All published articles of this journal are available on ScienceDirect.
Computational Pharmacogenetics of P-Glycoprotein Mediated Antiepileptic Drug Resistance
Abstract
Background:
The treatment of epilepsy using antiepileptogenic drugs is complicated by drug resistance, resulting in treatment failure in more than one-third of cases. Human P-glycoprotein (hPGP; MDR1) is a known epileptogenic mediator.
Methods:
Given that experimental investigations have suggested a role for pharmacogenetics in this treatment failure, it would be of interest to study hPGP polymorphisms that might contribute to the emergence of drug resistance. Changes in protein functional activity could result from mutations as well as altered abundance. Bioinformatics approaches were used to assess and rank the functional impact of 20 missense MDR1 polymorphisms and the top five were selected. The structures of the wildtype and variant hPGP were modelled based on the mouse PGP structure. Docking studies of the wildtype and variant hPGP with four standard anti-epileptic drugs were carried out.
Results:
Our results revealed that the drug binding site with respect to the wildtype protein was uniform. However, the variant hPGP proteins displayed a repertoire of binding sites with stronger binding affinities towards the drug.
Conclusion:
Our studies indicated that specific polymorphisms in MDR1 could drive conformational changes of PGP structure, facilitating altered contacts with drug-substrates and thus modifying their bioavailability. This suggests that MDR1 polymorphisms could actively contribute to the emergence of pharmaco-resistance in antiepileptic therapy.
1. INTRODUCTION
PGP (HGNC nomenclature: ABCB1) is a key transmembrane protein conserved from bacteria to man, and its function is to protect the organism from toxic xenobiotics. PGP has turned out to be a critical player in multiple drug resistance phenomena. Here, we are interested in its role in antiepileptic drug resistance. Epilepsy is a chronic neurological condition affecting more than 50 million people worldwide and 1-2% of the population [1]. The recurring limitation in the treatment protocol of epilepsy is the failure of drug-response in more than one-third of cases. This is the case with the > 30 FDA-approved drugs for epilepsy. PGP is an ATP-coupled efflux pump documented as an epileptogenic mediator [2]. It is known to be highly expressed in the blood-brain barrier, which is pharmacologically crucial for the bioavailability of drugs acting on the central nervous system [3]. The experimental evidence for the role of PGP polymorphisms in antiepileptic drug resistance has not been conclusive so far [4-10]. There is evidence for its cognate role in antidepressant therapy [11]. There are at least two mechanisms by which PGP could mediate refractory epilepsy. First, elevated levels of PGP protein might be linked with the low intracellular drug concentration in cortical cells observed in epilepsy treatment. Elevated abundance could result from multiple mechanisms, for example, promoter sequence mutations, decrease in methylation-mediated epigenetic silencing, change in miRNA-mediated transcript abundance, and decrease the rate of protein degradation. PGP is well-known for its broad substrate specificity, and greater PGP abundance would result in phenotypic drug resistance due to effective drug extrusion. Alternatively, a gain-of-function mutation or changes in protein folding might enhance its functional activity, resulting in the same phenotype, i.e., hyperactive PGP leading to pharmacoresistant epilepsy (Fig. 1)[12, 13].
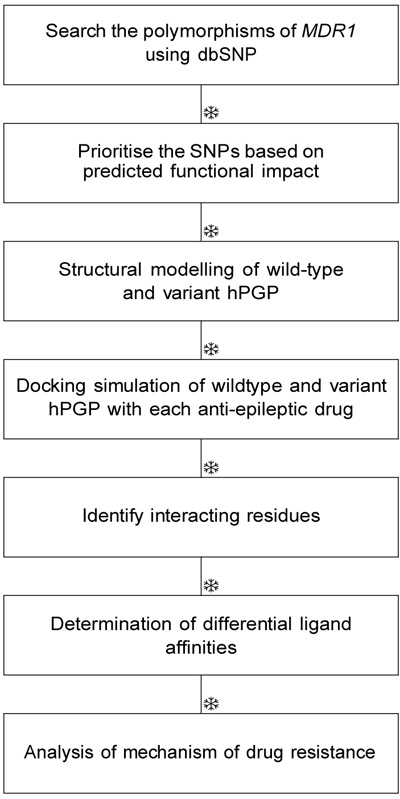
PGP consists of two homologous halves, each consisting of a transmembrane (TM) domain with six alpha helices and a Nucleotide-Binding Domain (NBD) [14]. A large, hydrophobic and polyspecific drug-binding pocket resides in the inverted V-shaped internal cavity formed by the transmembrane domains [15]. It is clear that he key to epilepsy treatment would involve control of the epileptogenic mediator proteins, including hPGP. Structural studies of hPGP might enhance our current understanding of the role of PGP in drug resistance mechanisms, and provide any evidence of the relationship between specific MDR1 haplotypes and altered drug pharmacokinetics. Given that little information is available on the pharmacology of missense polymorphisms of MDR1, analysis of the role of gain-of-function muta tions in PGP would be valuable. Earlier bioinformatic studies of susceptible genetic Loci have identified and analyzed key polymorphisms specific to pathological phenotypes, for e.g., polymorphisms associated with methylenetetrahydrofolate reductase (MTHFR) gene [16-21]. Genetic polymorphisms are known to influence sus ceptibility to epilepsy (for e.g., BDNF [22, 23]). Here, we have attempted an in silico study of PGP polymorphisms and their structure-activity relationships with respect to drug-substrates to obtain a comprehensive picture of PGP role in the emergence of antiepileptic drug insensitivity. PGP-mediated processes are also the major con tributors to the emergence of drug resistance in cancer therapy and other conditions. Our results could extend to examining the role of P-glycoprotein in generalized drug-resistance in multiple conditions [24].
2. MATERIALS AND METHODS
2.1. Polymorphism Analysis
The hPGP sequence was retrieved from UniProt (acc. no. P08183). A PSI-BLAST search was performed using hPGP as query and target database as Vertebrates, with an E-value of 0.001 until convergence [25]. We selected top 5000 sequences from this result and clustered for redundancy at 40% sequence identity [26]. Multiple align ment of all the hits was performed using ClustalX [27] and manually edited (available in Supporting Information). The dbSNP was to used to identify hPGP SNPs withs the search term: “human [orgn] AND missense AND PGP”. The hits were assessed for the functional impact of polymorphisms using the curated multiple alignments obtained above. Three different tools were used: SIFT [28], PolyPhen2 [29], and PhD SNP [30]. Consensus of these predictions was used to evaluate the functionally important SNPs.
2.2. Homology Modeling
The template structures were retrieved using a Blast search of hPGP against the PDB database [31]. ClustalX was used to align the template and hPGP (i.e., target). Modellerv9 was used for modelling and energy-minimisation [32]. For each target, five separate models were generated and the model with the least DOPE (discrete optimized potential energy) score was chosen as the best model. The structure of a variant protein could be obtained by modeling in the mutation on the wild-type structure, however, this would not model any global effects due to the mutation. In order to fully account for the effects of the mutation, we modelled the variant proteins independently of the wildtype protein. Molprobity was used to validate the models obtained [33].
2.3. Protein and Ligand Preparation
Autodock 4.2 suite of tools was used for carrying out the docking simulations of hPGP variants and anti-epileptic drugs [34, 35]. Hydrogen atoms missing in the protein were added. This was followed by the addition of partial charges to the atoms. The protein was then converted to PDBQT format. The SMILES notation of the drugs of interest was retrieved from Pubchem [36]. The PDB co-ordinates of the drugs of interest were generated from their SMILES representation using OpenBabel [37]. To generate the conformers of each drug, we used MGLtools by calculating the number of bond torsions in the 3D structure. The ligand was then converted to the PDBQT format as well using AutoDock Tools. Target affinity maps for each atom type in the ligand were generated by autogrid by defining a uniform grid box centered in the hPGP internal cavity. This procedure was repeated for each target-ligand pair, for a total of 6 × 4 = 24 times.
2.4. Docking
We employed the Lamarckian genetic algorithm with default parameters for docking search, with 2,500,000 cycles per run, and1 10 runs per receptor-ligand pair. The binding mode with the least binding energy was defined as the best pose. The ten poses obtained for each receptor-ligand pair were clustered at 2.0Å r.m.s. to validate the convergence to the best pose. The docked complex was then loaded in PDBQT format, converted to PDB coordinates using OpenBabel, and finally visualized using Rasmol2.7 [38]. The differential affinity of the variant for a given ligand relative to the wildtype was estimated as the difference in the binding energies, i.e. ∆∆Gmut = ∆Gbind,mut − ∆Gbind,wt.
3. RESULTS
Nearly 500 hPGP SNPs were retrieved, however most of these were unannotated, and we obtained a set of 20 hPGP SNPs for further study, none of whose functional effects were known in the literature (Table 1). The results of our assessment of functional impact by various approaches are summarised in Table 2. Most of the SNPs were determined to be neutral, not disease-causing or deleterious. Five SNPs were predicted to be functionally important by at least one of the tools, as shown in Table 2.
No. | rsid | SNP | Location |
---|---|---|---|
1 | rs28381804 | F17L | N-terminal domain |
2 | rs9282564 | N21D | N-terminal domain |
3 | rs1202183 | N44S | TM1 |
4 | rs9282565 | A80E | Linker between TM1 and TM2 |
5 | rs1128501 | G185V | TM3 |
6 | rs36008564 | I261V | Linker between TM4 and TM5 |
7 | rs2229109 | S400N | NBD1 |
8 | rs28381902 | E566K | NBD1 |
9 | rs28381914 | R593C | NBD1 |
10 | rs2235036 | A599T | NBD1 |
11 | rs35023033 | R669C | NBD1 |
12 | rs2235039 | V801M | Linker between TM8 and TM9 |
13 | rs2032581 | I829V | TM9 |
14 | rs28381967 | I836V | TM9 |
15 | rs2032582 | S893A | Linker between TM11 and TM12 |
16 | rs72552784 | A999T | NBD2 |
17 | rs28401798 | P1051A | NBD2 |
18 | rs55852620 | Q1107P | NBD2 |
19 | rs2229107 | S1141T | NBD2 |
20 | rs28364274 | V1251I | NBD2 |
No. | SNP | PolyPhen2 Prediction | PolyPhen2 Probability | SIFT Prediction |
SIFT Score |
phdsnp Prediction |
phdsnp Reliability |
---|---|---|---|---|---|---|---|
1 | G185V | Prob. Damaging | 1 | Damaging | 1 | Disease | 8 |
2 | R593C | benign | 0.392 | Damaging | 1 | Disease | 6 |
3 | E566K | Prob. Damaging | 1 | Damaging | 0.88 | Disease | 6 |
4 | Q1107P | Prob. Damaging | 0.962 | tolerated | 0.19 | Disease | 6 |
5 | A999T | Poss. Damaging | 0.465 | tolerated | 1 | Neutral | 7 |
Table 3 provides the representative structures of P-glycoprotein in the PDB. Of these homologous hits, the mouse structures cover the full length of the hPGP. Some mouse structures co-crystallised with a ligand might not be representative of the native PGP conformation. When 4Q9H was superimposed with the 3G5U structure, it was observed that the register of the C-terminal half of the ’inverted-V’ of 4Q9H was displaced relative to that of 3G5U (Fig. 2), which rendered 4Q9H unsuitable for modeling the full hPGP structure. The alignment between the hPGP and 3G5U is very good, showing > 87.5 % sequence identity and good sequence coverage (Fig. 3). 3G5U was used as the template for homology modeling. The target structures of the hPGP wildtype and the five variants were independently modeled and energy- minimised five times each, and the best model was used for further studies. All the modelled structures are available in the Supporting Information and their mutual rms deviations are shown in Table 4.
pdbid | Organism | Chains | Length | Res. ˚A | #TM helices |
---|---|---|---|---|---|
3G5U | Mus musculus | A,B | 1284 | 3.80 | 12 |
4Q9H | Mus musculus | A | 1284 | 3.40 | 12 |
4KSB | Mus musculus | A | 1284 | 3.80 | 12 |
3G61 | Mus musculus | A,B | 1284 | 4.35 | 12 |
3G60 | Mus musculus | A,B | 1284 | 4.40 | 12 |
4KSC | Mus musculus | A | 1284 | 4.00 | 12 |
4LSG | Mus musculus | A,B | 1284 | 3.80 | 12 |
4M1M | Mus musculus | A,B | 1282 | 3.80 | 12 |
2HYD | Staphylococcus aureus | A,B | 578 | 3.00 | 6 |
3B5Z | Salmonella enterica | A,B,C,D | 582 | 4.20 | 5 |
3WME | Cyanidioschyzon merolae | A | 612 | 2.75 | 3 |
– | 3g5u | wt | Mut1 | Mut2 | Mut3 | Mut4 |
---|---|---|---|---|---|---|
wt | 4.51 | – | – | – | – | – |
Mut1 | 4.24 | 0.53 | – | – | – | – |
Mut2 | 4.43 | 0.62 | 1.31 | – | – | – |
Mut3 | 4.32 | 0.71 | 1.19 | 1.33 | – | – |
Mut4 | 4.33 | 0.43 | 0.97 | 0.81 | 0.59 | – |
Mut5 | 4.63 | 0.49 | 0.71 | 1.04 | 0.48 | 0.44 |
Phenobarbital was first used as an antiseizure drug in 1912, followed by phenytoin. Today, more than 30 drugs are FDA-approved in the treatment of epilepsy, yet all of them face pharmacoresistance and more than one-third of epilepsy cases remain untreatable. In addition to phenobarbital and phenytoin, two other common antiepileptic medications, namely valproate and carbamazepine, were included in the set of ligands studied (Table 5).
No. | Drug | Pubchemid | Smiles Notation |
---|---|---|---|
1 | Valproate | 3121 | CCCC(CCC)C(=O)O |
2 | Phenytoin | 1775 | C1=CC=C(C=C1)C2(C(=O)NC(=O)N2)C3=CC=CC=C3 |
3 | Carbamazepine | 2554 | C1=CC=C2C(=C1)C=CC3=CC=CC=C3N2C(=O)N |
4 | Phenobarbital | 4763 | CCC1(C(=O)NC(=O)NC1=O)C2=CC=CC=C2 |
Docking between each hPGP protein (wildtype + 5 variants) and ligand was carried out. Ten docking runs were performed per receptor-ligand pair. Each run provides one low-energy docked conformation of the respective receptor-ligand pair. The site corresponding to the least-energy binding mode was taken as the binding site of the ligand with the receptor. To ascertain convergence to the lowest-energy binding mode, the ten runs of each receptor-ligand binding conformations were clustered at 2.0Å r.m.s. The lowest-energy binding modes showed good convergence. Of the 24 receptor-ligand pairs, 21 had energy-histograms showing the least binding energy (±0.2 kcal/mol) as the most probable conformation and the least binding energies of the rest were within 1 kcal/mol of the binding energies of the most prob- able conformation. This provided confidence that the docking procedure resulted in convergence to the optimum receptor-ligand conformation. The structures of the receptor-drug complexes, as well as the best poses (defined as within 4.5Å of the ligand), are available in the Supporting Information. A comparison of the best poses between the wildtype and one of the variants is illustrated in Fig. (4). The groups of contacting residues specific to each docked complex are shown in Table 6. These residues were defined at a contact distance of < 4.5Å from the drug in the bound conformation. The summary of the docking analysis is presented in Table 7.
Receptor | Ligand | Interacting Residues |
---|---|---|
Wildtype | Drug1 | Leu244,Tyr247,Arg286,Ile289,Lys290,Ile293,Ala823,Lys826 |
Wildtype | Drug2 | Gly141,Ile144,His145,Arg148,Val179,Ser180,Asn183,Asp886,Leu890,Leu924,Pro927,Tyr928,Ser931,Lys934 |
Wildtype | Drug3 | Gly141,Ile144,His145,Arg148,Val179,Ser180,Asn183,Asp886,Leu890,Leu924,Pro927,Tyr928,Ser931,Lys934 |
Wildtype | Drug4 | Met701,Asn704,Leu705,Trp708,Ile829,Arg832,Leu833 |
G185V | Drug1 | Thr422,Leu554,Gln556,Ala557,Thr558,Gln570,Leu573,Asp574,Arg577,Val584,Thr591,Val592,Asn594,Ala595 |
G185V | Drug2 | Ile199,Phe303,Tyr307,Asn721,Leu724,Gln725,Ser766,Gln838,Met986,Ala987,Gln990,Val991,Phe994 |
G185V | Drug3 | Ile199,Phe303,Tyr307,Asn721,Leu724,Gln725,Ser766,Gln838,Ala987,Gln990,Val991,Phe994 |
G185V | Drug4 | Lys291,Ala292,Asn296,Gln773,Phe777,Gly778,Glu782,Lys826,Ser831,Phe994,Ala995,Pro996 |
R593C | Drug1 | Leu244,Tyr247,Lys285,Arg286,Ile289,Lys290,Ile293,Thr785,Lys826 |
R593C | Drug2 | Thr627,Met628,Gln629,Thr630,Leu688,Phe804,Pro807,Lys808,Asn809,Thr810,Thr811 |
R593C | Drug3 | Gln132,Val133,Trp136,Ile190,Glu875,Leu879,Phe938,Thr941,Phe942 |
R593C | Drug4 | Gln132,Val133,Trp136,Ile190,Glu875,Leu879,Phe938,Gly939,Thr941,Phe942 |
E566K | Drug1 | Leu244,Tyr247,Lys285,Arg286,Ile289,Lys290,Ile293,Thr785,Lys826 |
E566K | Drug2 | Gln132,Val133,Trp136,Cys137,Gly187,Ile190,Glu875,Leu879,Phe938,Thr941,Phe942 |
E566K | Drug3 | Ile293,Phe777,Gly778,Gly781,Ala823,Gln824,Lys826,Gly827,Ile829,Gly830,Phe994,Ala995,Pro996 |
E566K | Drug4 | Ser831,Ala834,Val835,Gln838,Gln990,Val991,Ser992,Ser993,Phe994,Ala995,Pro996,Asp997,Tyr998 |
Q1107P | Drug1 | Tyr247,Glu282,Arg286,Lys290,Gly781,Glu782,Thr785,Arg789,Ala823,Gln824,Lys826 |
Q1107P | Drug2 | Asp689,Glu690,Ser691,Ile692,Lys808,Asn809,Ala813,Leu814,Thr816,Arg817,His1007,Met1010,Ile1011 |
Q1107P | Drug3 | Val133,Trp136,Cys137,Glu875,Leu879,Lys934,Phe938,Thr941,Phe942 |
Q1107P | Drug4 | Ser831,Ala834,Val835,Gln838,Gln990,Val991,Ser992,Ser993,Phe994,Ala995,Pro996,Asp997,Tyr998 |
A999T | Drug1 | Leu244,Tyr247,Glu282,Arg286,Ile289,Lys290,Ile293,Thr785,Arg789,Ala823,Lys826 |
A999T | Drug2 | Ala259,Ala260,Ile261,Arg262,Thr630,Leu688,Phe804,Pro807,Lys808,Asn809,Thr810,Thr811,Leu814 |
A999T | Drug3 | Ser831,Ala834,Val835,Gln838,Gln990,Val991,Ser993,Phe994,Ala995,Pro996,Asp997,Tyr998 |
A999T | Drug4 | Ser831,Ala834,Val835,Gln838,Gln990,Val991,Ser992,Ser993,Phe994,Ala995,Pro996,Asp997,Tyr998 |
Receptor | Valproate | Phenytoin | Carbamazepine | Phenobarbital |
---|---|---|---|---|
wildtype | -4.28 | -5.48 | -6.35 | -5.24 |
G185V | -3.90 | -6.38 | -6.27 | -5.62 |
R593C | -5.21 | -5.52 | -6.37 | -5.74 |
E566K | -5.53 | -6.17 | -5.55 | -6.15 |
Q1107P | -5.50 | -5.47 | -6.66 | -5.52 |
A999T | -5.15 | -5.65 | -6.67 | -6.48 |
∆∆GG185V | 0.38 | -0.90 | 0.08 | -0.38 |
∆∆GR593C | -0.93 | -0.04 | -0.02 | -0.5 |
∆∆GE566K | -1.25 | -0.69 | 0.80 | -0.91 |
∆∆GQ1107P | -1.22 | 0.01 | -0.31 | -0.28 |
∆∆GA999T | -0.87 | -0.17 | -0.32 | -1.24 |
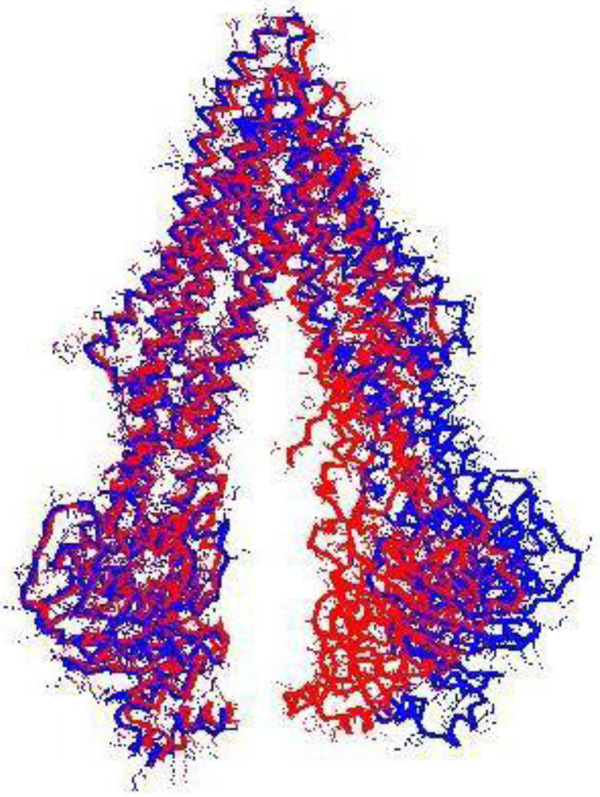
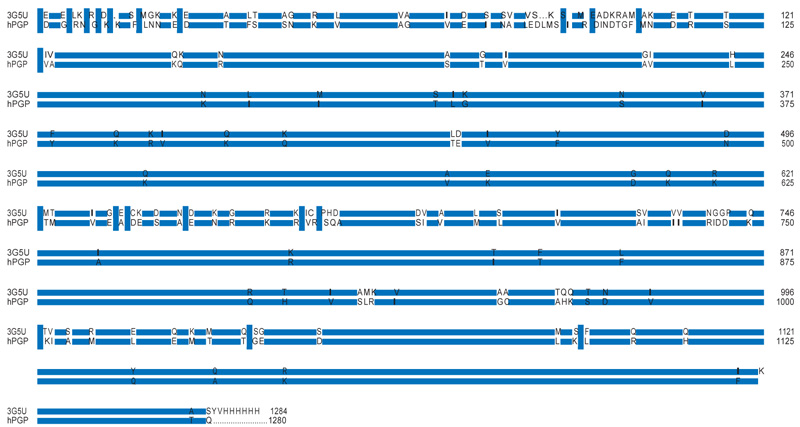
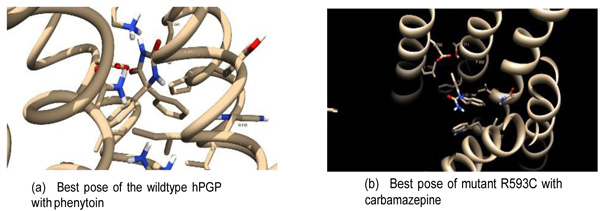
4. DISCUSSION
The evolutionary conservation of the location of the prioritised SNPs was computed using the multiple alignments constructed from PGP homologous sequences non-redundant at 40% sequence identity. The quantile scores of each position with respect to hPGP sequence are given in the Supporting information. All five polymorphism locations ranked at least in the 50th quantile, and the E566K in particular ranked 97th. The polymorphisms were found in evolutionarily conserved positions. Experimental evidence is available on some of the polymorphisms [39-41].
The hPGP residues binding the ligand in each hPGP-drug pair represent the drug-specific binding pocket (Table 6). These residues contributed to stabilizing the docked complex by forming hydrogen bonds and Van der Waals interactions with the substrates. It is observed that the binding site of wildtype hPGP is identical for phenytoin and carbamazepine. This is a 14-residue binding pocket in the internal cavity lined by four hydrophobic residues (Ile144, Val179, Leu890, Leu924), three charged residues (Arg148, Asp886, Lys934) and four polar residues (Ser180, Asn183, Tyr928, Ser931). In contrast, the variant proteins bound the drugs in alternative variable regions, notably a binding pocket involving Gln132, Val133, Trp136, Ile190, Glu875, Leu879, Phe938, Thr941, and Phe942 that bound all drugs except valproate. It was remarkable that for a given variant hPGP, the binding pocket interactions differed for each drug. The R593C hPGP variant bound phenytoin very close to the mutation site, suggesting evidence for a local conformational change in binding the drug.
Further clarity on these observations could be obtained on an examination of the estimated binding free energies of the wildtype and variant hPGPs with the different drugs of interest and the corresponding predicted differential ligand affinity given by ∆∆Gmut = ∆Gbind,mut − ∆Gbind,wt (Table 7). It was observed that all but three of the differential ligand affinities were negative. This implied that the variant hPGP bound each drug with a stronger affinity than the wildtype hPGP. The maximum range of differential response was observed with variant E566K (−1.25 kcal/mol < ∆∆Gmut < 0.80 kcal/mol).
Two features indicated the neutrality of wildtype hPGP with respect to binding anti-epileptic drugs. First, the binding pocket appeared constant for both phenytoin and carbamazepine. Second, the binding energy with the drug was higher relative to the variants and hence less tight. On the other hand, there were two features that indicated that variant hPGPs would assist in the development of drug resistance.
First, variant hPGPs bound each drug in a different location in the internal cavity. Variability in location affords a better search of the optimal binding modes of the drug. Second, consistently lower binding energies were observed, implying stable drug-PGP complexes for possible energetic extrusion of the drug. The in silico analysis showed that polymorphisms could have played a role in relocating the optimal drug-binding cavity for a higher affinity, relative to the wild-type hPGP.
An elevated affinity between a variant hPGP and the drug could suggest a potential differential adverse response to therapy. From Table 7, it is observed that this is the case for 17 out of the 20 drug-protein combinations studied. Experimental studies are necessary to validate these results and determine whether the mag nitude of any differential adverse responses could translate to the threshold for the development of pharmacoresistance. By contributing to drug resistance, PGP polymorphisms could conceivably play an adverse role in the treatment of multiple epilepsy subtypes. One example is electrical status epilepticus during slow sleep (’ESES’), a condition which could primarily affect children [42] and should best be treated early. The response to use of valproate and diazepam treatment regimens for ESES is quite heterogeneous [43-45], indicating inter-individual variability and a possible role for polymorphisms of pharmacogenomic importance, including those of PGP analyzed in our study.
CONCLUSION
Though hPGP is well-documented as a modifier of drug bioavailability in many conditions, its role in antiepileptic drug resistance has been controversial. At least two alternative mechanisms could explain the hPGP-mediated epileptogenic phenotype. Our work suggests that polymorphisms are a viable mechanism of PGP action that could lead to drug resistance acquisition independent of other mechanisms. It is interesting that all the polymorphisms appeared to result in gain-of-function. Coupled with the observation that somatic mutations could have a similar effect to identical inherited polymorphisms, this would suggest that PGP is a potential oncogene in the context of cancer drug resistance.
Developing a drug resistance strategy to combat drug resistance is a top priority. Our work has highlighted that MDR1 polymorphisms could potentially lower the threshold for development of pharmacoresistance. This gain-of-function process in hPGP offers a novel candidate target in the fight against antiepileptic drug re sistance. Experimental validation of our work is necessary to apply our findings towards achieving pharmacosensitive response in epilepsy treatment. Our methodology is extendable to studies investigating the effect of genetic polymorphisms on phenotypes in other diseases and conditions.
AUTHORS’ CONTRIBUTION
Dr. Ashok Palaniappan conceived and designed the experiments. Experiment was performed and data was analyzed by Ms. Sindhu Varghese and Dr. Ashok Palaniappan. Dr. Ashok Palaniappan prepared the figures and/or tables.
Dr. Ashok Palaniappan drafted the work and revised it critically for important content.
ETHICS APPROVAL AND CONSENT TO PARTICIPATE
Not applicable.
HUMAN AND ANIMAL RIGHTS
No animals/humans were used for studies that are the basis of this research.
CONSENT FOR PUBLICATION
Not applicable.
CONFLICT OF INTEREST
The authors declare no conflict of interest, financial or otherwise.
ACKNOWLEDGMENTS
Declared none.
SUPPLEMENTARY MATERIAL
Supplementary material is available on the publishers Website along with the published article.