drought and salinity stresses was conducted. The Affymetrix raw data (.CEL) files from two independent experiments -drought stress time course (Unpublished data from Kudla Group, ME00338 (Expression Set: 1007966668)) and salt stress time course (Unpublished data from Kudla Group, ME00328 (Expression Set: 1007966888))- were retrieved from the TAIR dataset (https://www.arabidopsis.org/). The collected data were analyzed by FlexArray software version 1.6.3. In the first step, CEL data were normalized by Robust Multiarray Average (RMA) algorithm [26] and in the second step the RMA signal values were transformed into log2. In order to select Differentially Expressed Genes (DGEs), the RMA expression values were analyzed by two-sample student’s t-test and the up-regulated genes with more than 1-symmetrical raw fold changes, and down-regulated genes of less than 0-symmetrical raw fold changes were selected for further data analysis. p-values were adjusted in false discovery rate as less than <0.05.
2.2. Comparing Gene List
To compare results of microarray data analysis, the Venn diagram was used to identify the overlapped of the resulting genes (differentially expressed genes) and representing interactions among these gene sets in different tissues under both salinity and drought stresses conditions. The Venn diagram could be available in effective and free web application at http://bioinformatics.psb.ugent.be/webtools/Venn/.
2.3. Functional Annotation Analysis
Gene Ontology (GO) analysis was conducted with AgriGO database tool (http://bioinfo.cau.edu.cn/agriGO/). Singular Enrichment Analysis (SEA) was done to complete GO analysis of Arabidopsis thaliana species and the Affymetrix ATH1 genome Array was selected as a reference background. In the next step, the Fisher and Yekutieli (FDR under dependency) methods were chosen for statistical test and multi-test adjustment at 0.05 significance level, respectively. Finally, the results were described in three categories including, molecular function, biological processes and cellular components [27].
2.4. Cis-Acting Regulatory Element Analysis (CAREs Analysis)
Based on the GO analysis, the complete nucleotide sequence of 13 gene accession numbers (representative of 21 known genes from binding category) involved in transcription regulatory activity under salinity and drought stress conditions were downloaded from the National Center for Biotechnology Information (NCBI) (https://www.ncbi.nlm.nih.gov/) database. For CAREs analysis, the 1.5 kbp of 5´ upstream of each gene was retrieved from Phytozome (http://www.phytozome.net/) database and they were subjected to the PlantCare (http://bioinformatics.psb.ugent.be/webtools/plantcare/html/) database to identify common CAREs in the responsive genes for both salinity and drought stress conditions [28].
3. RESULTS AND DISCUSSION
3.1. Identification of Differentially Expressed Genes
The availability of a large volume of microarray data provides an opportunity to study the gene expression at whole-genome, gene family or single gene level and to identify the gene(s) involved in various biological processes [29].
The results of t-test for the microarray data demonstrated 2558 and 3691 gene accessions were differentially expressed in response to drought stress in root and shoot tissue, respectively. For salt stress, 9078 gene accessions in root and 5785 gene accessions in shoot tissue were differentially expressed.
Differences and cross-talk of gene expression among drought and salinity stress responses were analyzed based on Venn diagram analysis. As shown in Fig. (1), 683, 1226, 2276 and 1634 gene accessions were identified as drought root-, drought shoot-, salinity root- and salinity shoot-induced genes with higher than one-fold change induction, respectively (Fig. 1). The list and expression data of up-regulated genes in response to drought and salinity stress are available as a supplementary material (Table S1). A number of 450 gene accessions were induced in response to both drought and salinity in shoot tissue, whereas only 224 gene accessions were induced by both drought and salinity stress in root tissue (Fig. 1). These results indicate the existence of greater crosstalk between drought and salinity stress signaling processes in shoot tissue, than those in root tissue. Analysis of overlapping on the Venn diagram showed that 49 gene accessions were induced under all two stresses (Table 1), of which 0.47% (49 out of 10467 of the gene accessions) were responded to drought stress regulated by salinity stress. Therefore, it can be concluded that there is a strong correlation between the responses of plant to drought and salinity stress.
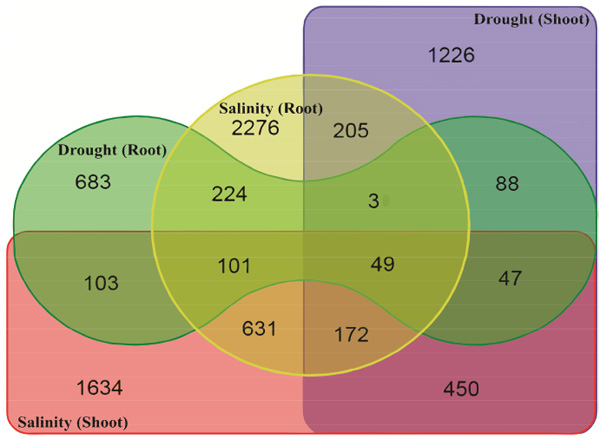
Gene Bank Acc. |
Gene symbol | Gene type | Chromosome number | Fold change | Symmetrical raw fold change | T statistic | P-value | |
---|---|---|---|---|---|---|---|---|
At3g12570 | FYD | Protein coding | 3 | 1.116 | 1.116 | 8.046 | 0.015 | |
At5g58140 | PHOT2 | Protein coding | 5 | 1.131 | 1.131 | 4.410 | 0.048 | |
PLPB | Protein coding | 2 | ||||||
PHOT1 | Protein coding | 3 | ||||||
At4g24690 | NBR1 | Protein coding | 4 | 1.155 | 1.155 | 5.904 | 0.028 | |
At2g01460 | AT2G01460 | Protein coding | 2 | 1.155 | 1.155 | 4.791 | 0.041 | |
At5g19400 | SMG7 | Protein coding | 5 | 1.156 | 1.156 | 6.016 | 0.027 | |
AT1G28260 | Protein coding | 1 | ||||||
At4g35890 | LARP1c | Protein coding | 4 | 1.163 | 1.163 | 5.449 | 0.032 | |
LARP1a | Protein coding | 5 | ||||||
LARP1b | Protein coding | 5 | ||||||
At1g23950 | AT1G23950 | Protein coding | 1 | 1.166 | 1.166 | 50.860 | 0.028 | |
At4g20930 | AT4G20930 | Protein coding | 4 | 1.167 | 1.167 | 8.359 | 0.014 | |
AT1G18270 | Protein coding | 1 | ||||||
ATARD2 | Protein coding | 4 | ||||||
At1g67300 | AT1G67300 | Protein coding | 1 | 1.179 | 1.179 | 17.386 | 0.003 | |
SGB1 | Protein coding | 1 | ||||||
At1g79610 | NHX6 | Protein coding | 1 | 1.181 | 1.181 | 12.284 | 0.007 | |
NHX5 | Protein coding | 1 | ||||||
At1g64110 | DAA1 | Protein coding | 1 | 1.181 | 1.181 | 7.058 | 0.019 | |
At5g20900 | JAZ12 | Protein coding | 5 | 1.187 | 1.187 | 4.979 | 0.038 | |
JAZ11 | Protein coding | 3 | ||||||
JAZ10 | Protein coding | 5 | ||||||
AT5G03235 | ncRNA | 5 | ||||||
At2g39720 | RHC2A | Protein coding | 2 | 1.197 | 1.197 | 6.285 | 0.024 | |
At3g51830 | SAC8 | Protein coding | 3 | 1.202 | 1.202 | 11.518 | 0.007 | |
At1g13190 | AT1G13190 | Protein coding | 1 | 1.204 | 1.204 | 5.031 | 0.037 | |
AT5G55670 | Protein coding | 5 | ||||||
At4g10610 | CID12 | Protein coding | 4 | 1.206 | 1.206 | 4.960 | 0.038 | |
CID11 | Protein coding | 1 | ||||||
At5g61810 | APC1 | Protein coding | 5 | 1.221 | 1.221 | 8.105 | 0.015 | |
APC3 | Protein coding | 5 | ||||||
At1g08000 | GATA10 | Protein coding | 1 | 1.260 | 1.260 | 6.868 | 0.021 | |
GATA11 | Protein coding | 1 | ||||||
At4g01070 | GT72B1 | Protein coding | 4 | 1.263 | 1.263 | 10.318 | 0.009 | |
At1g19190 | AT1G19190 | Protein coding | 1 | 1.264 | 1.264 | 6.476 | 0.023 | |
At2g22240 | MIPS2 | Protein coding | 2 | 1.270 | 1.270 | 5.188 | 0.035 | |
MIPS1 | Protein coding | 4 | ||||||
MIPS3 | Protein coding | 5 | ||||||
At1g69295 | PDCB4 | Protein coding | 1 | 1.323 | 1.323 | 9.209 | 0.012 | |
At4g18020 | APRR2 | Protein coding | 4 | 1.332 | 1.332 | 12.573 | 0.006 | |
At5g57050 | ABI2 | Protein coding | 5 | 1.341 | 1.341 | 4.481 | 0.046 | |
ABI1 | Protein coding | 4 | ||||||
At2g32700 | LUH | Protein coding | 2 | 1.362 | 1.362 | 5.206 | 0.035 | |
LUG | Protein coding | 4 | ||||||
At4g34460 | AGB1 | Protein coding | 4 | 1.373 | 1.373 | 60.304 | 0.001 | |
At5g02310 | PRT6 | Protein coding | 5 | 1.389 | 1.389 | 8.168 | 0.015 | |
CER3 | Protein coding | 5 | ||||||
ATE1 | Protein coding | 5 | ||||||
ATE2 | Protein coding | 3 | ||||||
At5g05600 | AT5G05600 | Protein coding | 5 | 1.398 | 1.398 | 4.726 | 0.042 | |
At3g29575 | AFP3 | Protein coding | 3 | 1.402 | 1.402 | 11.564 | 0.007 | |
NINJA | Protein coding | 4 | ||||||
TMAC2 | Protein coding | 3 | ||||||
At3g16740 | AT3G16740 | Protein coding | 3 | 1.409 | 1.409 | 5.649 | 0.030 | |
At4g05150 | AT4G05150 | Protein coding | 4 | 1.418 | 1.418 | 4.635 | 0.044 | |
At5g03490 | AT5G03490 | Protein coding | 5 | 1.423 | 1.423 | 5.427 | 0.032 | |
AT1G51210 | Protein coding | 1 | ||||||
At3g24520 | HSFC1 | Protein coding | 3 | 1.460 | 1.460 | 5.014 | 0.038 | |
AT3G24518 | ncRNA | 3 | ||||||
At5g50800 | SWEET13 | Protein coding | 5 | 1.470 | 1.470 | 5.495 | 0.032 | |
SWEET14 | Protein coding | 4 | ||||||
SWEET10 | Protein coding | 5 | ||||||
At1g30200 | AT1G30200 | Protein coding | 1 | 1.485 | 1.485 | 10.054 | 0.010 | |
At5g13740 | ZIF1 | Protein coding | 5 | 1.507 | 1.507 | 8.479 | 0.014 | |
ZIFL1 | Protein coding | 5 | ||||||
At1g79000 | HAC1 | Protein coding | 1 | 1.519 | 1.519 | 5.192 | 0.035 | |
HAC12 | Protein coding | 1 | ||||||
HAC5 | Protein coding | 3 | ||||||
HAC2 | Protein coding | 1 | ||||||
At1g17530 | TIM23-1 | Protein coding | 1 | 1.547 | 1.547 | 7.624 | 0.017 | |
TIM23-2 | Protein coding | 1 | ||||||
TIM23-3 | Protein coding | 3 | ||||||
At5g45430 | AT5G45430 | Protein coding | 5 | 1.561 | 1.561 | 5.266 | 0.034 | |
AT4G19110 | Protein coding | 4 | ||||||
At1g07750 | AT1G07750 | Protein coding | 1 | 1.574 | 1.574 | 10.959 | 0.008 | |
GRF10 | Protein coding | 1 | ||||||
AT2G28680 | Protein coding | 2 | ||||||
At3g06780 | AT3G06780 | Protein coding | 3 | 1.683 | 1.683 | 4.926 | 0.039 | |
RBP1 | Protein coding | 1 | ||||||
AT3G01895 | ncRNA | 3 | ||||||
At5g15950 | AT5G15950 | Protein coding | 5 | 1.842 | 1.842 | 9.646 | 0.011 | |
SAMDC | Protein coding | 3 | ||||||
psaC | psaC | Protein coding | - | 1.911 | 1.911 | 5.772 | 0.029 | |
nad5.1 | nad5 | Protein coding | - | 1.955 | 1.955 | 9.612 | 0.011 | |
nad4 | nad4 | Protein coding | - | 2.141 | 2.141 | 6.937 | 0.020 | |
At5g10960 | AT5G10960 | Protein coding | 5 | 2.289 | 2.289 | 10.651 | 0.009 | |
At4g30460 | AT4G30460 | Protein coding | 4 | 2.983 | 2.983 | 6.386 | 0.024 | |
RD20 | Protein coding | 2 | ||||||
RAB18 | Protein coding | 5 | ||||||
RABA1e | Protein coding | 4 | ||||||
AT1G71000 | Protein coding | 1 | ||||||
AT3G22570 | Protein coding | 3 | ||||||
At4g38680 | GRP2 | Protein coding | 4 | 3.150 | 3.150 | 8.641 | 0.013 | |
GRP2B | Protein coding | 2 | ||||||
At5g10770 | AT5G10770 | Protein coding | 5 | 3.562 | 3.562 | 230.784 | 0.001 |
Analysis of stress-down-regulated as well as stress-up-regulated genes is important in understanding molecular responses to abiotic stresses [22]. In the present study, a total of 635, 553, 3762 and 1186 gene accessions were identified as drought root-, drought shoot-, salinity root- and salinity shoot-down-regulated genes by means of microarray analysis (Fig. 2). The list and expression data on these drought and salinity stress-down-regulated gene accessions are available as a supplementary material (Table S2). A total 358 down-regulated gene accessions were observed in shoot tissue under both drought and salinity and expression of 362 number of gene accessions were decreased by both drought and salinity stress in root tissue (Fig. 2). The results indicated that 0.25% (27 out of 10645) of DEGs from both tissues showed possible crosstalk in response to drought and salinity stresses (Table 2). Based on the result presented in Figs. (1 and 2), the number of down-regulated genes was higher than the number of up-regulated ones indicating most of Arabidopsis genes have been switched off under drought and salinity stresses.
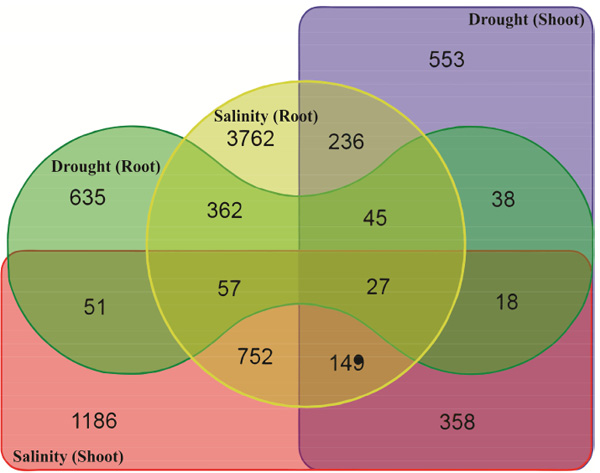
Gene Bank Acc. | Gene symbol | Gene type | Chromosome number | Fold Change | Symmetrical fold change | T statistic | P-value | |||||||
---|---|---|---|---|---|---|---|---|---|---|---|---|---|---|
At3g62930 | AT3G62930 | Protein coding | 3 | 0.484 | -2.064 | -7.144 | 0.019 | |||||||
AHBP-1B | Protein coding | 5 | ||||||||||||
WEE1 | Protein coding | 1 | ||||||||||||
AT1G03020 | Protein coding | 1 | ||||||||||||
At2g04440 | AT2G04440 | Protein coding | 2 | 0.670 | -1.493 | -16.229 | 0.004 | |||||||
At5g09650 | PPa6 | Protein coding | 5 | 0.685 | -1.460 | -4.607 | 0.044 | |||||||
IPY1 | Protein coding | - | ||||||||||||
At5g62980 | FOLB2 | Protein coding | 5 | 0.742 | -1.347 | -5.529 | 0.031 | |||||||
FOLB1 | Protein coding | 3 | ||||||||||||
At1g55490 | CPN60B | Protein coding | 1 | 0.764 | -1.309 | -5.398 | 0.033 | |||||||
Cpn60beta4 | Protein coding | 1 | ||||||||||||
Cpn60beta2 | Protein coding | 3 | ||||||||||||
Cpn60beta3 | Protein coding | 5 | ||||||||||||
Cpn60alpha2 | Protein coding | 5 | ||||||||||||
At1g31860 | AT-IE | Protein coding | 1 | 0.784 | -1.275 | -5.276 | 0.034 | |||||||
At4g38160 | pde191 | Protein coding | 4 | 0.794 | -1.260 | -4.706 | 0.042 | |||||||
AT4G38150 | Protein coding | 4 | ||||||||||||
FRS9 | Protein coding | 4 | ||||||||||||
At3g46010 | ADF1 | Protein coding | 3 | 0.799 | -1.251 | -10.853 | 0.008 | |||||||
ADF4 | Protein coding | 5 | ||||||||||||
ADF3 | Protein coding | 5 | ||||||||||||
ADF6 | Protein coding | 2 | ||||||||||||
At4g15680 | AT4G15680 | Protein coding | 4 | 0.810 | -1.234 | -5.407 | 0.033 | |||||||
AT5G18600 | Protein coding | 5 | ||||||||||||
AT4G15670 | Protein coding | 4 | ||||||||||||
At2g43920 | HOL2 | Protein coding | 2 | 0.826 | -1.210 | -13.424 | 0.006 | |||||||
HOL1 | Protein coding | 2 | ||||||||||||
At2g28000 | CPN60A | Protein coding | 2 | 0.828 | -1.208 | -7.739 | 0.016 | |||||||
Cpn60alpha2 | Protein coding | 5 | ||||||||||||
Cpn60beta2 | Protein coding | 3 | ||||||||||||
Cpn60beta3 | Protein coding | 5 | ||||||||||||
Cpn60beta4 | Protein coding | 1 | ||||||||||||
At2g31490 | AT2G31490 | Protein coding | 2 | 0.831 | -1.203 | -6.081 | 0.026 | |||||||
VAP27-1 | Protein coding | 3 | ||||||||||||
NLM1 | Protein coding | 4 | ||||||||||||
ASIL1 | Protein coding | 1 | ||||||||||||
AT1G27300 | Protein coding | 1 | ||||||||||||
AT3G16310 | Protein coding | 3 | ||||||||||||
At5g61440 | ACHT5 | Protein coding | 5 | 0.840 | -1.190 | -10.586 | 0.009 | |||||||
At1g79850 | RPS17 | Protein coding | 1 | 0.844 | -1.185 | -8.908 | 0.012 | |||||||
AT1G09927 | ncRNA | 1 | ||||||||||||
At1g76405 | AT1G76405 | Protein coding | 1 | 0.854 | -1.171 | -4.902 | 0.039 | |||||||
CSN5A | Protein coding | 1 | ||||||||||||
AT1G20816 | Protein coding | 1 | ||||||||||||
At5g24840 | AT5G24840 | Protein coding | 5 | 0.856 | -1.169 | -4.668 | 0.043 | |||||||
AT5G17660 | Protein coding | 5 | ||||||||||||
At3g25920 | RPL15 | Protein coding | 3 | 0.866 | -1.155 | -7.801 | 0.016 | |||||||
AT5G64670 | Protein coding | 5 | ||||||||||||
At4g14110 | COP9 | Protein coding | 4 | 0.867 | -1.153 | -4.897 | 0.039 | |||||||
At5g49910 | cpHsc70-2 | Protein coding | 5 | 0.872 | -1.147 | -7.714 | 0.016 | |||||||
cpHsc70-1 | Protein coding | 4 | ||||||||||||
At1g71020 | AT1G71020 | Protein coding | 1 | 0.900 | -1.111 | -4.737 | 0.042 | |||||||
MYC2 | Protein coding | 1 | ||||||||||||
MYC3 | Protein coding | 5 | ||||||||||||
CIPK3 | Protein coding | 2 | ||||||||||||
MYC4 | Protein coding | 4 | ||||||||||||
SCL30 | Protein coding | 3 | ||||||||||||
PDLP6 | Protein coding | 2 | ||||||||||||
AT1G23030 | Protein coding | 1 | ||||||||||||
AT1G03520 | Protein coding | 1 | ||||||||||||
AT4G39050 | Protein coding | 4 | ||||||||||||
AT1G15170 | Protein coding | 1 | ||||||||||||
AT5G47070 | Protein coding | 5 | ||||||||||||
AT4G35500 | Protein coding | 4 | ||||||||||||
At5g03905 | AT5G03905 | Protein coding | 5 | 0.904 | -1.107 | -5.300 | 0.034 | |||||||
CPISCA | Protein coding | 1 | ||||||||||||
At4g28660 | PSB28 | Protein coding | 4 | 0.908 | -1.101 | -8.447 | 0.014 | |||||||
At4g14410 | bHLH104 | Protein coding | 4 | 0.909 | -1.100 | -9.993 | 0.010 | |||||||
bHLH34 | Protein coding | 3 | ||||||||||||
At2g46225 | ABIL1 | Protein coding | 2 | 0.917 | -1.090 | -4.938 | 0.039 | |||||||
AT2G09710 | ncRNA | 2 | ||||||||||||
At3g49640 | AT3G49640 | Protein coding | 3 | 0.918 | -1.089 | -6.274 | 0.024 | |||||||
AT4G38890 | Protein coding | 4 | ||||||||||||
AT5G67220 | Protein coding | 5 | ||||||||||||
At1g49630 | PREP2 | Protein coding | 1 | 0.919 | -1.088 | -8.914 | 0.012 | |||||||
PREP1 | Protein coding | 3 | ||||||||||||
At2g01860 | EMB975 | Protein coding | 2 | 0.921 | -1.086 | -5.867 | 0.028 |
3.2. Functional Annotation Analysis of DGEs
The GO analysis of up-regulated common genes (49 gene accessions, Table 1) of root and shoot tissues under salinity and drought stress revealed that DEGs products were dissected into three distinct categories: biological process, molecular function and cellular component (Fig. 3). In the biological process category, the overrepresented genes belonged to the metabolic (42.86%) and cell (40.82%) processes (Fig. 3). In molecular function process, the largest group with 46.94% and 38.78% related to the binding and catalytic activity, respectively (Fig. 3). In the cellular component process, the largest group belonged to the cell (46.94%) and cell part (46.94%). To identify the functional key genes, we used non-repetitive genes distinct in each functional category.
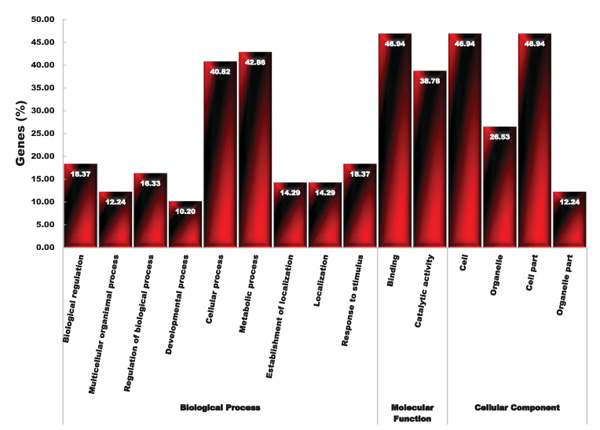
In metabolic process, the genes AT5G10770, AT2G01460, AT1G19190, AT5G03490 and AT1G51210 were identified to contribute to proteolysis, kinase, hydrolase and UDP-glucosyltransferase activity under both salinity and drought stresses. Proteome analysis has contributed significantly to understanding the plant response to abiotic stress through changes in the abundance of proteases [30]. For instance, up-regulation of different subunits of the 20S proteasome has been detected in desiccation-tolerant maize embryos during desiccation [31], leaves of drought-treated Medicago sativa plants [32], wheat stems under drought [33], and during dehydration of desiccation tolerant grass Sporobolus stapfianus [34]. On the other hand, in response to dehydration, proteins involved in proteolysis carried out by the proteasome were found to be expressed to constant levels in nuclei in the desiccation tolerant Xerophyta viscosa, probably to help maintain minimum viability in cells under stress [35]. Specific proteolysis is essential for the activation of the many enzymes and regulatory proteins during plant development and diverse environmental changes [36].
There are different products of genes with kinase activity, which typically makes them useful for abiotic stress tolerance. Among the protein kinases involved in stress signal transduction in plants are those common to all eukaryotic organisms, i.e. Mitogen-Activated Protein Kinases (MAPKs) [36-38], Glycogen Synthase Kinase3 (GSK3) [40-41], S6 Kinase (S6K) [42], Calcium-Dependent Protein Kinases (CDPKs) [43-45] and most of SNF1-related kinases (SnRKs). Also, SnRK2 family members are plant-specific serine/threonine kinases involved in plant response to abiotic stresses and abscisic acid (ABA)-dependent plant development [46]. The results of GO analysis indicated that in metabolic process, the gene AT1G19190 had hydrolase activity and controls alpha/beta-Hydrolases superfamily protein expression. Recently, a novel α/β-Hydrolase gene IbMas containing maspardin domain from α/β -hydrolase superfamily has been discovered [47]. The biological functions of α/β-hydrolase fold enzymes in organisms are widely variable and include biosynthesis, metabolism, signal transduction and gene regulation [48]. To date, several of α/β-hydrolase fold enzymes (esterase, phospholipase D and OsPOP5) have been shown to be involved in plant salt tolerance [49-52].
In cell process, GRP2, GRP2B, AT1G67300, SGB1, TIM23-1, TIM23-2 and TIM23-3 genes had function in plasma membrane, transmembrane transport and protein targeting to mitochondrion. The nad5, GT72B1, ABI2, ABI1, AT1G19190, AT5G15950, SAMDC, nad4, AT5G03490, AT1G51210, PRT6, CER3, ATE1, ATE2 and SAC8 genes in catalytic activity category had important role in response to toxin, xenobiotic catabolic process, photoinhibition, protein amino acid de-phosphorylation, osmotic stress, spermine biosynthetic process, adenosyl-methionine decarboxylase activity, ubiquitin-protein ligase activity, regulation of lipid catabolic process and phosphatidylinositol-4,5-bisphosphate 5-phosphatase activity.
For binding category HSFC1, AT3G24518, CID12, CID11, GATA10, GATA11, APC1, APC3, RHC2A, PDCB4, APRR2, GRP2, GRP2B, psaC, AT5G10770, NBR1, AT1G13190, AT5G55670, TIM23-1, TIM23-2 and TIM23-3 genes had important effect on DNA duplex unwinding, transcription factor activity, protein binding, RNA binding, mRNA binding and DNA binding protein. Among these genes, the role of AT3G24518 is noticeable because it codes a non-coding RNA. Accordingly, this gene could reflect various epigenetic regulation mechanisms i.e. histone modification and chromatin remodeling to induce a number of genes against different environmental stimuli. Furthermore, transcription factors like DRE-Binding Protein (DREB), C-repeat-Binding Factor (CBF), ABA-Binding Factor (ABF), myelocytomatosis oncogene (MYC) and myeloblastosis oncogene (MYB) [53-55] have important role on regulation of the expression of environmental stress-inducible genes. DREBs (dehydration responsive element binding) are important plant Transcription Factors (TFs) regulating the expression of many stress-inducible genes mostly in an ABA-independent manner and play a critical role in improving the abiotic stress tolerance of plants by interacting with a DRE/CRT cis-element present in the promoter region of various abiotic stress-responsive genes [56]. The protein binding is a critical part for the regulation of the genes against abiotic stresses [57].
RNA-binding proteins are important in cases such as post-transcriptional gene regulation mechanism. Most of RNA-binding proteins are plant specific with specific function. Definitely, recent research on RNA-binding proteins indicated that, beside their regulation function, they are also important for adaptation of plants to various environments. Their function is associated with the regulation of pre-mRNA splicing, polyadenylation, RNA stability and RNA export [58]. The DREB TFs contain a highly conserved AP2/ERF DNA-binding domain across the plant kingdom including Arabidopsis, rice, soybean, chickpea, tomato, tobacco, and millets [56].
Phosphorylation may be necessary for the activation of proteins under drought-stress conditions, thus enhancing the DNA-binding activity of several transcription regulators [59]. The functional gene group for DNA binding activity, plays a key role in providing tolerance to multiple stresses, generally in an ABA-independent manner through DRE/ CRT cis-elements and the AP2/ERF DNA binding domain [56].
3.3. Identification of Cares in the Promoter Site of Transcription Factors
Transcription Factors (TFs) have a critical role in response to unregular and harsh environmental condition such as abiotic stresses through regulation of gene expression. According to the results of GO analysis, 21 genes including HSFC1, AT3G24518, CID12, CID11, GATA10, GATA11, APC1, APC3, RHC2A, PDCB4, APRR2, GRP2, GRP2B, psaC, AT5G10770, NBR1, AT1G13190, AT5G55670, TIM23-1, TIM23-2 and TIM23-3 had significant role in binding activity as TF elements. In order to identify the presence of most presented CAREs that regulated the expression of the salinity and drought responsive genes in Arabidopsis, a genomic sequence of 1.5 kbp from aforementioned genes were used in the promoter analysis. The most present CAREs in the promoter region are shown in Table (3). CAREs functions were predominantly associated with light response, hormonal regulation and stress response that were detected in the upstream of DGEs under drought and salinity stress conditions in Arabidopsis (Table 3). The hormonal regulatory elements included Methyl jasmonate (MeJA), Gibberellin (GA), Ethylene (ET) and abscisic acid (ABA) responsive such as ABRE, ERE, P-box, TATC-box, CGTCA-motif, GARE-motif and TGACG-motif elements were presented in the majority of DGEs under salinity and drought stress conditions (Table 3). Plants perceive and respond adaptively to abiotic stress imposed by salt, cold, drought and wounding and the adaptive process is controlled mainly by the phytohormone, abscisic acid (ABA), which acts as an endogenous messenger in the regulation of the plant’s water status [60]. ABA is generated as a signal during a plant’s life cycle to control developmental processes such as seed germination. The ABA function can target specifically guard cells for the induction of stomatal closure but may also involve in signaling pathway for adjustment towards severe water shortage conditions. Since various stresses induce ABA synthesis, it is considered as a plant stress hormone [61, 62]. Various transcription factors are known to regulate the ABA-responsive gene expression [61, 63]. ABA induced expression often relies on the presence of cis-acting element called ABRE element (ABA-responsive element) [6, 8, 64]. ABRE element regulates the dehydration and salinity responses in model plants like Arabidopsis and rice [65]. In addition, this element has been involved in abiotic stress responses in Arabidopsis [66] and Brassica napus [67].
Cis-Acting Regulatory Element (CARE) | Function | ||
---|---|---|---|
5UTR Py-rich stretch | cis-acting element conferring high transcription levels | ||
ABRE | cis-acting element involved in the abscisic acid responsiveness | ||
ARE | cis-acting regulatory element essential for the anaerobic induction | ||
Box 4 | part of a conserved DNA module involved in light responsiveness | ||
CAAT-box | common cis-acting element in promoter and enhancer regions | ||
CGTCA-motif | cis-acting regulatory element involved in the MeJA-responsiveness | ||
circadian | cis-acting regulatory element involved in circadian control | ||
ERE | ethylene-responsive element | ||
GAG-motif | part of a light responsive element | ||
GA-motif | part of a light responsive element | ||
GATA-motif | part of a light responsive element | ||
GARE-motif | gibberellin-responsive element | ||
G-Box | cis-acting regulatory element involved in light responsiveness | ||
G-box | cis-acting regulatory element involved in light responsiveness | ||
GT1-motif | light responsive element | ||
GCN4_motif | cis-regulatory element involved in endosperm expression | ||
HSE | cis-acting element involved in heat stress responsiveness | ||
I-box | part of a light responsive element | ||
LAMP-element | part of a light responsive element | ||
MBS | MYB binding site involved in drought-inducibility | ||
P-box | gibberellin-responsive element | ||
Skn-1-motif | cis-acting regulatory element required for endosperm expression | ||
Sp1 | light responsive element | ||
TATA-box | core promoter element around -30 of transcription start | ||
TATC-box | cis-acting element involved in gibberellin-responsiveness | ||
TCA-element | cis-acting element involved in salicylic acid responsiveness | ||
TC-rich repeats | cis-acting element involved in defense and stress responsiveness | ||
TCT-motif | part of a light responsive element | ||
TGACG-motif | cis-acting regulatory element involved in the MeJA-responsiveness |
Jasmonates (JAs) [Jasmonic Acid (JA) and methyl jasmonates (MeJAs)] are known to participate in various physiological processes [68]. An important phytohormone, JA (Jasmonic Acid) and its methyl ester, methyl jasmonates (MeJAs), are derivatives of the fatty acid metabolism [69-71]. According to the results of this study, TGACG and CGTCA motives are involved in Methyl jasmonate (MeJA) response that affects the regulation of plant defense against biotic stresses. In a study the role of these motives in abiotic stress tolerance has been highlighted [72]. The recently published research indicated both JA and MeJA promote plant defense against different stresses [73]. In the case of ethylene (ET), the Ethylene Response Factor Protein, JERF3, has been demonstrated to activate the expression of oxidative genes, resulting in lower accumulation of ROS and, in turn, enhanced adaptation to drought, freezing, and salt stresses in tobacco [74]. The effect of ethylene as stress hormones controlling adaptation to abiotic and biotic stress has been reported [75, 76]. In the present study, the results indicated that these elements interfere with drought and salinity stress responses. The presence of these hormonal regulatory elements in the majority of expressed genes helps to expand our knowledge to better understand the function of hormone responsive genes under different abiotic stresses in Arabidopsis and consequently in other plant species. The central role of the gibberellin (GA) class of growth hormones in the response to abiotic stress is becoming increasingly evident [77]. Reduction in GA levels and signaling has been shown to contribute to plant growth restriction on exposure to several stresses, including cold, salt and osmotic stress. Conversely, increased GA biosynthesis and signaling promote growth in plant escape responses to shading and submergence. In several cases, GA signaling has also been linked to stress tolerance [77]. The interaction of the GA-signaling molecule -DELLA- with the components of the signaling pathways for the stress hormone jasmonic acid indicates the role of an additional mechanism by which GA signaling may integrate multiple hormone signaling pathways in response to abiotic stresses [77].
The results of this study revealed that the light responsive elements such as GAG-motif, GA-motif, GATA- motif, TCT-motif, GT1-motif, Box 4, G-Box, G-box, I-box, LAMP-element and Sp1 were located in the promoter region of expressed salinity and drought responsive genes (Table 3). The result of CARE analysis demonstrated that the light responsive elements were mainly controlled by the responsive genes. These regulatory elements play a fundamental role in the regulation of transcriptional activity [78]. GATA-box, G-box, Box-4, GAG, GAP, GA-motif and I-box are known as light responsive cis-elements (LREs). They have been found in the regulatory region of light-regulated genes, with the GATA-motif and I-box being the most prominent among them, apparently essential for light-controlled transcriptional activity [79, 80]. G-Box element is one of the parts of the response mechanism against abiotic stresses in Arabidopsis [81]. GAG motif plays a major role in abiotic stress in tobacco [82]. In addition, the role of G-Box element under drought stress on wheat ancestors has been reported [83].
The circadian clock in plants coordinates the responses to multiple simultaneous environmental challenges as the sessile plant cannot avoid. With respect to this issue, several circadian elements were found in drought and salinity responsive genes in this study (Table 3). These circadian CAREs control photoperiod-responsive genes. On the one hand, these genes are involved in opening and stomata closure, regulation of flowering, water use efficiency and response to hormone and light especially in leaves. They affect transpiration and regulate the biological processes and are involved in metabolic and physiological networks that are essential for growth, reproduction and proper response to abiotic and biotic stresses [84-86]. The role of this element to improve Arabidopsis tolerance against salinity, drought and cold stress has been confirmed [87]. Therefore, our study confirmed the role of circadian regulatory element in response to salinity and drought stresses in Arabidopsis.
The stress responsive elements such as MBS were presented in the majority of responsive genes (Table 3). MBS is a binding site for MYB transcription factors, which control many abiotic stress responses [88]. Genetic and molecular approaches have facilitated extensive functional characterization of MYB domain proteins, particularly the R2R3-type members in various plant species, including rice, maize and soybean. A genome wide comparative analysis of MYB genes and their expression in Arabidopsis and rice have indicated the potential role of several MYB domain proteins in plant stress responses [89]. Several members of R2R3-type MYB transcription factors are involved in the regulation of phenylpropanoid pathway and production of various secondary metabolic compounds under abiotic stress conditions in plants. In addition, most studies declared the role of MBS element against drought stress in common Bean [90] and maize [91].
TC-rich repeats and TCA-element were another CAREs that are present in the majority of responsive genes (Table 3). The TC-rich repeats and TCA-element have been associated with stress responses according to previous reports [92-95].
In the present study, the HSE element has been found in the regulatory region of responsive genes showing a possible role of this CARE in response to salinity and drought stress in Arabidopsis. TF is an HSF that binds to HSE cis-acting elements in the promoter of stress-inducible genes and plays central roles in the acquisition of plant tolerance against abiotic stresses [96].
Furthermore, ARE regulatory elements are essential for the induction of anaerobic respiration (Table 3). The role of these elements against abiotic stresses is limited, although our study indicates the possibility of the role of these elements in tolerance against drought and salinity stresses.
Moreover, other elements such as GCN4_motif and Skn-1 motives were present in the majority of responsive genes specifically in endosperm (Table 3). Although our study shows that these elements could be considered as regulatory candidates against abiotic stresses, their function and contribution are still unknown.
CONCLUSION
In the present study, the expression pattern and regulation network of Arabidopsis as genetic model crop under salinity and drought stresses were highlighted. According to higher number of co-expressed gene accessions in shoot (450 genes) with respect to root tissue (224 genes) under both salinity and drought stresses it can be concluded that there was a great crosstalk between drought and salinity stress signaling processes in shoot tissue. Also, there was a strong correlation between the responses of plant to drought and salinity stress. Furthermore, a number of 13 gene accessions had important effect on binding activity such as DNA duplex unwinding and transcription factor activity. Among genes, the role of AT3G24518 was remarkable because it codes a non-coding RNA which suggests some possible epigenetic regulation mechanisms like histone modification and chromatin remodeling. The result of CARE analysis indicated that hormonal regulatory and light responsive elements were the most presented CAREs in the majority of DGEs under salinity and drought stress conditions. Overall, these kinds of studies on model plants and such high-throughput analyses give an insight into the genetic architecture of important plants like cereals under stress condition and consequently help plant breeders to perform comprehensive breeding programs in order to develop abiotic stress- tolerant cultivars.
LIST OF ABBREVIATIONS
npcRNAs | = non-protein-coding RNAs |
EST | = Expressed Sequence Tags |
RMA algorithm | = Robust Multiarray Average algorithm |
DGEs | = Differentially Expressed Genes |
GO | = Gene Ontology |
SEA | = Singular Enrichment Analysis |
FDR | = False Discovery Rate |
NCBI | = National Center for Biotechnology Information |
CAREs | = Cis-Acting Regulatory Element |
MAPKs | = Mitogen-Activated Protein Kinases |
GSK3 | = Glycogen Synthase Kinase3 |
CDPKs | = Calcium-Dependent Protein Kinases |
S6K | = S6 kinase |
SnRKs | = SNF1-Related Kinases |
DREB | = Dehydration Responsive Element Binding |
CBF | = C-repeat-Binding Factor |
ABA | = Abscisic Acid |
ABF | = Abscisic Acid-Binding Factor |
MYC | = Myelocytomatosis oncogene |
MYB | = myeloblastosis oncogene |
TFs | = Transcription Factors |
MeJA | = Methyl Jasmonate |
GA | = Gibberellin |
ET | = Ethylene |
ETHICS APPROVAL AND CONSENT TO PARTICIPATE
Not applicable.
HUMAN AND ANIMAL RIGHTS
No Animals/Humans were used for studies that are base of this research.
CONSENT FOR PUBLICATION
Not applicable.
CONFLICT OF INTEREST
The authors declare no conflict of interest, financial or otherwise.
ACKNOWLEDGEMENTS
The authors gratefully acknowledge the support of this study by Shiraz University.