All published articles of this journal are available on ScienceDirect.
Decoding Retinoblastoma: Unveiling Gene Networks and Potential Targets through In Silico Analysis
Abstract
Background
Retinoblastoma is an aggressive cancer whose majority of patients are infants and children below the age of five. Approximately 80% of the total patients of retinoblastoma reside in low-to-middle-income countries like India. Lack of public and medical awareness and the absence of significant and regular clinical trials to test and authenticate new potential treatments impede the process of treating retinoblastoma. Attempts have been made to establish an effective way to diagnose retinoblastoma early so that it can be controlled in time, but so far, no significant success has been documented on that front. Moreover, recent strategies include computational and informatics solutions to identify potential targets at a genetic level to alter the expression of defective proteins in human subjects.
Aim
The main aim of the current study is to unravel the potential targets of Retinoblastoma, an aggressive pediatric cancer, utilizing an in silico network biology approach.
Methods
In the present study, we have utilized the gene network analysis approach to identify hub genes that affect the expression in the human system. We developed the Protein – Protein Interaction network utilizing 158 genes extracted from the NCBI OMIM database and identified 15 key genes, which were then subjected to metascape analysis to identify pathways and processes that affect and prioritize genes based on their significance scores. We were able to identify the following target genes: RBBP4, TFDP1, and RBBP7.
Result
RBBP4, TFDP1, and RBBP7 were identified as the most novel target genes against retinoblastoma after gene network and enrichment analysis.
Conclusion
Our in-silico network analysis unveiled the intricate mechanisms behind the progression of retinoblastoma by dissecting 158 associated genes in humans. Thus, this work not only illuminates the underlying dynamics of the disease but also offers a promising avenue for intervention.
1. INTRODUCTION
Retinoblastoma is a malignant tumor of the eye which mainly affects infants and young children [1]. It is identified to be very lethal if left untreated and also has a hereditary effect linked to it [2, 3]. In high-income countries, retinoblastoma is considered to be a curable disease with a disease-free survival rate of almost 100% [3]. But in low- and middle-income countries, the prognosis of retinoblastoma cancer is often very sober, and it is in these countries where 80% of the global cases are documented [4, 5]. Prognosis and survival can only be achieved when there is early diagnosis and appropriate treatment of such fatal maladies [6]. With almost 90% and even more than that of the affected pediatric patients living in underprivileged countries, it is a very troublesome situation that children die of a potentially curable disease that has a high survival rate [7, 8].
Retinoblastoma is caused by the RB1 gene inactivation, which is commonly known as a “Tumor suppressor gene” [9]. The constitutional loss of one allele of the RB1 gene makes an individual predisposed to cancer. However, retinoblastoma is only developed once the second allele is also lost from the developing retinal cells [10]. In 1971, Knudson suggested the two-hit hypothesis. According to Knudson, retinoblastoma requires two chromosomal abnormalities to occur. In hereditary retinoblastoma, the first hit is a germinal mutation, which is inherited and found in all cells. Retinoblastoma is caused by the second hit, which develops in somatic retinal cells. As a result, inherited cases are more likely to develop non-ocular malignancies like osteosarcoma. Both hits in unilateral sporadic retinoblastoma originate during retinal development and are somatic mutations [11, 12]. As a result, there is no danger of developing secondary non-ocular malignancies. The signs and symptoms of retinoblastoma include leukocoria (white pupil), strabismus, pain in the eyes, and loss of vision [5]. India has the biggest burden of retinoblastoma, with almost 1500 cases documented annually, which makes it number one in the Asia-Pacific region and also globally [5]. In the present study, we have attempted to understand the disease mechanism by using a systems biology approach through the construction of a gene network of retinoblastoma genes and performing a meta-analysis on the key genes obtained from the network to identify potent target genes for retinoblastoma in humans (Homo sapiens).
2. METHODOLOGY
In the present study, we have utilized a systems biology approach for target gene identification. This whole study was performed with one focal point, i.e., the identification of key target genes, which can be utilized in the future to suggest a possible treatment strategy for retinoblastoma. The complete workflow of this study has been summarized in Fig. (1).
2.1. Retrieval of Retinoblastoma-associated Genes
In the present study, we retrieved a total of 158 retinoblastoma-associated genes of Homo sapiens from the NCBI OMIM database [13] after immense data mining. Out of all the genes present in the database, these 158 were taken as they were present in humans and were involved in the pathogenesis of retinoblastoma tumors as per the description and literature available. All the genes were compiled in an Excel sheet for further analysis.
2.2. Construction and Analysis of the PPI Network
After the retrieval of genes, a PPI (Protein-Protein Interaction) network of all 158 retinoblastoma-associated genes was made using the STRING 11.0 database [14]. We constructed a network based on the high confidence score of 0.007, which means that only interactions with a high level of confidence in the network were considered a reliable PPI network. STRING (Search Tool for the Retrieval of Interacting Genes) allowed an in-depth study of network interaction by providing several features. STRING was also used for obtaining direct and indirect human protein-protein interaction networks. The constructed network was analyzed and visualized with the help of Cytoscape v3.8.1 plug-in network analyzer [15].
2.3. Metascape Analysis of Key Genes
Once we obtained the top 15 key genes from the PPI network, we moved on to the next step of the study, i.e., meta-analysis of the shortlisted genes. For this purpose, we used the Metascape server (https://metascape.org). Metascape is an online web-based platform that provides users with comprehensive gene annotation and resources for their analysis. It combines functional enrichment analysis, interactome analysis gene annotation, and membership search to leverage almost 40 independent knowledgebases that too in one single portal [16].
The shortlisted genes were uploaded to the metascape portal, and then an analysis was done with all parameters kept in the default state. The obtained results were then analyzed to understand and identify the target genes for retinoblastoma.
3. RESULTS
3.1. Protein-protein Interaction Network Generation and Hub Gene Identification
The PPI network of 158 retinoblastoma-associated genes was built (Fig. 2), and after analyzing the PPI network in Cytoscape, we identified the top 15 key genes from the network based on topology parameter betweenness centrality and Node degree. Node is represented by a gene, and its interaction is known as edge. The number of edges specifies the node degree in a network. On the other hand, betweenness centrality specifies the significance of the nodes which is based on the shortest path which every node undergoes. A comprehensive list of all 15 key genes, along with their node degree and betweenness centrality values, are given in Table 1.
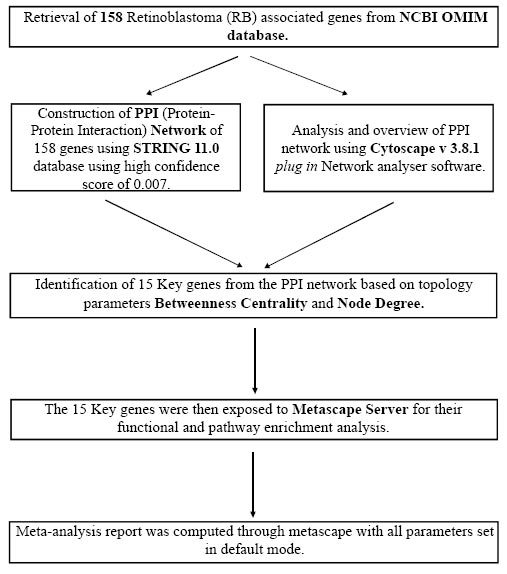
Graphical representation of the methodology adopted in this study.
S. No. | Gene Symbols | Node Degree | Betweenness Centrality |
---|---|---|---|
1. | RBBP4 | 15 | 0.05299482 |
2. | TFDP1 | 14 | 0.12652059 |
3. | RBBP7 | 13 | 0.05407063 |
4. | CTNNB1 | 13 | 0.022879413 |
5. | HDAC | 12 | 0.1086844 |
6. | RB1 | 12 | 0.10376508 |
7. | E2F6 | 12 | 0.0570658 |
8. | SMAD3 | 12 | 0.08675065 |
9. | E2F1 | 11 | 0.03806124 |
10. | CCND1 | 11 | 0.16905705 |
11. | RBL1 | 10 | 0.03036036 |
12. | CDKN2A | 9 | 0.11584578 |
13. | KRAS | 9 | 0.19192889 |
14. | RBBP5 | 8 | 0.06506864 |
15. | RBL2 | 8 | 0.00564027 |
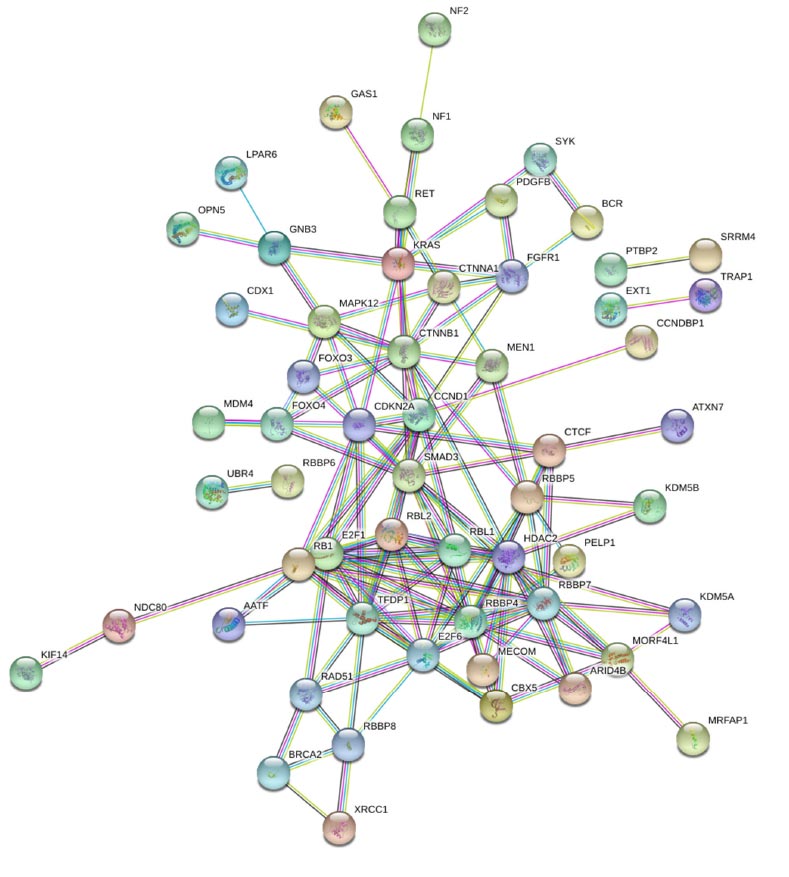
The Protein-Protein Interaction (PPI) network of retinoblastoma-associated genes constructed using STRING 11.0 database with a confidence score of 0.007.
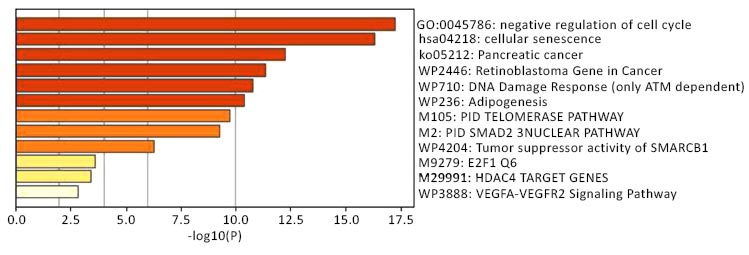
Bar graph of enriched terms across 15 key genes colored by p-values.
GO | Category | Description | Count | Log10(P) |
---|---|---|---|---|
GO:0045786 | GO Biological Processes | Negative regulation of cell cycle | 12 | -17.24 |
Hsa04218 | KEGG Pathway | Cellular senescence | 9 | -16.31 |
Ko05212 | KEGG Pathway | Pancreatic cancer | 6 | -12.27 |
WP2446 | WikiPathways | Retinoblastoma gene in cancer | 6 | -11.35 |
WP710 | WikiPathways | DNA damage response (only ATM dependent) | 6 | -10.77 |
WP236 | WikiPathways | Adipogenesis | 6 | -10.38 |
M105 | Canonical pathways | PID telomerase pathway | 5 | -9.71 |
M2 | Canonical Pathways | PID SMAD2 3nuclear pathway | 5 | -9.26 |
WP4204 | WikiPathways | Tumor suppressor activity of SMARCB1 | 3 | -6.26 |
M9279 | Transcription Factor Targets | E2F1 Q6 | 3 | -3.61 |
M29991 | Transcription Factor Targets | HDAC4 target genes | 4 | -3.43 |
WP3888 | WikiPathways | VEGFA-VEGFR2 signaling pathway | 3 | -2.84 |
3.2. Functional and Pathway Enrichment of Identified Genes and Proteins
For all the 15 key genes entered in the metascape server, pathway and functional enrichment analysis was performed using GO Biological Processes (in-built). All genes in the genome were used as enrichment background. Terms with a p-value of <0.01, minimum count of 3, and an enrichment factor >1.5 were collected and grouped into clusters based on their membership similarities. Fig. (3) represents a bar graph summary of the clusters colored based on their p-values. From the graph, it is quite evident that the maximum genes (12 out of 15) are involved in negative regulation of the cell cycle, 9 genes are involved in cellular senescence, and 6 genes among the total 15 entered are Retinoblastoma genes. The completed count and p-values are given in Table 2.
4. DISCUSSION
Retinoblastoma, a malignancy affecting the retina, represents a complex interplay of genetic and environmental factors. Despite its lethality, early detection offers a high chance of cure, underscoring the critical need for advanced diagnostic and therapeutic approaches. Presently, chemotherapy stands as the primary treatment modality, yet the evolving landscape of ophthalmological advancements promises a paradigm shift towards more effective and preventive strategies.
To unlock the full potential of these innovations, a comprehensive understanding of the molecular underpinnings of retinoblastoma is imperative. Harnessing the power of systems biology, we delved into the intricate network of genes and pathways implicated in the progression of the disease. Leveraging data from literature and authoritative databases like NCBI OMIM, a systematic exploration of the genes associated with retinoblastoma ensues. Central to this endeavor is the construction of protein-protein interaction (PPI) networks, a cornerstone of systems biology. Employing resources like the STRING database, we delineated the intricate web of molecular interactions underlying retinoblastoma pathogenesis. Through advanced analytical tools, such as Cytoscape, the PPI network was scrutinized, unveiling key nodes pivotal in disease progression. Topological parameters, such as betweenness centrality and node degree, emerged as crucial metrics in identifying pivotal genes within the network. Through rigorous analysis, a cadre of the top 15 key genes was elucidated, representing potential targets for therapeutic intervention. To unravel the functional significance of these genes, further enrichment analysis was conducted using platforms like the Metascape server.
This integrative approach not only sheds light on the molecular landscape of retinoblastoma but also unveils novel avenues for therapeutic exploration. By deciphering the intricate interplay of genetic elements driving disease progression, researchers pave the way for personalized interventions and targeted therapies, heralding a new era in the management of retinoblastoma and offering hope for improved patient outcomes [17, 18].
In the network, RBBP4, TFDP1, and RBBP7 were the top three hub genes, and the enrichment analysis also showed that these were a part of the cluster of genes representing retinoblastoma-associated genes. Therefore, these genes play an important role in the pathogenesis of retinoblastoma in humans. They also are associated with negative regulation of the cell cycle.
RBBP4 and RBBP7 are highly homologous proteins and contain WD40 motifs, and several studies have claimed that they are associated with various types of cancers [19]. Targeting these proteins could be very effective in curing retinoblastoma as both the network analysis and enrichment analysis show their potent role in retinoblastoma in humans. TFDP1, on the other hand, is a transcription factor. It is also implicated to be associated with certain hepatocellular and other potentially lethal categories of cancers. Thus, the metascape analysis for functional and pathway enrichment of the key genes from the PPI network does show compelling evidence suggesting a role of the above-mentioned three hub genes in the regulation of retinoblastoma in humans.
CONCLUSION
The in-silico network analysis of retinoblastoma associated with 158 genes in humans unfolds the underlying mechanism responsible for the progression of retinoblastoma. It also presents before us an opportunity to target the hub genes and hopefully cure them. Retinoblastoma is a very lethal disease, especially when we consider the fact that most of the patients suffering from it are infants and small children. The network analysis helps in prioritizing the key genes against an array of genes in the human system. This study has helped in understanding the disease at the genetic level, given us plausible target genes, and has presented a way forward.
Current therapeutic options help individuals with intraocular disorders save their vision and improve overall survival rates in people with extraocular diseases. However, significant morbidity is associated with plaque treatment. An improved understanding of the pathobiology of retinoblastoma could lead to new treatments, improved therapeutic outcomes with fewer long-term morbidity and mortality, and prevent secondary tumors from developing. Furthermore, translational studies have demonstrated the effectiveness of adjuvant therapy in animal models, and retinoblastoma cell lines have revealed fresh information about the disease development. Lastly, the introduction of ideally timed therapeutic drugs that operate synergistically in the control of retinoblastoma tumors will be the focus of future retinoblastoma therapy trends [20].
AUTHORS' CONTRIBUTIONS
It is hereby acknowledged that all authors have accepted responsibility for the manuscript's content and consented to its submission. They have meticulously reviewed all results and unanimously approved the final version of the manuscript.