All published articles of this journal are available on ScienceDirect.
Network Biology Approaches to Identify the Drug Lead Molecule for Neurodevelopmental Disorders in Human
Abstract
Aims:
To identify most novel drug target and lead molecule for neurodevelopmental disorder Autism, Intellectual Disability (ID) and Attention Deficit Hyperactivity Disorder (ADHD) diseases through system biology approaches
Background:
Neurodevelopmental disorders (NNDs) are disabilities associated chiefly with the functioning of the neurological system and brain. Children with neurodevelopmental disorders have difficulties with speech, behaviour, learning and other neurological functions. Systems biology is a holistic approach to enciphering the complexity of biological systems and their interactions. It opens the way to a more successful discovery of novel therapeutics.
Objective:
To identify most novel drug target and lead molecule for neurodevelopmental disorder Autism, Intellectual Disability (ID) and Attention Deficit Hyperactivity Disorder (ADHD) diseases through system biology approaches.
Methods:
A list of genes was collected from NCBI database for Autism, Intellectual Disability (ID) and Attention Deficit Hyperactivity Disorder (ADHD) diseases. STRING database and Cytoscape software was used for construction and interpreting molecular interaction in the network. 3D structure of target protein, was build and validated.The phytochemicals were identified through various research articles and filtered out by virtual screening through Molinspiration. Molecular docking analyses of known phytochemical with target proteins were performed usingAutoDock tool.
Result:
AKT1 for Autism, SNAP25 for Intellectual Disability (ID) and DRD4 for Attention Deficit Hyperactivity Disorder (ADHD) were identified as most potential drug target through network study. further the modelled structure of obtained target were undergo molecular docking study with kown phytochemicals. Based on lowest binding energy, Huperzine A for Autism and ID, Valerenic acid for ADHD found to be the most potential therapeutic molecules.
Conclusion:
Huperzine A against Autism and ID, Valerenic acid against ADHD found to be the most potential therapeutic molecules and expected to be effective in the treatment of NNDs. Phytochemicals do not have side effects so extract of these can be taken in preventive form too as these disorders occur during developmental stages of the child. Further the obtained molecule if experimentally validated would play promising role for the treatment of NDDs in human.
1. INTRODUCTION
The neurodevelopmental disorders are a group of circumstances with onset in the developmental stage [1, 2]. The disorders usually clear early in development, often before the child enters grade school, and are marked by developmental deficits that produce problems of personal, social, academic, or occupational functioning [3, 4]. Systems biology approaches can be examined as a new area of study that aims to find out biology at the system level, entailing the functional study of the structure and dynamics of cells and organisms [5].
Systems biology approaches applied to healthcare would try to identify the systems that, when altered, shift the body from a “healthy” to a “disease” state. The working hypothesis is that the elements of the biological system that are involved in the observed switch between states are specific to the disease and may be candidate targets for treatment to restore the system to its original healthy state [5, 6]. From a medical approach, systems biology offers a primal input to conduct functional analyses of genomic functions that could be commonly used in finding of the gene, detection of biomarker, disease categorization, drug discovery, therapy strategies and, in the last instance, predictive and preventive medicine [6, 7].
Autism is the highest expanding neurodevelopmental disorder in the world today. In the US, the rates of autism have increased from 1 in 2500 in 1970 to 1 in 88 today [8, 9]. A triad of deficits in the areas of social communication, language advancement, repetitive behavior, and limited range of interests describe the core symptoms used in the diagnosis of autism [10]. The growth of autism seems to be a complex interaction between genes and environmental factors. Autism influences many systems in the body, which include metabolic, mitochondrial, immunological, gastrointestinal, and neurological [8].
Attention deficit hyperactivity disorder (ADHD) has been characterized by the core symptoms of inattention, hyperactivity, and impulsivity. ADHD presents in childhood but persists into adulthood in almost 60 percent of cases, with an estimated prevalence of 4.4 percent in United States adults [11].
Intellectual disability (ID) affects about 2–3% of the general population [12, 13]. Diagnostic criteria for identifying individuals with ID have undergone significant revisions, changes perhaps best chronicled through the versions of the Diagnostic and Statistical Manual of Mental Disorders (DSM) [14].
Therefore for identification of potential drug targets for Autism, Intellectual Disability (ID), and Attention Deficit Hyperactivity Disorder (ADHD), first one should understand the underlying mechanism of disease pathogenesis. With the inception of network biology, it has become easier to understand the whole disease mechanism at the system level by constructing a network-based computational model for diseases. Exploring these networks will provide valuable information about the functional association of genes/proteins in the network that in-turn helps in identifying the most potential drug target for disease among the vast array of genes/proteins in the network. The current in-silico study was attempted to study the underlying disease mechanism of Autism, Intellectual Disability (ID), and Attention Deficit Hyperactivity Disorder (ADHD) and to identify the most potential target for diseases. Further drug discovery approach was used to identify novel lead molecules for the disease.
2. MATERIALS AND METHODS
2.1. Data Collection
For Autism 494 genes, for Intellectual Disability (ID) 399 genes, and 137 genes for Attention Deficit Hyperactivity Disorder (ADHD) were reported from NCBI.
2.2. Construction of PPI Network
STRING [15] allows a detailed study of network interaction by providing several features. PPI network constructed using of STRING 10.0 (Search Tool for the Retrieval of Interacting Genes) [16] by 494 genes for Autism, 399 genes for Intellectual Disability (ID), and 137 genes for Attention Deficit Hyperactivity Disorder (ADHD) as input and based on highest confidence score 0.7 obtained from experimental data [17]. Further PPI network was analyzed and visualized by Cytoscape 3.3.0 [18, 19].
2.3. Analysis of PPI Network
Analysis of the protein-protein interaction (PPI) network was done by using Cytoscape 3.3.0. Betweenness Centrality (BC) and node degree are the parameters included in Cytoscape Plug-in Network Analyzer. Node is represented by gene and interaction is represented by edges. Edges' number specifies node degree [20]. Betweenness centrality explains the significance of the node primarily based on the quantity of the shortest ways that undergo every node. In the study, the PPI network was analyzed based on these parameters.
2.4. Protein Modeling and Structure Validation
Obtained drug target from PPI network analysis for neurodevelopmental disorders: Autism, Intellectual Disability (ID), and AHDH. For the identification of the target sequence, the BLAST program was performed for the search of a structurally similar sequence with the protein data bank (PDB). Based on the structure similarity parameter, SNAP25 and DRD4 were modeled using on-line modeling server SWISS-MODEL. The structure of target protein 4EJN (PDB-ID) for Autism was retrieved from PDB. Further, the modeled structures were validated through the Ramachandran Plot and Errat using the SAVES server.
2.5. Ligand Identification
The structures of twenty-seven possible phytochemical compounds having neuroprotective activity were selected for study based on various literature reviews (Table 1). The 2D & 3D structures of compounds were retrieved from the PubChem database. These compounds were downloaded in (sdf) file format & converted into (PDB) format using Chimera software. Prediction of Molecular & bioactivity analysis of leads was performed by using molinspiration chemoinformatics software that satisfied them as potential inhibitors.
S.No. | Phytochemical Name | Plant Name |
1 | Ginkgolide A | Ginkgobiloba L |
2 | Ginkgolide B | Ginkgobiloba L |
3 | Ginkgolide J | Ginkgobiloba L |
4 | Ginkgolide M | Ginkgobiloba L |
5 | Oroxylin A | Scutellariabaicalensis |
6 | Salidroside | Rhodiolarosea |
7 | Rosmarinic acid | Melissa officinalis |
8 | Urosolic acid | Melissa officinalis |
9 | Valepotriate | Valerian |
10 | Valerenic acid | Valerian |
11 | Acetoxyvalerenic Acid | Valerian |
12 | Coriaria lactone | Loranthusparasiticus |
13 | Huperzine A | Huperzia serrate |
14 | Ajmalicine | Catharanthusrosea |
15 | Lochnerine | Catharanthusrosea |
16 | Ajmaline | Rauwolfia serpentine |
17 | Serpentine | Rauwolfia serpentine |
18 | Beta amyrin | Canscoradiffusa |
19 | Hyoscyamine | Daturametel |
20 | Scopolamine | Daturametel |
21 | Sulphoraphane | Broccoli |
22 | Ginkgolide C | Ginkgo biloba L |
23 | Mangiferin | Mangiferaindica |
24 | Reserpine | Rauwolfia serpentine |
25 | Rosavin | Rhodiolarosea |
26 | Vinblastine | Madagascar periwinkle |
27 | Vincristine | Madagascar periwinkle |
2.6. Molecular Docking
Rigid molecular docking was performed between the target protein and ligand using Autodock 1.5.6 tool to predict the binding affinity, ligand efficiency, and inhibitory constant. The criteria of binding energy were taken into consideration (based on the lowest binding energy score) to select the best protein-ligand complex. Binding energy was calculated using a semi-empirical free energy force field with charge-based desolvation and grid-based docking.
3. RESULT AND DISCUSSION
3.1. Network Analysis and Identification of Target
The PPI networks were constructed inputting 494 genes for Autism, 399 genes for Intellectual Disability (ID), and 137 genes for Attention Deficit Hyperactivity Disorder (ADHD) using the STRING database. Obtained networks were analyzed and visualized using Cytoscape 3.3.0 plug-in Network Analyzer. Based on a topological parameter BC and node degree: AKT serine/threonine kinase 1 (AKT1) for Autism, Synaptosomal nerve-associated protein 25 (SNAP25) for Intellectual Disability (ID), and Dopamine receptor D4 (DRD4) for Attention Deficit Hyperactivity Disorder (ADHD) are selected as a key drug target with highest BC value and degree node Figs. (1, 2 & 3).
Various literature finding suggests the active role of AKT1 gene as a key modulator of the AKT-mTOR signaling pathway controlling the tempo of the process of newborn neurons integration during adult neurogenesis, including correct neuron positioning, dendritic development, and synapse formation. Ile67Asn variation in SNAP-25 isoform B inhibits exocytosis of synaptic vesicles by altering the α-helical coiled structure of the SNARE complex. This mutation is a cause disorder for Intellectual Disability(ID). SNAP25 is substantially involved in many major neurological disorders which makes it a primary target for diagnosis and treatment of threatening diseases [21]. Dopamine D4 receptor gene (DRD4) has an important role in the pathogenesis of ADHD and one of the most important genes and is held to be responsible for neuronal signaling in the mesolimbic system of the brain area [22].
3.2. Protein Modeling and Structure Validation
The total length of AKT1 protein is 480 amino acids, SNAP25 protein is 206 amino acids, and DRD4 protein is 467 amino acids. The 3D structure of target protein 4EJN for Autism was retrieved from PDB and the 3-D structures of SNAP25 and DRD4 were built using SWISS-MODEL. The model structure is further validated through online server Rampage. The position of amino acid residues in allowed and disallowed region and overall Stereochemical property of the protein was accessed by Ramachandran plot. The Ramachandran plot indicates 91% for Autism, 89.8% for Intellectual Disability (ID), and 93.9% for Attention Deficit Hyperactivity Disorder (ADHD) in the favored region that reflects the good quality model as the expected value is above 85% in the favored region (Figs. 4, 5, & 6).
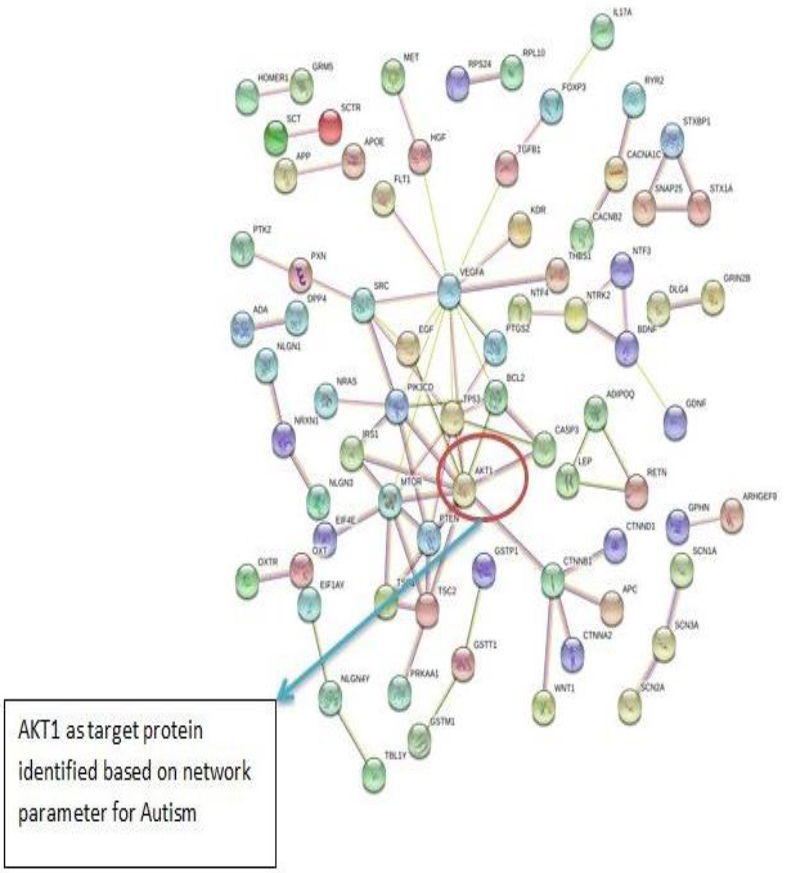
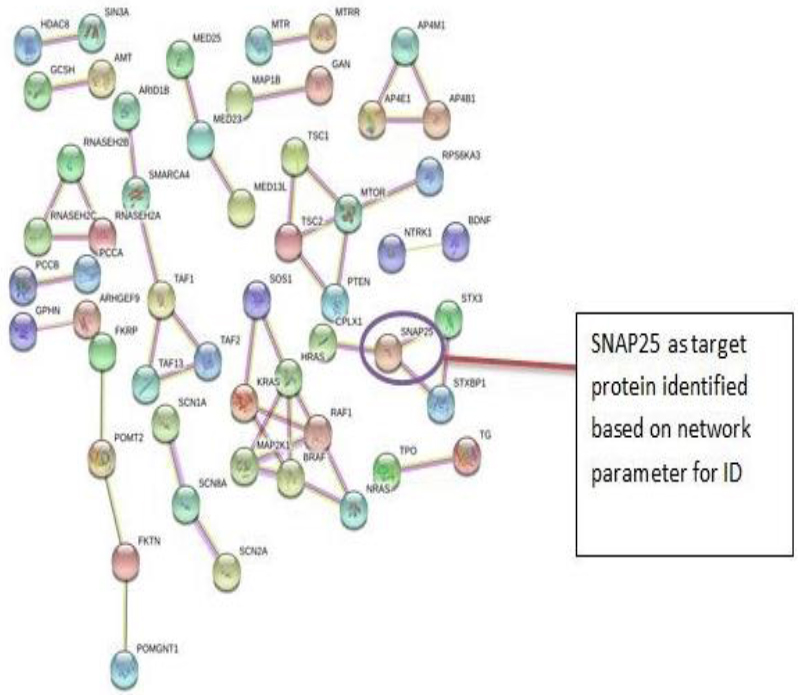
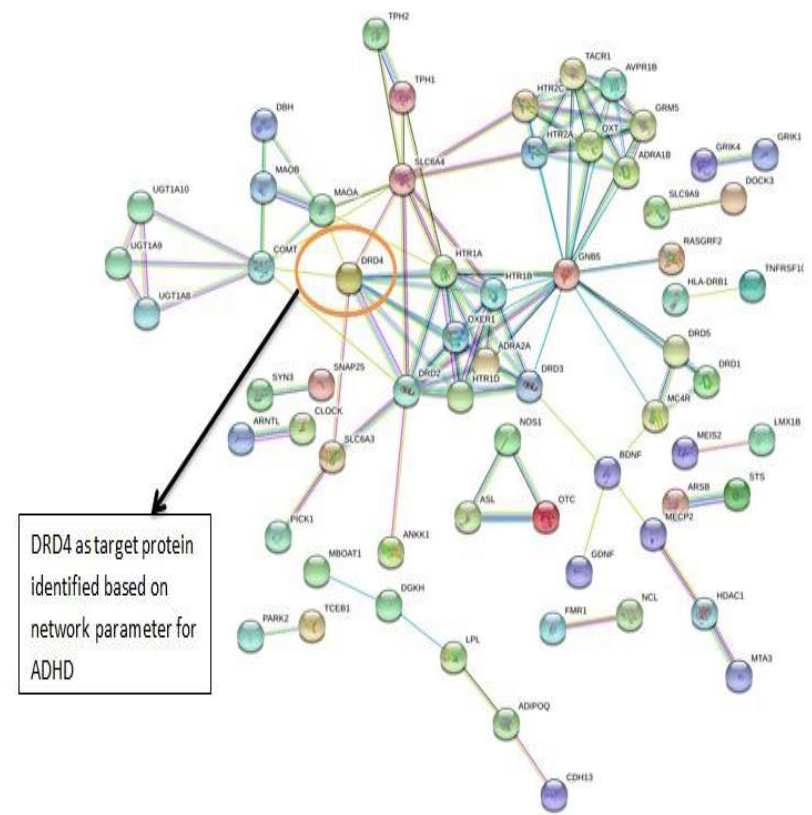
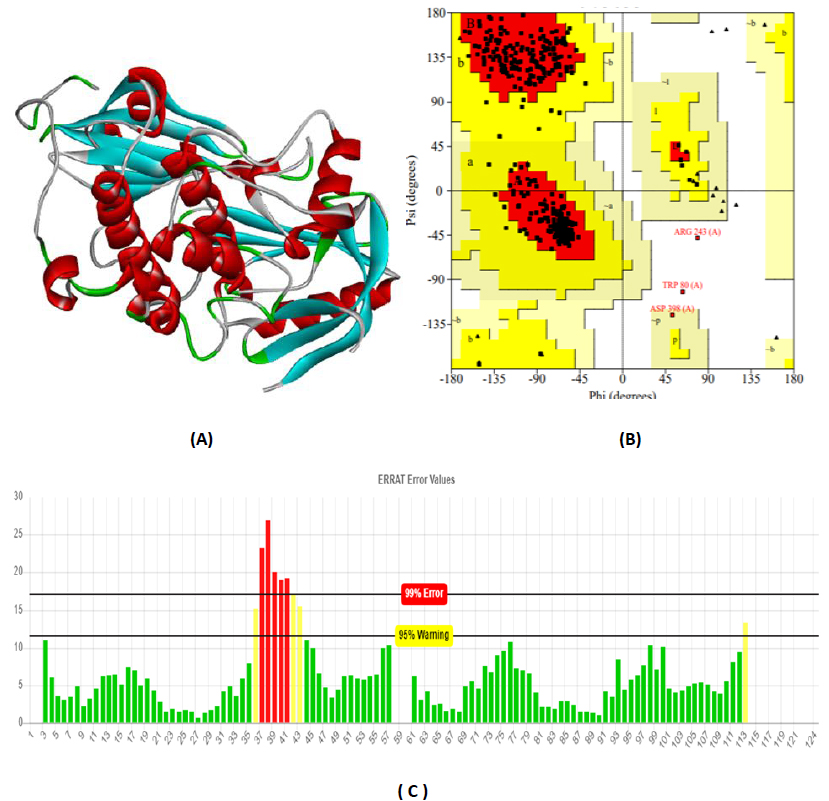
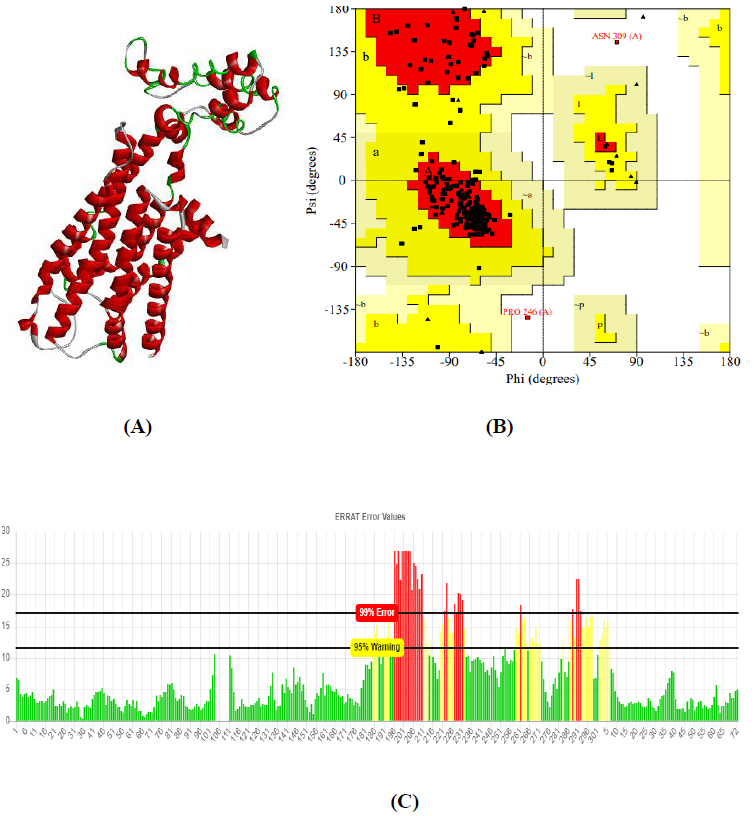
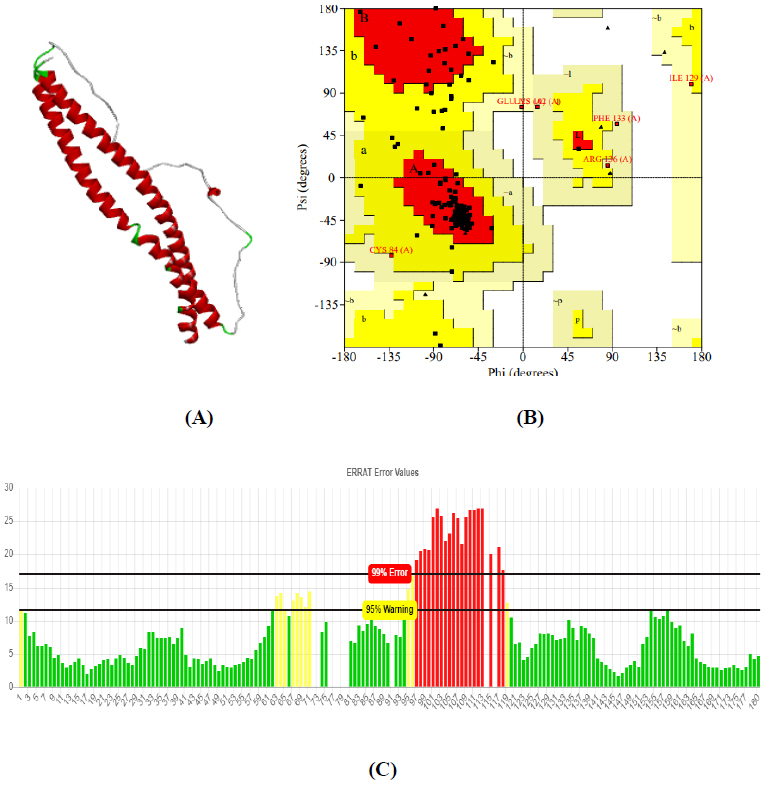
3.3. Molecular Docking Analysis
The molecular docking analysis is the probably most suitable method to identify best-fit interaction between protein and ligand. Protein-ligand interaction plays a key role in drug discovery. Docking uses the computational simulation to predict the preferred orientation of ligand to a receptor when interacted with each other to form a higher stability complex.
Molecular docking was performed for best 5 phytochemicals having neuroprotective activity reported in various literature searches, against AKT1, SNAP25, and DRD4 proteins using Autodock 1.5.6 tool. The protein PDB file was prepared by adding essential hydrogen atoms, Kollman charges, and solvation parameters and converting it into PDBQT format by using the Auto Dock Tool (ADT). The ligand file was prepared by detecting root and torsion and converting it into PDBQT format. The grid map was set of 60 x 60 x 60-angstrom grid points that were generated using the Auto grid program. The docking was performed using a Lamarckian Genetic Algorithm (LGA) parameters and run on the Cygwin platform. Docking results were analysed based on the lowest binding free energy (BE) and optimal docking energy conformation (Tables 2, 3 and 4 & Figs. 7, 8 and 9).
S.No. | Ligand (Phytochemical) | Affinity (kcal/mol) | Plant source | PubChem ID |
---|---|---|---|---|
1. | Hyoscyamine | -2.23 | Daturametel | 154417 |
2. | Sulphoraphane | -3.49 | Broccoli | 5350 |
3. | Oroxylin A (5,7-dihydroxy-6-methoxyflavone) | -4.18 | Scutellariabaicalensis | 5320315 |
4. | Valerenic acid | -4.50 | Valerian | 6440940 |
5. | Huperzine A | -6.70 | Lycopodiumserratum | 5912039 |
S.No. | Ligand (Phytochemical) | Affinity (kcal/mol) | Plant source | PubChem ID |
---|---|---|---|---|
1. | Sulphoraphane | -2.27 | Broccoli | 5350 |
2. | Valerenic acid | -2.46 | Valerian | 6440940 |
3. | Oroxylin A (5,7-dihydroxy-6-methoxyflavone) | -2.98 | Scutellariabaicalensis | 5320315 |
4. | Hyoscyamine | -3.51 | Daturametel | 154417 |
5. | Huperzine A | -4.32 | Lycopodiumserratum | 5912039 |
S No. | Ligand (Phytochemical) | Affinity | Plant source | PubChem ID |
---|---|---|---|---|
1. | Huperzine A | -2.27 | Lycopodiumserratum | 5912039 |
2. | Sulphoraphane | -2.30 | Broccoli | 5350 |
3. | Hyoscyamine | -2.64 | Daturametel | 154417 |
4. | Oroxylin A (5,7-dihydroxy-6-methoxyflavone) | -2.78 | Scutellariabaicalensis | 5320315 |
5. | Valerenic acid | -3.00 | Valerian | 6440940 |
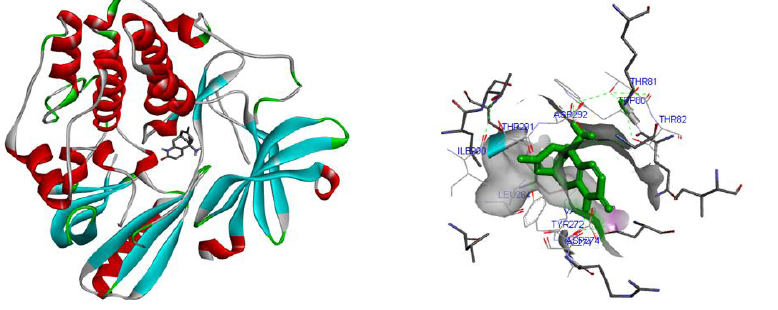
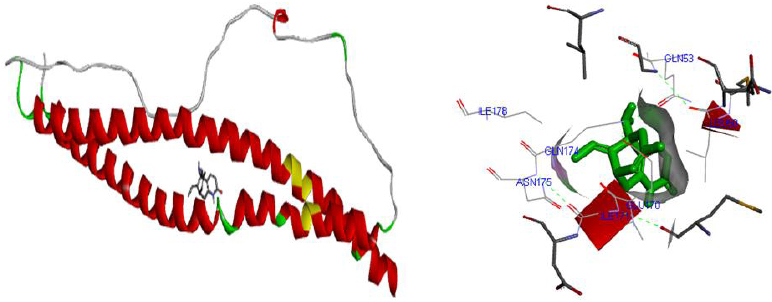
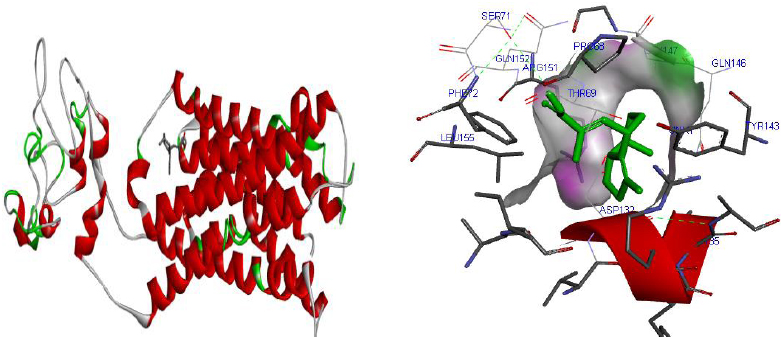
CONCLUSION
The candidate genes responsible for the progression of Autism is AKT1 gene, SNAP25 gene for Intellectual Disability (ID), and DRD4 gene for Attention Deficit Hyperactivity Disorder (ADHD) and were identified as a potential target. Information on these genes was retrieved from NCBI (National Center for Biotechnology Information. STRING and Cytoscape database aims to simplify access to this information by providing a comprehensive, yet a quality-controlled collection of protein-protein associations for a large number of organisms. The 3D structures of protein AKT1 were retrieved from PDB and SNAP25 and DRD4 were modeled and validated. Various phytochemicals were curated from the literature study that showed a therapeutic effect against various diseases. Natural products especially derived from plants have long been used in the treatment of human ailments. “Biological friendliness”, “drug-likeness”, low toxicity, and easy availability take natural products far ahead to be developed as drugs than synthetic compounds.
Docking is a valuable and skilled tool for in silico screening. The docking studies were performed between the target proteins 4EJN for Autism, SNAP25 for Intellectual Disability (ID), and DRD4 for Attention Deficit Hyperactivity Disorder (ADHD) with known phytochemicals. Based on the lowest binding affinity: Huperzine A (extract of Lycopodiumserratum) against Autism and Intellectual Disability and Valerenic acid (extract of Valerian) against Attention Deficit Hyperactivity Disorder (ADHD) could be possible potential therapeutic phytochemical for treatment. Further, the obtained molecule if experimentally validated would play a promising role in the treatment of NDDs in humans.
ETHICS APPROVAL AND CONSENT TO PARTICIPATE
Not applicable.
HUMAN AND ANIMAL RIGHTS
No animals/humans were used for studies that are the basis of this research.
CONSENT FOR PUBLICATION
Not applicable.
AVAILABILITY OF DATA AND MATERIALS
The data supporting the findings of the article is available in the [National Center for Biotechnology Information ] at [https://www.ncbi.nlm.nih.gov/gene/].
FUNDING
None.
CONFLICT OF INTEREST
The authors declare no conflict of interest, financial or otherwise.
ACKNOWLEDGEMENTS
We gratefully acknowledge the support provided by Bioinformatics tools, software, & databases in the work.