Immunoinformatics Approach for the Design of Chimeric Vaccine Against Whitmore Disease
Abstract
Purpose:
Multidrug-resistant Burkholderia pseudomallei is associated with significant morbidity and mortality. Hence, there is a requirement for a vaccine for this pathogen. Using subtractive proteomics and reverse vaccinology approaches, we have designed a chimeric multiepitope vaccine against the pathogen in the present study.
Methods:
Twenty-one non-redundant pathogen proteomes were mined using a subtractive proteomics strategy. Out of these, by various analyses, we found proteins that were non-homologous to humans, essential, and virulent. BLASTp against the PDB database and Pocket druggability analysis yielded nine proteins whose 3D structure is available and are druggable. Four proteins that could be candidates for vaccines were identified by subcellular localization and antigenicity prediction, and they could be used in reverse vaccinology methods to create a chimeric multiepitope vaccine.
Results:
Using online resources and servers, MHC class I, II, and B cell epitopes were identified. The predicted epitopes were selected based on analysis of toxicity, solubility, allergenicity, and hydrophilicity. These predicted epitopes, which were immunogenic, were used for the construction of a multivalent chimeric vaccine. The epitopes, adjuvants, linkers, and PADRE amino acid sequences were employed to create the vaccine. Shortlisted vaccine constructs also interact with the HLA allele and TLR4, as evident from docking and molecular dynamics simulation. Thus, vaccine construct V1 can elicit an immune response against Burkholderia pseudomallei.
Conclusion:
The availability of the proteome of B. pseudomallei has made this study possible through the usage of various in silico approaches. We could shortlist vaccine targets using subtractive proteomics and then construct chimeric vaccines using reverse vaccinology and immunoinformatics approaches.
1. INTRODUCTION
Melioidosis, or Whitmore disease, is caused by a facultative intracellular gram-negative bacterium, Burkholderia pseudomallei [1]. Burkholderia pseudomallei can survive as a saprophyte in soil and water, but it can cause severe infection in entering mammals. It is difficult to treat, resulting in high morbidity and mortality. The symptoms of the disease range from skin abscesses to acute pneumonia and septicemia. The bacterium can be transmitted by inhalation, percutaneous inoculation, and ingestion. However, other modes of transmission, viz. laboratory-acquired cases, person-to-person spread [2], sexual transmission [3], breast milk [4], and mother-to-child transmission [5] have also been reported. The incubation period is varied. It ranges from less than a day to 21 days and can extend up to several months or years [6, 7].
The treatment of the disease is biphasic: intravenous therapy of 10-14 days consisting of ceftazidime administered every 6-8 hours or meropenem administered every 8 hours. This is followed up by 3-6 months of oral antimicrobial therapy consisting of trimethoprim-sulfamethoxazole taken every 12 hours or amoxicillin/clavulanic acid (co-amoxiclav) taken every 8 hours. However, B. Pseudomallei is resistant to many antibiotics, so its treatment is complicated. The pathogen is resistant due to various intrinsic processes like target deletion, enzymatic inactivation, and efflux from the cell and is controlled by encoded genes [6].
No vaccine against the disease is available; hence, rapid detection and effective antibiotic treatment are required to manage the disease successfully. Relapse in antibiotic therapy or re-infection with a different strain can cause recurrent melioidosis [7]. B. Pseudomallei can also survive inside non-phagocytic and phagocytic cells, further complicating the treatment [8].
Melioidosis is most prevalent in Southeast Asia and Northern Australia [9-12]. Despite the rigorous antibiotic therapy, mortality rates are usually high, about 40%. Human cases of melioidosis are found to be the third most common cause of death from infectious diseases in Northeast Thailand (behind HIV and tuberculosis) [10]. In Northern Australia and regions of Southeast Asia, B. pseudomallei infections mostly cause community-acquired pneumonia, septic shock, and death, the most common outcome of acute infections [11]. According to a recent report, the global distribution of the pathogen is highly underreported. It has been found that B. pseudomallei is the leading cause of at least 165,000 human infections and approx 89,000 deaths worldwide [12]. The detection of disease in many areas of the world is a colossal challenge as clinical manifestations of B. pseudomallei infections are non-specific, and there is difficulty in the availability of diagnostics.
Furthermore, due to high morbidity and mortality, poor response to antibiotic treatment, and the ease with which it aerosolizes, B. pseudomallei has the potential to be used as a bioweapon. It has been classified as Category B Tier 1 Select Agent by the US Centers for Disease Control and Prevention (CDC) because of this reason. Although there is no evidence of B. pseudomallei being used as a weapon, B. mallei has a history of malicious use as a bioweapon [13].
B. pseudomallei is susceptible to very few antibiotics and also resistant to many, hence called multidrug-resistant [14]. Also, B. pseudomallei is listed as a Schedule five pathogen and toxin controlled under ATCSA in the United Kingdom; ATCSA is the Anti-Terrorism, Crime, and Security Act. Some vaccine candidates have shown partial protection against Whitmore disease in the murine infection model [15-17]. Few vaccine candidates have been tested on non-human primates or humans [18]. Live attenuated vaccine candidates induce a more comprehensive immune response in animal models and are considered the best way to protect against Burkholderia pseudomallei infection [19]. However, subunit vaccines are safer and have the potential for manufacturing at a large scale. Also, it has been experimentally proved that a combination of bacterial polysaccharides (LPSs or other capsular polysaccharides) with protein antigens (glycoconjugates) can elicit a better immune response against infection [19]. However, a multivalent vaccine candidate comprising numerous immunogenic epitopes will probably be required for complete protection, as they elicit an antibody response and cellular (CD4+ and CD8+ Tcell) immunity for protection against human Whitmore disease.
Vaccine target identification and prioritization against various diseases have been documented in multiple investigations such as those relating to Enterococcus faecium [20], Salmonella typhi H58 [21], Shigella sonnei [22], Burkholderia pseudomallei [23, 24], Porphyromonas gingivalis [25], Klebsiella pneumoniae [26], Ehrlichia chaffeenis [27], Lasa virus [28], Clostridium perfringens [29], Staphylococcus saprophyticus [30] and Trepanoma pallidum [31]
The availability of genomics data of different strains of B. psuedomallei makes the analysis all the easier to predict putative vaccine candidates. Subtractive proteomics and reverse vaccinology approaches have become more promising recently for designing an effective, affordable vaccine against various pathogens [32-34]. Subtractive proteomics and later reverse vaccinology approaches were used in this study to shortlist the antigenic protein, predict epitopes, and construct chimeric vaccines. A variety of bioinformatics approaches, such as protein-protein docking, MD simulation, and in silico cloning, verified the chimeric vaccine's stability and efficacy. It was found that the designed multiepitope chimeric vaccine in the current study can make stable interactions with human immune receptors and elicit a strong immune response.
2. MATERIALS AND METHODS
The flowchart describing the whole pipeline of the current study is illustrated in Fig. (1), which is a comparative and subtractive proteomics systemic workflow for the identification of outer membrane, periplasmic and extracellular proteins with the potential for vaccine development (2.1.1 to 2.1.8) and Fig. (2), which is, reverse vaccinology workflow for identification of antigenic protein, epitope prediction, construction of chimeric vaccine and its in silico validation (2.2.1 – 2.2.15).
2.1. Comparative and Subtractive Proteomics Workflow (Fig. 1)
2.1.1. Collection of Proteome Data
The list of all available strains of Burkholderia pseudomallei was downloaded from the UNIPROT server. Burkholderia pseudomallei (strain K96243) is the reference strain. Twenty other non-redundant proteomes were taken in the study. Shared proteins between proteomes and reference proteomes were found against CD-hit-2D available on the CD-HIT server [35]. All the shared proteins were then compiled as a single fasta file and taken for further analysis.
2.1.2. Identification and Removal of Duplicate Proteins
To identify the duplicate proteins by clustering techniques, subtractive analysis (Fig. 1) of protein was done using CD-HIT [35]. Sequence identity cut-off was fixed at 0.6 or 60% identity because sequences with more than 60% identity had similar structures and functions [35]. The alignment of the amino acids was done using the Global sequence identity algorithm. Alignment coverage was done by selecting a bandwidth of 20 amino acids and default parameters.
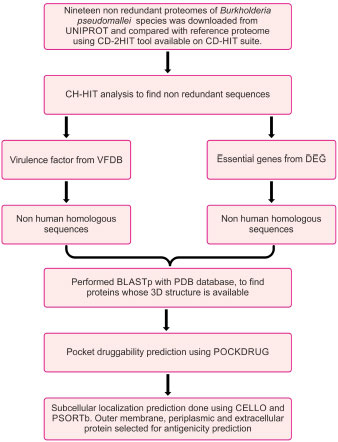
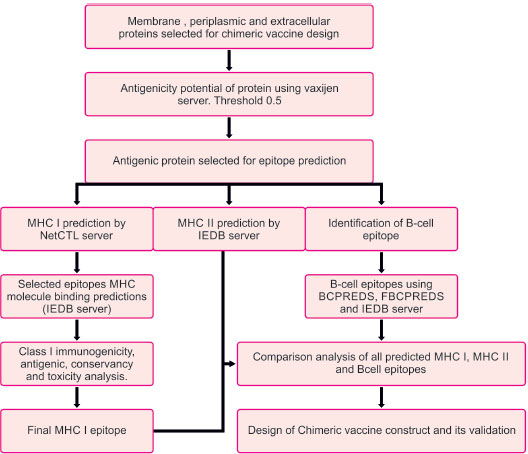
2.1.3. Screening of Essential Proteins using the TID Tool
The database of essential genes (DEG) [36] has essential protein-coding genes determined by genome-wide gene essentiality analysis. Essential proteins were screened out by BLASTp of non-redundant sequences against the DEG database using the TID tool [37]. The e value used was 10-5 and a bit score of 100.
2.1.4. Screening of Virulence Factors using the TID Tool
The host defense mechanism of bacteria is modulated and degraded by virulence factors under adhesion, colonization, and invasion. A BLASTp of CD-HIT result with the VFDB database [38] using TID [37] with an e value of 10-3 was done.
2.1.5. Screening of Proteins which are Non-homologous to Humans
The above three independent searches yielded a comprised list of proteins. The BLASTp search of the above proteins was done against the non-redundant protein sequence (nr) database of the host Homo sapiens (taxid:9606) using the TID tool [37], with a bit score of 100 and a cut-off value of 10-3. The purpose of comparing proteins with human host proteins was to find non-human homologous proteins of pathogens. This activity will help in the design of pathogen-specific therapeutics.
2.1.6. Identification of Proteins with PDB Structure which are Non-homologous to Humans
BLASTp of non-homologous proteins to humans was done against the PDB database of Burkholderia pseudomallei (strain K96243).
2.1.7. Identification of Proteins with Druggable Pockets
PockDrug (http://pockdrug.rpbs.univ-paris-diderot.fr) [39] web servers were used to predict possible cavities in the protein structure, prioritizing those located near or on their Pleckstrin Homology domain (PH).
2.2. Reverse Vaccinology Workflow
2.2.1. Prediction of Antigenic Protein
The subtractive proteomics approach helps us identify outer membrane, periplasmic, and extracellular proteins with potential vaccine development. The antigenic properties of these selected proteins were deciphered using the Vaxijen web server [42]. A threshold value of 0.5 was considered to be a potent antigenic protein. These identified potent antigenic proteins were taken for further analysis (Fig. 2).
2.2.2. T-Cell MHC Class I Epitope Prediction
Prediction of MHC class I epitopes of selected proteins was made by the NetCTL server [43]. We chose epitopes based on a high overall combinatorial score, and a prediction threshold value of 0.75 was set to identify epitopes. Various prediction method has been integrated, like TAP transporter efficiency with antigen processing, proteasomal cleavage, and MHC I affinity predictor. Scores obtained by each method were merged, and a combined score of epitopes was achieved
2.2.3. MHC I Binding Prediction
MHC I binding prediction for epitopes was made using the Immune epitope database analysis resource (IEDB AR) [44]. The default prediction method is IEDB recommended, and it uses the consensus method consisting of ANN [45], SMM [46], CombLib [47], and NetMHCpan [48].
IC50 value and percentile rank were used to select the identified T-cell epitope with HLA alleles. A lower percentile rank indicates a higher interaction between the MHC molecule and peptide epitope. MHC I binding prediction of predicted T cell epitope was done using the IEDB server. The predicted epitope with higher affinity (IC<200nm) and percentile rank (<=0.2) was taken for Class I immunogenicity prediction.
2.2.4. Class I Immunogenicity Prediction
Epitopes or MHC complexes are supposed to have the ability to trigger an immune response. Hence, the MHCI immunogenicity prediction tool using the IEDB server [49] was used. Immunogenicity prediction was made, keeping the default parameters. After the immunogenicity prediction, the epitopes that had a positive value were taken for further analysis.
2.2.5. Analysis of Antigenicity, Conservancy, and Toxicity of Predicted Epitopes
The immunogenicity tool yielded a list of promiscuous epitopes, which were further analyzed by the Vaxijen version 2.0 server for their antigenicity, keeping a threshold of 0.5. IEDB conservancy analysis [50] was used to assess the conservancy level of epitopes within genotype sequences. Default sequence identity parameters were used for the purpose. This analysis aims to calculate the epitope's degree of conservancy within a protein sequence [51]. The physicochemical property of epitopes was analyzed using the ToxinPred online server tool to predict the toxicity level. This analysis uses default parameters to confirm that the host cell immune response targets only bacteria instead of host tissue [52].
2.2.6. T Cell MHC Class II Epitope Predictions
IEDB-AR server was used to predict T-cell epitope binding to class II MHC molecule. The consensus method was used to compute T-cell epitopes [53, 54]. Moreover, this consensus prediction approach used a combination of the average relative binding matrix method and stabilization matrix alignment method.
2.2.7. Analysis of Antigenic and Toxicity Behavior of Predicted Epitopes
Antigenicity and toxicity analysis of MHC class I and MHC class II epitopes obtained by the above prediction was done. VaxiJen version 2.044 [42] and ToxinPred [52] tools were used for antigenicity and toxicity analysis, respectively. Vaxijen (threshold >0.5)uses the physiochemical behavior of epitopes to predict its antigenic behavior. The toxinpred tool also analyzed whether the induced specific immune response would target only bacterial cells or host cells.
2.2.8. B-Cell Epitope Prediction
Prediction of linear B-cell epitope for proteins was achieved. Epitopes were predicted by BCPREDS [55], FBCPREDS [56], and BEPIPRED servers [57]. Then, we did the antigenicity prediction using the vaxijen server. The epitope with a higher value of antigenicity >0.5 was selected for analysis.
2.2.9. Comparative Prediction of MHC I, MHC II, and B-Cell Epitopes along with Physiochemical Analysis
B-cell epitopes, MHC-I epitopes, and MHC II epitopes were compared, and then the final vaccine construct was designed using these epitopes. For chimeric vaccines, all epitopes should have a hydrophilic nature; otherwise, the epitopes will not be able to illicit /induce an immune response in human cells. The Protparam tool [58] was used to find the GRAVY score. A Positive GRAVY score is indicative that the protein is hydrophobic, while a negative score indicates that the protein is hydrophilic. All negative value ones were selected.
2.2.10. Construction of Multiepitope Chimeric Vaccine
All the selected OMP epitopes, i.e., HTL, CTL, and B cell epitopes, were joined together using amino acid linkers (HEYGAEALERAG and GGGS linkers) to design a chimeric vaccine. Different adjuvants were joined using 'EAAAK' linkers at both termini (N and C) to enhance the immunogenicity of constructs. Adjuvants used were 50s ribosomal L7/L12 protein [59], beta-defensin [60], HBHA protein (M. tuberculosis, accession no. AGV15514.1), and HBHA conserved sequence [61] respectively. A non-natural pan DR(PADRE) sequence was also combined with adjuvants to improve the vaccine potency and efficacy. This PADRE is a 13 amino acid epitope (AKVAAWTLKAAAC) that induces CD4+ T-cells.
2.2.11. Allergenicity, Antigenicity, and Solubility Prediction of Vaccine Construct
Four vaccine constructs (V1, V2, V3, V4) were made. Vaccine constructs were analyzed based on their allergenicity, antigenicity, and soluble prediction methods to select a suitable vaccine. Allergenicity analysis was done using the AlgPred server [62]. Nevertheless, two servers were used to predict antigenicity, viz. ANTIGENpro [63] and VaxiJen 2.044 server [42]. The solubility of the vaccine constructs and the probability (≥0.5) were also predicted using the SOLpro server [64].
2.2.12. Prediction of Various Physiochemical Properties of Vaccine Constructs using PROTPARAM Tool
Vaccine constructs physiochemical properties were characterized using the Expasy ProtParam server. The physicochemical properties of the vaccine constructs were characterized using the Expasy ProtParam server [58]. The Protparam server yields information regarding PI values, aliphatic index, instability index, molecular weight, number of amino acids, and GRAVY score. The instability index of the protein predicts protein stability (<40). The Aliphatic index explains the thermostability of protein, whereas proteins' hydrophilic and hydrophobic nature is explained by GRAVY values.
2.2.13. Prediction of Secondary Structure of Vaccine Construct
Vaccine constructs (V1, V2, V3) were used for predicting its secondary structure parts using the PSIPRED v3.3 program [65]. It predicts structure with 81.6% accuracy. Components can be alpha, beta-helix, and coil.
2.2.14. Molecular Docking and Molecular Dynamics Simulation
All four vaccine constructs were modeled using the Phyre2 online tool [66]. RCSB-PDB was used to download the PDB ID of all the HLA alleles. HLA alleles used were 1A6A, 1XRS, 2Q6W, and 6J1W. Molecular docking of the HLA alleles with four vaccine constructs was done using PatchDock [67] to show HLA-peptide interactions. The Fire Dock (Fast Interaction Refinement in Molecular Docking) server was used to refine and reverse the rigid body molecular docking score. Firelock gives the best ten solutions for final refinement. The refined models were based on global; binding energy and binding score. Also, the Clus Pro server [68] was used for docking the vaccine construct (V1) with TLR4/MD2 complex (PDB ID 2z65). GROMACS was used for molecular dynamics simulation of the V1-TLR4 complex.
2.2.15. Codon Optimization and in Silico Cloning of Vaccine Constructs
To adapt the codon usage of vaccines to the E. coli host strain, the codon adaptation tool (JCAT) was used [69]. An amino acid sequence of the vaccine was translated backward to DNA sequence and was adapted for codon usage to E. coli. CAI values are the basis for adaptation, which was calculated by applying an algorithm. We avoided prokaryotic ribosome binding sites, rho-independent transcription terminators, and cleavage sites of some restriction enzymes. The adapted gene sequence of the final vaccine construct was cloned in the E. coli pET28a vector using the SnapGene tool [70] to ensure the expression of the vaccine construct.
3. RESULTS
3.1. Shortlisted B. Pseudomallei Strains
There are many strains of selected organisms in UNIPROT, but we took only 20 non-redundant proteomes, including the reference proteome of Burkholderia pseudomallei (strain K96243). Using CD-HIT 2d, we could find out the shared protein between the proteome and reference proteome. We removed redundant sequences by CD-HIT analysis, leaving only non-redundant sequences.
3.2. Identification of Essential Proteins of B. Pseudomallei
DEG result revealed that 2027 proteins of B.pseudomallei were essential, so we kept these and discarded the rest. These 2027 essential proteins are necessary for the survival of Burkholderia pseudomallei. Blocking these bacterial proteins will destroy this micro-organism.
3.3. Identification of Virulence Factors of B.Pseudomallei
The virulence factor database (VFDB) result revealed that 1796 proteins were associated with the virulence of B. pseudomallei. These proteins are also an important target for inhibiting the pathogenesis of B. pseudomallei. The search for novel VFs is important as virulence factors explore the significance of pathogen in various diseases. These identified protein targets will be more important for screening prominent antibiotics and helpful in drug discovery.
3.4. Identification of Non-human Homologous Proteins in B.Pseudomallei
In this work, a total of 1446 VF proteins and 1411 essential proteins were identified, which are non-human homologous proteins. The remaining 350 VF's and 616 essentials showed similarity with human proteins; therefore, they filtered out. Detection of non-human homologous proteins helps to find the candidate proteins, which can be helpful for screening and drug development.
3.5. BLASTp with PDB Database
After BLAST's structure database analysis, we retrieved five essential proteins and seven virulence proteins with their structures from PDB. Manual analysis comparison showed that there were at least three proteins that are common (4RLH, 4CFI, 5X9Q) in both essential and virulence proteins.
3.6. Pocket Druggability Prediction
Pocket druggability prediction showed that all 9 proteins (2XBL, 4RLH, 4CFI, 5X9Q, 5WNN, 4JGB, 4HCN,4UTI and, 4USM) were druggable and had druggability scores above 0.5., as shown in Table 1.
3.7. Subcellular Localization Prediction and Antigenicity Prediction
Prediction of subcellular localization was essential as we learned about the druggable protein's biological location. Extracellular, periplasmic, and outer membrane proteins were considered probable vaccine candidates. We found 4CFI, 5WNN, 4HCN, and 4UTI to be potential vaccine candidates. This was also confirmed by antigenicity prediction by the vaxijen server, where all four had antigenicity scores above 0.5. These four proteins are selected for chimeric vaccine design. Results are shown in Tables 2 and 3.
PDB ID | Number of Pockets | Number of Druggable Pockets | Best Druggable Pocket Score |
---|---|---|---|
2XBL | 27 | 7 | 0.99 |
4RLH | 34 | 27 | 1.0 |
4CFI | 4 | 4 | 0.88 |
5X9Q | 24 | 13 | 0.99 |
5WNN | 23 | 13 | 1.0 |
4JGB | 12 | 9 | 0.99 |
4HCN | 12 | 2 | 0.81 |
4UT1 | 38 | 14 | 0.99 |
4USM | 19 | 10 | 0.8 |
PDB ID | PSORTb | CELLO |
---|---|---|
2XBL | Cytoplasmic | Cytoplasmic |
4RLH | Cytoplasmic membrane | Cytoplasmic |
4CFI | Extracellular | extracellular |
5X9Q | Cytoplasmic | Cytoplasmic |
5WNN | Periplasmic | Periplasmic |
4JGB | Unknown | Cytoplasmic |
4HCN | Cytoplasmic | Outer membrane |
4UT1 | Extracellular | Extracellular |
4USM | cytoplasmic | cytoplasmic |
Table 3.
PDB ID | Names | Antigenicity |
---|---|---|
4CFI | 3D structure of FliC from Burkholderia pseudomallei | 0.6542 antigen |
5WNN | Crystal structure of Phosphate-binding protein PstS protein from Burkholderia pseudomallei | 0.7290 antigen |
4HCN | Crystal structure of Burkholderia pseudomallei effector protein CHBP in complex with ubiquitin | 0.5191 antigen |
4UTI | The structure of the flagellar hook junction protein FlgK from Burkholderia pseudomallei | 0.5822 antigen |
3.8. Selection of Potent T-cell MHC-I Epitopes
Best T-cell epitopes were predicted for four shortlisted proteins by the NetCTL server based on a high combinatorial score. We set the prediction threshold value at 0.75. The software identified 7 epitopes in 4CFI, 8 in 4HCN, 33 in 4UTI, and 7 in 5WNN.
MHC-I binding prediction of all the T cell epitopes was made using the IEDB server. We selected epitopes with high affinity (IC<200nm) and percentile rank (<=0.2) for class I immunogenicity prediction. 2 out of 7 in 4CFI, 4 out of 8 in 4HCN, 15 out of 33 in UTI, and 1 out of 7 in 5WNN were selected for further analysis. Table 4.
3.9. Class I Immunogenicity Prediction
We subjected epitopes selected above to IEDB immunogenicity prediction. The epitope's immunogenicity score ranged from -0.44395 to 0.18585. High immunogenicity score shows a high ability to stimulate naïve T cells and induce cellular immunity. 2 out of 2 in 4CFI, 2 out of 4 in 4HCN, 6 out of 15 in 4UTI, and 0 out 1 in 5WNN had positive scores, so we selected these (2+2+6+0=10) epitopes for further analysis. See Table 4.
Protein PDB ID | Peptide | Interacting MHC I Allele | Class I Immunogenicity |
---|---|---|---|
5WNN | YAKKNNMVY | HLA-B*15:01 (0.8) HLA-B*35:01 (1.5) |
-0.42347 |
4CFI | LSSTAVTAV | HLA-A*68:02 (1.1) | 0.11794 |
- | SSTAVTAVF | HLA-B*58:01 (0.5) HLA-B*15:01 (0.7) |
0.18585 |
4HCN | LTQEPRTAY | HLA-B*15:01 (1.0) | 0.15669 |
- | SLDELNQLL | HLA-A*02:01 1.2 | -0.01318 |
- | KLRFASHEY | HLA-B*15:01 (0.5) HLA-A*30:01 (0.8) |
0.10277 |
- | TLDSHKNYV | HLA-A*02:01 (1.0) | -0.33839 |
4UTI | TTSDYALSY | HLA-B*15:01 (2.0) | -0.10417 |
- | TSATTPVPY | HLA-B*35:01 (1.1) | 0.10748 |
- | ISNAATPGY | HLA-B*58:01 (0.7) HLA-B*15:01 (0.9) HLA-B*35:01 (1.1) |
0.12235 |
- | LLDQRDLAV | HLA-B*08:01 (0.8) HLA-A*02:01 (2.0) |
-0.02458 |
- | SLSTYYTLV | HLA-A*02:01 (0.5) | 0.00456 |
- | SAQPGPTQY | HLA-B*35:01 (1.7) | -0.06112 |
- | SSAAQTALV | HLA-A*68:02 (0.5) | 0.00235 |
- | QLVAAGQQY | HLA-B*15:01 (1.0) | -0.04267 |
- | ALDGFSLAI | HLA-A*32:01 (0.5) HLA-A*02:01 (1.3) |
0.01307 |
- | FAVGAPAVY | HLA-B*35:01 (0.1) HLA-B*53:01 (1.2) HLA-B*15:01 (1.2) |
0.1323 |
- | QSNGNYSVF | HLA-B*15:01 (1.0) HLA-B*58:01 (1.2) |
-0.09328 |
- | NTGSATLSV | HLA-A*68:02 (0.48) | -0.18685 |
- | GSATLSVSF | HLA-A*32:01 (0.7) HLA-B*58:01 (0.9) |
-0.17659 |
- | SQGSVSAGY | HLA-B*15:01 (0.22) | -0.21818 |
- | TQGSSLSTY | HLA-B*15:01 (0.5) | -0.44395 |
3.10. Toxicity, Conservancy, and Antigenicity Prediction
We used the toxinpred tool to determine if the epitopes were non-toxic. We found that all ten epitopes were non-toxic. We also analyzed selected epitopes' conservancy using the IEDB conservancy analysis tool. Selection of Epitopes was done with more than 50% conservancy for further analysis. It was found that all epitopes were 100% conserved. We made the antigenicity prediction of epitopes using the vaxijen server. Results showed that a total of 2 epitopes of 4CFI (LSSTAVTAV and SSTAVTAVF), two epitopes of 4HCN (LTQEPRTAY and KLRFASHEY), and three epitopes of 4UTI (SSAAQTALV, ALDGFSLAI, and FAVGAPAVY) had antigenicity score above 0.5 and hence were highly antigenic. After that, we selected one epitope of each protein with the highest antigenicity score for further hydrophobicity analysis. SSTAVTAVF of 4CFI, KLRFASHEY of 4HCN, and ALDGFSLAI of 4UTI were selected. The result is shown in Table 5.
3.11. MHC II Epitope Prediction
The four selected proteins were subjected to MHC II epitope prediction using the IEDB server. After that, antigenicity and toxicity analysis led to the shortlisting of 9 epitopes in 4CFI, 14 in 4HCN, 14 in 5WNN, and 13 in 4UTI, which were non-toxic and antigenic. Thereafter, one epitope in each protein with the highest antigenicity score was selected for further hydrophobicity analysis. QINVVSDGKGGFTFT in 4CFI, KVDIKKLHLDGKLRF in 4HCN, EPKTETF KAAAAGAN in 5WNN, and QSVNSQLTDTVTQIN in 4UTI were selected—results in Tables 6-9.
Protein Name | Peptide Epitopes | Toxicity (SVM score) | Antigenicity | Conservancy |
---|---|---|---|---|
4CFI | LSSTAVTAV | Non-toxin (-1.02) | 0.7342 antigen | 100% |
- | SSTAVTAVF | Non toxin (-1.08) | 0.7876 antigen | 100% |
4HCN | LTQEPRTAY | Non toxin(-1.45) | 0.5702 antigen | 100% |
- | KLRFASHEY | Non toxin (-1.06) | 0.7290 antigen | 100% |
4UTI | TSATTPVPY | Non toxin (-1.04) | 0.2237 non antigen | 100% |
- | ISNAATPGY | Non toxin (-0.87) | 0.2937 non antigen | 100% |
- | SLSTYYTLV | Non-toxin (-1.09) | 0.3229 non-antigen | 100% |
- | SSAAQTALV | Non toxin(-0.56) | 0.6819 antigen | 100% |
- | ALDGFSLAI | Non toxin (-0.97) | 1.579 antigen | 100% |
- | FAVGAPAVY | Non-toxin (-1.28) | 0.7139 antigen | 100% |
Allele | Toxicity(SVM score) | Antigenicity | Start | End | Peptide | Percentile_rank |
---|---|---|---|---|---|---|
HLA-DRB1*03:01 | -0.93 Non-Toxin | 0.9294 (Probable ANTIGEN) | 202 | 216 | ETTQINVVSDGKGGF | 0.03 |
HLA-DRB1*03:01 | -0.94 Non-Toxin | 1.1150 (Probable ANTIGEN). | 203 | 217 | TTQINVVSDGKGGFT | 0.03 |
HLA-DRB1*03:01 | -1.14 Non-Toxin | 1.1178 (Probable ANTIGEN). | 204 | 218 | TQINVVSDGKGGFTF | 0.03 |
HLA-DRB1*03:01 | -1.02 Non-Toxin | 1.2455 (Probable ANTIGEN). | 205 | 219 | QINVVSDGKGGFTFT | 0.03 |
HLA-DRB1*03:01 | -1.04 Non-Toxin | 0.8924 (Probable ANTIGEN). | 206 | 220 | INVVSDGKGGFTFTD | 0.03 |
HLA-DQA1*01:02/DQB1*06:02 | -0.40 Non-Toxin | 0.6162 (Probable ANTIGEN). | 265 | 279 | ATDQANATAMVAQIN | 0.04 |
HLA-DQA1*01:02/DQB1*06:02 | -0.28 Non-Toxin | 0.6597 (probable ANTIGEN) | 266 | 280 | TDQANATAMVAQINA | 0.04 |
HLA-DQA1*01:02/DQB1*06:02 | -0.50 Non-Toxin | 0.6322 (Probable ANTIGEN). | 264 | 278 | SATDQANATAMVAQI | 0.06 |
HLA-DRB4*01:01 | --1.29 Non Toxin | 0.1580 (Probable NON-ANTIGEN). | 85 | 99 | TNSLQRIRQLAVQAS | 0.06 |
HLA-DRB4*01:01 | -1.30 Non-Toxin | 0.0761 (Probable NON-ANTIGEN) . |
86 | 100 | NSLQRIRQLAVQASN | 0.06 |
HLA-DRB4*01:01 | -1.36 Non-Toxin | 0.4018 (probable NON-ANTIGEN) | 87 | 101 | SLQRIRQLAVQASNG | 0.08 |
HLA-DQA1*01:02/DQB1*06:02 | -0.39 Non- Toxin | 0.4076 (probable NON-ANTIGEN) | 267 | 281 | DQANATAMVAQINAV | 0.09 |
HLA-DRB4*01:01 | -1.33 Non- Toxin | -0.0658 (Probable NON-ANTIGEN) . |
84 | 98 | LTNSLQRIRQLAVQA | 0.11 |
HLA-DRB4*01:01 | -1.23 Non- Toxin | -0.0302 (probable NON-ANTIGEN) | 83 | 97 | SLTNSLQRIRQLAVQ | 0.13 |
HLA-DRB4*01:01 | -1.30 Non- Toxin | 0.2310 (Probable NON-ANTIGEN). | 88 | 102 | LQRIRQLAVQASNGP | 0.14 |
HLA-DRB1*07:01 | -1.05 Non- Toxin | 0.3738 (Probable NON-ANTIGEN) . |
68 | 82 | NDGVSILQTASSGLT | 0.18 |
HLA-DRB1*07:01 | -0.88 Non- Toxin | 0.2619 (probable NON-ANTIGEN) | 67 | 81 | ANDGVSILQTASSGL | 0.2 |
HLA-DRB1*07:01 | -1.33 Non- Toxin | 0.3539 (Probable NON-ANTIGEN). | 70 | 84 | GVSILQTASSGLTSL | 0.2 |
HLA-DQA1*01:02/DQB1*06:02 | -0.41 Non-Toxin | 0.5635 (probable ANTIGEN) | QANATAMVAQINAVN | 0.2 | ||
HLA-DRB1*08:02 | -1.26 Non- Toxin | 0.3494 (probable NON-ANTIGEN) | 323 | 337 | QNRFTAIATTQQAGS | 0.2 |
Allele | Toxicity(SVM score) | Antigenicity (score) | Start | End | Peptide | Percentile_rank |
---|---|---|---|---|---|---|
HLA-DRB1*03:01 | -1.31Non-Toxin | 0.5212 (Probable ANTIGEN) | 296 | 310 | IKKLHLDGKLRFASH | 0.01 |
HLA-DRB1*03:01 | -1.07Non-Toxin | 1.3367 (Probable ANTIGEN) | 293 | 307 | KVDIKKLHLDGKLRF | 0.02 |
HLA-DRB1*03:01 | -1.13Non-Toxin | 1.1233 (Probable ANTIGEN). | 294 | 308 | VDIKKLHLDGKLRFA | 0.02 |
HLA-DRB1*03:01 | -1.24Non-Toxin | 0.7486 (Probable ANTIGEN). | 295 | 309 | DIKKLHLDGKLRFAS | 0.02 |
HLA-DRB1*03:01 | -1.22Non-Toxin | 0.5526 (Probable ANTIGEN). | 297 | 311 | KKLHLDGKLRFASHE | 0.02 |
HLA-DRB1*03:01 | -1.02Non-Toxin | 0.7110 (Probable ANTIGEN) | 298 | 312 | KLHLDGKLRFASHEY | 0.03 |
HLA-DRB1*03:01 | -0.93Non-Toxin | 0.6783 (Probable ANTIGEN). | 299 | 313 | LHLDGKLRFASHEYD | 0.03 |
HLA-DRB1*11:01 | -1.35Non-Toxin | 0.6535 (Probable ANTIGEN | 273 | 287 | PDDVQMRLLASILQI | 0.09 |
HLA-DRB1*11:01 | -1.37Non-Toxin | 0.8034 (Probable ANTIGEN). | 274 | 288 | DDVQMRLLASILQID | 0.09 |
HLA-DRB1*11:01 | -1.26 Non- Toxin | 0.3477 (Probable NON-ANTIGEN). | 275 | 289 | DVQMRLLASILQIDK | 0.09 |
HLA-DRB1*11:01 | -1.43 Non-toxin | 0.1986 (Probable NON-ANTIGEN). | 276 | 290 | VQMRLLASILQIDKD | 0.09 |
HLA-DRB1*03:01 | -1.12 Non- Toxin | 0.0178 (Probable NON-ANTIGEN). | 197 | 211 | HKNYVVIVNDGRLGH | 0.09 |
HLA-DRB1*03:01 | -1.12 Non- Toxin | 0.3948 (Probable NON-ANTIGEN). | 198 | 212 | KNYVVIVNDGRLGHK | 0.09 |
HLA-DRB1*03:01 | -0.94Non-Toxin | 0.6503 (Probable ANTIGEN). | 199 | 213 | NYVVIVNDGRLGHKF | 0.09 |
HLA-DRB1*03:01 | -0.92Non-Toxin | 0.6535 (Probable ANTIGEN). | 200 | 214 | YVVIVNDGRLGHKFL | 0.09 |
HLA-DRB1*03:01 | -0.93Non-Toxin | 0.7029 (Probable ANTIGEN). | 201 | 215 | VVIVNDGRLGHKFLI | 0.09 |
HLA-DRB1*11:01 | -1.76 Non- Toxin | 0.4055 (Probable NON-ANTIGEN) | 272 | 286 | MPDDVQMRLLASILQ | 0.1 |
HLA-DRB1*03:01 | -0.81Non-Toxin | 0.8333 (Probable ANTIGEN) | 202 | 216 | VIVNDGRLGHKFLID | 0.14 |
HLA-DRB1*03:01 | -0.88Non-Toxin | 1.0533 (Probable ANTIGEN) | 203 | 217 | IVNDGRLGHKFLIDL | 0.15 |
Allele | Toxicity (SVM score) | Antigenicity (score) | Start | End | Peptide | Percentile_ rank |
---|---|---|---|---|---|---|
HLA-DRB1*09:01 | -1.66Non-Toxin | 0.4175 (Probable NON-ANTIGEN). | 62 | 76 | VTVERQYNQYLSNQL | 0.05 |
HLA-DRB1*09:01 | -1.62Non-Toxin | 0.5096 (Probable ANTIGEN) | 63 | 77 | TVERQYNQYLSNQLN | 0.05 |
HLA-DRB1*09:01 | -1.50 Non- Toxin | 0.4736 (Probable NON-ANTIGEN) | 64 | 78 | VERQYNQYLSNQLNA | 0.05 |
HLA-DRB1*09:01 | -1.49Non-Toxin | 0.5434 (Probable ANTIGEN). | 65 | 79 | ERQYNQYLSNQLNAA | 0.05 |
HLA-DRB1*09:01 | -1.32Non-Toxin | 0.7859 (Probable ANTIGEN) | 433 | 447 | ANGSAIAAASPVLAA | 0.08 |
HLA-DRB1*09:01 | -1.31Non-Toxin | 0.5757 (Probable ANTIGEN). | 434 | 448 | NGSAIAAASPVLAAG | 0.08 |
HLA-DRB1*09:01 | -1.46Non-Toxin | 0.5611 (Probable ANTIGEN). | 435 | 449 | GSAIAAASPVLAAGV | 0.08 |
HLA-DRB1*09:01 | -1.51 Non- Toxin | 0.4833 (Probable NON-ANTIGEN). | 436 | 450 | SAIAAASPVLAAGVA | 0.08 |
HLA-DQA1*01:02/DQB1*06:02 | -1.17 Non- Toxin | 0.3981 (Probable NON-ANTIGEN). | 430 | 444 | LAIANGSAIAAASPV | 0.08 |
HLA-DQA1*01:02/DQB1*06:02 | -1.11 Non- Toxin | 0.4440 (Probable NON-ANTIGEN). | 429 | 443 | SLAIANGSAIAAASP | 0.1 |
HLA-DRB3*01:01 | -1.14Non-Toxin | 0.6805 (Probable ANTIGEN). | 156 | 170 | RQSVNSQLTDTVTQI | 0.11 |
HLA-DRB3*01:01 | -1.23Non-Toxin | 0.8551 (Probable ANTIGEN). | 157 | 171 | QSVNSQLTDTVTQIN | 0.11 |
HLA-DRB3*01:01 | -1.07Non-Toxin | 0.7808 (Probable ANTIGEN). | 158 | 172 | SVNSQLTDTVTQINS | 0.11 |
HLA-DRB3*01:01 | -1.13Non-Toxin | 0.5874 (Probable ANTIGEN). | 159 | 173 | VNSQLTDTVTQINSY | 0.11 |
HLA-DRB3*01:01 | -1.21 Non- Toxin | 0.4986 (Probable NON-ANTIGEN) | 160 | 174 | NSQLTDTVTQINSYT | 0.11 |
HLA-DQA1*05:01/DQB1*03:01 | -1.18 Non- Toxin | 0.4397 (Probable NON-ANTIGEN) | 431 | 445 | AIANGSAIAAASPVL | 0.11 |
HLA-DQA1*05:01/DQB1*03:01 | -1.17 Non- Toxin | 0.3981 (Probable NON-ANTIGEN). | 430 | 444 | LAIANGSAIAAASPV | 0.12 |
HLA-DQA1*05:01/DQB1*03:01 | -1.24 Non- Toxin | 0.4904 (Probable NON-ANTIGEN). | 432 | 446 | IANGSAIAAASPVLA | 0.12 |
HLA-DQA1*05:01/DQB1*03:01 | -1.32Non-Toxin | 0.7859 (Probable ANTIGEN). | 433 | 447 | ANGSAIAAASPVLAA | 0.12 |
HLA-DQA1*01:02/DQB1*06:02 | -1.18 Non- Toxin | 0.4397 (Probable NON-ANTIGEN). | 431 | 445 | AIANGSAIAAASPVL | 0.15 |
HLA-DRB4*01:01 | 0.95 Non- Toxin | 0.0697 (Probable NON-ANTIGEN). | 634 | 648 | EAANLMQYQQLYQAN | 0.15 |
HLA-DQA1*01:02/DQB1*06:02 | -1.31Non-Toxin | 0.6585 (Probable ANTIGEN). | 428 | 442 | FSLAIANGSAIAAAS | 0.16 |
HLA-DQA1*05:01/DQB1*03:01 | -1.11 Non- Toxin | 0.4440 (Probable NON-ANTIGEN) | 429 | 443 | SLAIANGSAIAAASP | 0.16 |
HLA-DRB1*09:01 | -1.31 Non- Toxin | 0.3079 (probable NON-ANTIGEN) | 474 | 488 | TTLAYNAASKTLSGF | 0.17 |
HLA-DRB1*09:01 | -1.11Non-Toxin | 0.5803 (Probable ANTIGEN). | 472 | 486 | GTTTLAYNAASKTLS | 0.18 |
HLA-DRB1*09:01 | -1.25 Non- Toxin | 0.4118 (Probable NON-ANTIGEN). | 475 | 489 | TLAYNAASKTLSGFP | 0.18 |
HLA-DRB1*09:01 | -1.20Non-Toxin | 0.6347 (Probable ANTIGEN). | 473 | 487 | TTTLAYNAASKTLSG | 0.19 |
Allele | Toxicity (SVM score) | Antigenicity (score) | Start | End | Peptide | Percentile Rank |
---|---|---|---|---|---|---|
HLA-DPA1*01/DPB1*04:01 | -1.23Non-Toxin | 0.6013 (Probable ANTIGEN). | 266 | 280 | GKEAWPVVGATFVLL | 0.01 |
HLA-DPA1*01/DPB1*04:01 | -1.30Non-Toxin | 0.5675 (Probable ANTIGEN) | 267 | 281 | KEAWPVVGATFVLLH | 0.01 |
HLA-DPA1*01/DPB1*04:01 | -1.34Non-Toxin | 0.5568 (Probable ANTIGEN) | 268 | 282 | EAWPVVGATFVLLHA | 0.01 |
HLA-DPA1*01/DPB1*04:01 | -1.08Non-Toxin | 0.6616 (Probable ANTIGEN). | 269 | 283 | AWPVVGATFVLLHAK | 0.01 |
HLA-DPA1*01/DPB1*04:01 | -1.02Non-Toxin | 0.5595 (Probable ANTIGEN). | 270 | 284 | WPVVGATFVLLHAKQ | 0.01 |
HLA-DRB1*09:01 | -1.27Non-Toxin | 0.8929 (Probable ANTIGEN). | 238 | 252 | EPKTETFKAAAAGAN | 0.07 |
HLA-DPA1*01/DPB1*04:01 | -1.35Non-Toxin | 0.5532 (Probable ANTIGEN). | 271 | 285 | PVVGATFVLLHAKQD | 0.07 |
HLA-DPA1*01/DPB1*04:01 | -1.17Non-Toxin | 0.6022 (Probable ANTIGEN). | 272 | 286 | VVGATFVLLHAKQDK | 0.07 |
HLA-DQA1*04:01/DQB1*04:02 | -0.78Non-Toxin | 0.6989 (Probable ANTIGEN). | 9 | 23 | AGLAGALFAVAAHAD | 0.07 |
HLA-DRB1*09:01 | -1.22Non-Toxin | 0.5219 (Probable ANTIGEN). | 239 | 253 | PKTETFKAAAAGANW | 0.08 |
HLA-DRB1*09:01 | -1.22Non-Toxin | 0.6325 (Probable ANTIGEN) | 240 | 254 | KTETFKAAAAGANWS | 0.08 |
HLA-DRB1*09:01 | -1.20 Non- Toxin | 0.4477 (Probable NON-ANTIGEN). | 241 | 255 | TETFKAAAAGANWSK | 0.08 |
HLA-DRB1*01:01 | -1.13 Non- Toxin | 0.2367 (Probable NON-ANTIGEN). | 5 | 19 | QTAFAGLAGALFAVA | 0.09 |
HLA-DQA1*04:01/DQB1*04:02 | -0.89Non-Toxin | 0.7895 (Probable ANTIGEN). | 10 | 24 | GLAGALFAVAAHADI | 0.1 |
HLA-DQA1*04:01/DQB1*04:02 | -1.05Non-Toxin | 0.7103 (Probable ANTIGEN) | 11 | 25 | LAGALFAVAAHADIT | 0.1 |
HLA-DQA1*05:01/DQB1*03:01 | -1.20 Non-Toxin | 0.4477 (Probable NON-ANTIGEN). | 241 | 255 | TETFKAAAAGANWSK | 0.11 |
HLA-DPA1*01:03/DPB1*02:01 | -0.82 Non- Toxin | 0.4440 (Probable NON-ANTIGEN). | 158 | 172 | GSGTSFIWTNYLSKV | 0.12 |
HLA-DQA1*05:01/DQB1*03:01 | -1.21 Non- Toxin | 0.3770 (Probable NON-ANTIGEN). | 242 | 256 | ETFKAAAAGANWSKS | 0.12 |
HLA-DQA1*05:01/DQB1*03:01 | -1.19 Non- Toxin | 0.3860 (Probable NON-ANTIGEN). | 243 | 257 | TFKAAAAGANWSKSF | 0.12 |
HLA-DRB1*04:05 | -1.50 Non- Toxin | 0.1557 (Probable NON-ANTIGEN). | 252 | 266 | NWSKSFYQILTNQPG | 0.12 |
HLA-DRB1*04:05 | -1.42 Non- Toxin | 0.3352 (Probable NON-ANTIGEN) | 253 | 267 | WSKSFYQILTNQPGK | 0.12 |
HLA-DRB1*04:05 | -1.27 Non-Toxin | 0.6026 (Probable ANTIGEN). | 254 | 268 | SKSFYQILTNQPGKE | 0.12 |
HLA-DRB1*04:05 | -1.37 Non-Toxin | 0.6240 (Probable ANTIGEN). | 255 | 269 | KSFYQILTNQPGKEA | 0.12 |
HLA-DPA1*01:03/DPB1*02:01 | -0.93 Non- Toxin | 0.2636 (Probable NON-ANTIGEN) | 159 | 173 | SGTSFIWTNYLSKVN | 0.14 |
HLA-DPA1*01/DPB1*04:01 | -0.93 Non- Toxin | 0.2636 (Probable NON-ANTIGEN) | 159 | 173 | SGTSFIWTNYLSKVN | 0.14 |
HLA-DPA1*01/DPB1*04:01 | -0.91 Non- Toxin | 0.2126 (Probable NON-ANTIGEN). | 160 | 174 | GTSFIWTNYLSKVND | 0.14 |
HLA-DQA1*05:01/DQB1*03:01 | -1.22 Non-Toxin | 0.6325 (Probable ANTIGEN). | 240 | 254 | KTETFKAAAAGANWS | 0.14 |
HLA-DQA1*04:01/DQB1*04:02 | -0.92 Non- Toxin | 0.4504 (Probable NON-ANTIGEN). | 8 | 22 | FAGLAGALFAVAAHA | 0.14 |
HLA-DPA1*01/DPB1*04:01 | -0.82 Non- Toxin | 0.4440 (Probable NON-ANTIGEN). | 158 | 172 | GSGTSFIWTNYLSKV | 0.16 |
HLA-DPA1*01:03/DPB1*02:01 | --0.56 Non-Toxin | 0.8813 (Probable ANTIGEN). | 157 | 171 | DGSGTSFIWTNYLSK | 0.18 |
HLA-DPA1*01:03/DPB1*02:01 | -0.91 Non- Toxin | 0.2126 (Probable NON-ANTIGEN). | 160 | 174 | GTSFIWTNYLSKVND | 0.18 |
HLA-DRB1*01:01 | -1.38 Non- Toxin | 0.3206 (Probable NON-ANTIGEN). | 4 | 18 | MQTAFAGLAGALFAV | 0.19 |
HLA-DQA1*04:01/DQB1*04:02 | -0.99 Non-Toxin | 0.8033 (Probable ANTIGEN) | 12 | 26 | AGALFAVAAHADITG | 0.19 |
Protein | BCPRED | Start | Antigenicity | FBCPred | Start | Antigenicity | BEPIPRED | Start | Antigenicity |
---|---|---|---|---|---|---|---|---|---|
4CFI | DPCGTDASAPGGAKSVSIVQ | 396 | 0.9791 (probable ANTIGEN) | EDPCGTDASAPGGA | 395 | 1.2901 (probable ANTIGEN) | AFDEDPCGTDASAPGGAKS | 392 | 1.2053 (probable ANTIGEN) |
4CFI | VFGSSTAGTGTAASPSFQTL | 234 | 1.1854 (probable ANTIGEN) | GSSTAGTGTAASPS | 236 | 1.9710 (probable ANTIGEN) | GSSTAGTGTAASPSF | 236 | 1.9410 (probable ANTIGEN) |
4HCN | SSAATSPAGPLGGLPARSSS | 36 | 0.5827 (probable ANTIGEN) | AATSPAGPLGGLPA | 38 | 0.1331 (probable ANTIGEN) | INNVGKTGQAGGETERIPSTEPLGSSAAT- SPAGPLGGLPARSSSISNTNRTGENPM |
12 | 0.8203 (probable ANTIGEN) |
- | SNTNRTGENPMITPIISSNL | 57 | 1.0913 (probable ANTIGEN) | SNTNRTGENPMITP | 57 | 1.4813 (probable ANTIGEN) | INNVGKTGQAGGETERIPSTEPLGSSAAT- SPAGPLGGLPARSSSISNTNRTGENPM |
12 | 0.8203 (probable ANTIGEN) |
- | DVPIDPTSIEYLENTSFAEH | 171 | 0.2266 (probable NON-ANTIGEN) | TEKDVPIDPTSIEY | 168 | 0.6727 (probable ANTIGEN) | DVPIDPTSIE | 171 | 1.0831 (probable ANTIGEN) |
- | QSLSGESSNRVMWNDRYDTL | 94 | 0.7454 (probable ANTIGEN) | GESSNRVMWNDRYD | 98 | 0.8637 (probable ANTIGEN) | SGESSN | 97 | 2.7352 (probable ANTIGEN) |
4UTI | NITSATTPVPYDPSKGASMT | 505 | 0.4465 (probable NON-ANTIGEN) | TSATTPVPYDPSKG | 507 | 0.1948 (probable NON-ANTIGEN) | VTIAGTPPTSINITSATTPVPYDPSK- GASMTISSTTQPAPSGVM |
494 | 0.5384 (probable ANTIGEN) |
- | SKGVAGSAQPGPTQYLPDVS | 258 | 0.8982 (probable ANTIGEN) | GVAGSAQPGPTQYL | 260 | 1.0484 (probable ANTIGEN) | VAGSAQPGPTQYLP | 261 | 0.9731 (probable ANTIGEN) |
- | WGLTTTGQNISNAATPGYSV | 18 | 0.7917 (probable ANTIGEN) | WGLTTTGQNISNAATPGYSV | 18 | 0.7917 (probable ANTIGEN) | TTGQNISNAATPGYSVERPVYAEA- SGQYTSSGYLPQGVSTV |
22 | 0.6516 (probable ANTIGEN) |
- | GTPADGDQFTIGANKGTNDG | 546 | 1.4694 (probable ANTIGEN) | GTPADGDQFTIGAN | 546 | 1.4373 (probable ANTIGEN) | SLSGTPADGDQFTIGANKGT- NDGRN |
543 | 1.4773 (probable ANTIGEN) |
- | AVGAPAVYANQNNTGSATLS | 332 | 0.9549 (probable ANTIGEN) | AVYANQNNTGSATL | 337 | 1.0837 (probable ANTIGEN) | AVYANQNNTGSAT | 337 | 1.2373 (probable ANTIGEN) |
- | TVANNAADPSARQTAMSNAQ | 120 | 0.6727 (probable ANTIGEN) | TVANNAADPSARQT | 120 | 0.8115 (probable ANTIGEN) | VANNAADPSARQTAMS | 121 | 0.5948 (probable ANTIGEN) |
- | DGTQPTTSDYALSYDGAKYT | 356 | 1.0344 (probable ANTIGEN) | QPTTSDYALSYDGA | 359 | 0.8989 (probable ANTIGEN) | VDGTQPTTSDYALSY | 355 | 1.1391 (probable ANTIGEN) |
5WNN | EGTTVNWPTGTGGKGNDGVA | 182 | 1.8714 (probable ANTIGEN) | TTVNWPTGTGGKGN | 184 | 1.7655 (probable ANTIGEN) | NDEWKSKVGEGTTVNWPTGTGG- KGNDGV |
173 | 1.7688 (probable ANTIGEN) |
3.12. B-cell Epitope Prediction
To elicit humoral immunity, an epitope should be identified by B-lymphocytes. Prediction of B-cell epitopes was made by different servers like BCPREDS, FBCPREDS, and Bepipred server. B cell epitopes were predicted by different servers, like Epitopes predicted by three servers were selected. Thereafter, we made the antigenicity prediction, and epitopes with a higher value of antigenicity greater than 0.5 were selected for further hydrophobicity analysis. Results are shown in Table 10.
3.13. Comparative Prediction of Epitopes and Further Hydropathy Analysis
The final chimeric vaccine sequence was designed after a manual comparative analysis of B-cell epitopes, MHC I epitopes, and MHC II epitopes. Thereafter, GRAVY score analysis of epitopes using the Protparam tool was done. A positive GRAVY score means the protein is hydrophobic, and a negative value indicates the protein is hydrophilic. Epitopes should be hydrophilic (present on the surface); otherwise, they will not be able to induce an immune response in the host cell- indicated in Table 11.
3.14. Construction of Chimeric Vaccine
To construct a chimeric vaccine, all the predicted B cell, MHC I, and MHC II epitopes were joined by amino acid linkers (HEYGAEALERAG and GGGS). Adjuvants were linked to the construct using EAAAK linkers at both N and C terminus to enhance the immunogenicity of the construct. Adjuvants used were 50s ribosomal L7/L12 protein, beta-defensin, HBHA protein (M. tuberculosis, accession number. AGV15514.1), and HBHA conserved sequence. To overcome the problem caused by polymorphism of HLA-DR molecules in the worldwide population and to improve the vaccine efficacy and potency, a non-natural pan DR (PADRE) sequence is also added using HEYGAEALERAG and GGGS linkers. The amino acid sequence of PADRE is AKVAAWTLKAAA. A total of 4 vaccine construct was made, as in Table 12.
3.15. Allergenicity, Antigenicity, and Solubility Prediction of Designed Vaccine Construct
The four vaccine constructs, V1, V2, V3, and V4, were further analyzed using AlgPred, ANTIGENPRO, vaxijen, and SOLpro server. The predicted vaccine construct score indicates that V1, V2, and V3 are non-allergic, whereas V4 was found to be allergic and hence dropped from the analysis. Antigenicity of V1, V2, V3, and V4 was further predicted by ANTIGENpro and VAXIJEN server. The predicted antigenicity value of more than 0.90 and .75 in vaxijen was a good score and indicated the good antigenic nature of the four vaccines construct. All four showed a solubility score above 0.7, which indicates that the vaccine construct will be highly soluble during its heterologous expression in E. coli. All results are in Table 13.
Protein | MHC 1 Epitopes | Hydrophobicity | MHC II Epitopes | Hydrophobicity | B Cell Epitopes | Hydrophobicity |
---|---|---|---|---|---|---|
4CFI | SSTAVTAVF | 1.311 | QINVVSDGKGGFTFT | 0.047 | GSSTAGTGTAASPS | -0.193 |
4HCN | KLRFASHEY | -0.978 | KVDIKKLHLDGKLRF | -0.520 | SGESSN | -1.633 |
4UTI | ALDGFSLAI | 1.533 | QSVNSQLTDTVTQIN | -0.533 | SLSGTPADGDQFTIGANKGTNDGRN | -1.020 |
5WNN | -none | -none | EPKTETFKAAAAGAN | -0.660 | EGTTVNWPTGTGGKGNDGVA | -0.770 |
Vaccine | Construct Sequences |
---|---|
Vaccine Construct 1 with HBHA adjuvant (V1) |
EAAAKMAENPNIDDLPAPLLAALGAADLALATVNDLIANLRERAEETRAETRTRVEERRA- RLTKFQEDLPEQFIELRDKFTTEELRKAAEGYLEAATNRYNELVERGEAALQRLRSQTAF- EDASARAEGYVDQAVELTQEALGTVASQTRAVGERAAKLVGIELEAAAKAKFVAAWTLKA- AAHEYGAEALERAGKLRFASHEYGGGSKVDIKKLHLDGKLRFGGGSQSVNSQLTDTVTQI- NGGGSEPKTETFKAAAAGANGGGSGSSTAGTGTAASPSGGGSSGESSNGGGSSLSGTPAD- GDQFTIGANKGTNDGRNGGGSEGTTVNWPTGTGGKGNDGVAHEYGAEALERAGAKFVAAW- TLKAAAHEYGAEALERAG |
Vaccine construct 2 with HBHA conserved adjuvant (V2) |
EAAAKMAENSNIDDIKAPLLAALGAADLALATVNELITNLRERAEETRRSRVEESRARLT- KLQEDLPEQLTELREKFTAEELRKAAEGYLEAATSELVERGEAALERLRSQQSFEEVSAR- AEGYVDQAVELTQEALGTVASQVEGRAAKLVGIELEAAAKAKFVAAWTLKAAAHEYGAEA- LERAGKLRFASHEYGGGSKVDIKKLHLDGKLRFGGGSQSVNSQLTDTVTQINGGGSEPKT- ETFKAAAAGANGGGSGSSTAGTGTAASPSGGGSSGESSNGGGSSLSGTPADGDQFTIGAN- KGTNDGRNGGGSEGTTVNWPTGTGGKGNDGVAHEYGAEALERAGAKFVAAWTLKAAAHEY- GAEALERAG |
Vaccine construct 3 with BETA DEFENSIN adjuvant (V3) |
EAAAKGIINTLQKYYCRVRGGRCAVLSCLPKEEQIGKCSTRGRKCCRRKKEAAAKAKFVA- AWTLKAAAHEYGAEALERAGKLRFASHEYGGGSKVDIKKLHLDGKLRFGGGSQSVNSQLT- DTVTQINGGGSEPKTETFKAAAAGANGGGSGSSTAGTGTAASPSGGGSSGESSNGGGSSL- SGTPADGDQFTIGANKGTNDGRNGGGSEGTTVNWPTGTGGKGNDGVAHEYGAEALERAGA- KFVAAWTLKAAA HEYGAEALERAG |
Vaccine construct 4 with 50s ribosomal L7/L12 adjuvant (V4) |
EAAAKMAKLSTDELLDAFKEMTLLELSDFVKKFEETFEVTAAAPVAVAAAGAAPAGAAVEA- AEEQSEFDVILEAAGDKKIGVIKVVREIVSGLGLKEAKDLVDGAPKPLLEKVAKEAADEAK- AKLEAAGATVTVKEAAAKAKFVAAWTLKAAA HEYGAEALERAGKLRFASHEYGGGSKVDI- KKLHLDGKLRFGGGS QSVNSQLTDTVTQINGGGSEPKTETFKAAAAGANGGGSGSSTAGT- GTAASPSGGGSSGESSNGGGS SLSGTPADGDQFTIGANKGTNDGRNGGGS EGTTVNWPT- GTGGKGNDGVAHEYGAEALERAGAKFVAAWTLKAAA HEYGAEALERAG |
Vaccine Construct | ALGPRED | ANTIGENpro | VAXIJEN | SOLpro |
---|---|---|---|---|
V1 | Non-allergen | 0.859882 | 1.1665 | 0.974576 |
V2 | Non allergen | 0.884183 | 1.1826 | 0.980100 |
V3 | Non allergen | 0.919282 | 1.4418 | 0.964183 |
V4 | allergen | 0.926492 | 1.1456 | 0.982857 |
Vaccine Construct | MOL WT. | PI | Gravy | Aliphatic Index | Instability Index | Negative Amino Acid | Positive Amino Acid |
---|---|---|---|---|---|---|---|
VI | 39001.67 (378 AA) |
5.15 | -0.512 | 68.44 | 28.03 | 54 | 42 |
V2 | 37883.45 (369 AA) |
5.05 | -0.486 | 70.87 | 32.08 | 54 | 40 |
V3 | 26534.22 (264 AA) |
9.26 | -0.554 | 54.58 | 21.30 | 25 | 34 |
V4 | 34813.51 (349 AA) |
5.15 | -0.262 | 71.29 | 71.29 | 48 | 37 |
3.16. Physiochemical Analysis of Vaccine Construct
The physicochemical properties of the vaccine construct were analyzed using the PROTPARAM server. The molecular weight was between 26 to 39 kDa. GRAVY values were found to be negative values, which shows the hydrophilic nature of the vaccine construct. Stability at different temperatures of vaccine constructs is indicated by a high aliphatic index range (58 to 71.29). Also, V1, V2, and V3 had an instability score below 40, which indicates that protein has good stability to induce immunogenic reactions. Total positive and negative amino acids were also indicated in the Table 14.
3.17. Structure Prediction of Selected Vaccine Construct
Secondary structure prediction of the final three vaccine constructs (V1, V2, V3) was predicted using the PSIPRED server. The secondary structure is shown in Figs. (3-5). The structure of all vaccine constructs has a helix, strand, and coil.
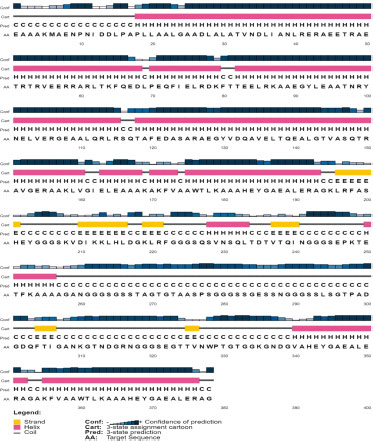
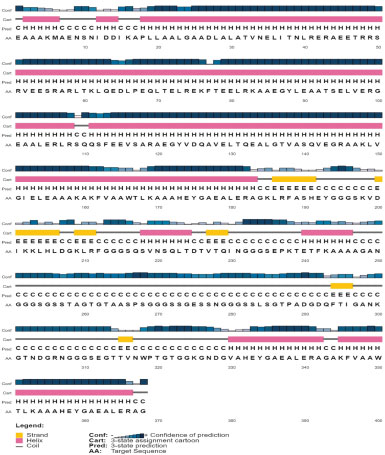
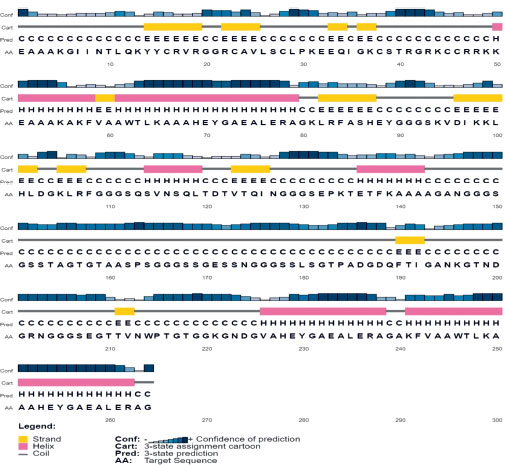
The 3-D models of V1, V2, and V3 were generated using the Phyre2 tool and were validated by Ramachandran plot analysis. The modeled structure of the V1 and Ramachandran plots has been shown in the figure. 83.64% of residues are in the allowed region, as shown in Figs. (6, 7).
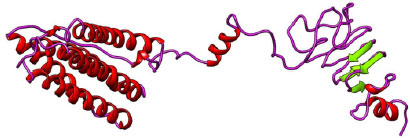
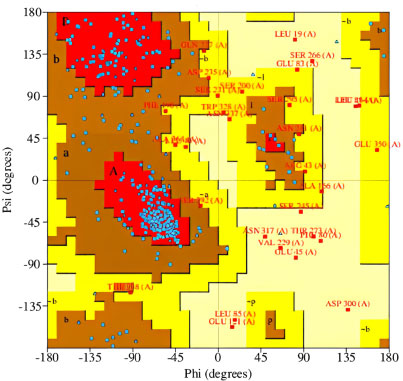
Vaccine Constructs | HLA Allele PDB ID | Score | Area | Hydrogen Bond Energy | Global Energy | ACE |
---|---|---|---|---|---|---|
V1 | 1A6A | 19756 | 3090.10 | -2.40 | -11.45 | 5.07 |
- | 1XR8 | 16426 | 2784.50 | -2.24 | -12.88 | 6.28 |
- | 2Q6W | 17566 | 3001.90 | -3.06 | -12.88 | 5.55 |
- | 6J1W | 17780 | 2307.30 | -4.18 | -5.26 | 7.95 |
V2 | 1A6A | 16338 | 1865.0 | -5.14 | -53.53 | 7.80 |
- | 1XR8 | 18626 | 3338.0 | 0.00 | 8.66 | 0.68 |
- | 2Q6W | 17640 | 2423.2 | -1.97 | 9.56 | 11.82 |
- | 6J1W | 21846 | 3122.7 | -1.47 | 7.13 | 5.76 |
V3 | 1A6A | 14968 | 2066.20 | -2.21 | -3.94 | 4.69 |
- | 1XR8 | 15660 | 2149.10 | -2.72 | 3.62 | 11.70 |
- | 2A6A | 15008 | 2220.40 | -1.28 | -8.10 | 0.88 |
- | 6J1W | 15972 | 2260.90 | -1.42 | -14.36 | 10.28 |
3.18. Docking of V1, V2, and V3 with HLA Alleles Protein
HLA allele of the human population interacts with vaccines. To explore this, we have docked V1, V2, and V3 with 4 different alleles i.e. 1A6A, 1XR8, 2Q6W, and 6J1W. V1 has the lowest global binding energy value with different alleles, i.e., 1A6A (HLA-DR B1*03:01); -11.45, 1XR8 (HLA-B*15:01); -12.88, 2Q6W (HLA-DR B3*01:01); -12.88 and 6J1W (HLA-A*30:01); -5.26 as shown in Table 15. We have analyzed all three different constructs and finalized the V1 suitable., which can be developed to control Burkholderia pseudomallei.
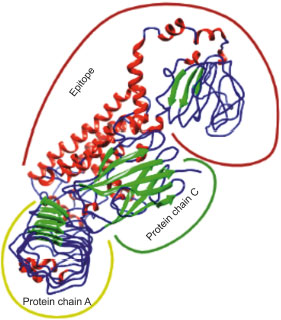
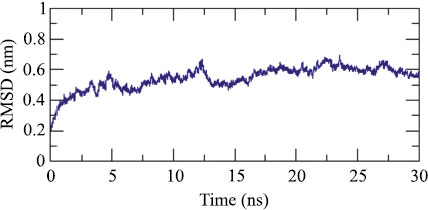
3.19. Docking and Molecular Dynamics Simulation of V1 with TLR4
Adjuvant attached to vaccine construct interacts with TLR4. Hence, we performed an interaction study between V1 and TLR4/MD2 Complex (2Z65). Patchdock result indicated negative (-2.03) binding energy that suggests good interaction between V1 and TLR/MD2 complex. This interaction was also studied using Cluspro. The best-modeled ClusPro complex has been shown in the figure and has a minimum energy score of -1136.1, which explains the interaction of V1 with the TLR/MD2 complex. Best docked complex interaction was further validated by molecular dynamics simulation by GROMACS. RMSD graph shows that complex stabilizes after 20 ns as shown in Figs. (8, 9).
3.20. In Silico Cloning of Chimeric Vaccine Construct V1 for its Heterologous Expression in E. coli
Cloning of chimeric multivalent vaccine and its expression within the expression vector pET28a were analyzed by Java Codon Adaptation Tool. Reverse translation generates a cDNA sequence that will be used in silico cloning. Codon optimization analysis of V1 showed 53.88% GC content of the construct. The CAI value of V1 was 0.988, indicating heterologous expression of a selected gene, which will be highly expressed in E. coli cells. The DNA sequence of restriction sites of EcoR1 and BMT1 were added at 5' and 3' end, respectively. V1 was in silico cloned into the pET28a vector for its heterologous expression in E. coli using EcoR1 and BMT1 restriction enzymes, as shown in Fig. (10).
4. DISCUSSION
Burkholderia pseudomallei is the cause of Melioidosis in humans and animals. Melioidosis is prevalent in subtropical and tropical climates like Thailand and Australia. However, it is an emerging infection in India, mostly affecting rural males who are either diabetic or alcoholic and are at risk for contracting this disease. A common presentation of the disease was sepsis with bacteremia and localized disease involving focal abscesses and joints [71]. Burkholderia pseudomallei is resistant to many antibiotics, which include polymixins, macrolides, aminoglycosides, and β-lactams. Hence, the treatment is often intensive and prolonged, with greater chances of being unsuccessful. There are chances of recurrence, too, which can range from 13% to 26%, depending on the kind of antibiotic being chosen to treat primary infection. Approximately 75% of cases relapse instead of re-infection [16]. There is no approved vaccine against Whitmore disease.
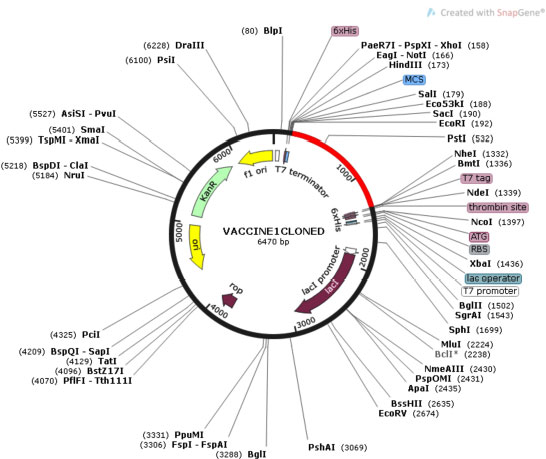
The availability of genome information of the pathogen and recent progress in immunoinformatics has assisted researchers in developing the Chimeric multiepitope vaccine. We have employed subtractive and comparative proteomics and reverse vaccinology approaches to design a chimeric vaccine in the present study. Twenty non-redundant proteomes and one reference proteome (K96243) were taken. Further analysis with the CD-HIT 2D server found the shared proteins; after that, the redundant sequence was also removed. Further subtractive proteomics analysis was done using the TID tool. Virulence factors were non-human homologous, and essential non-human homologous proteins were found. After structure database analysis using BLASTp, five essential and seven virulence protein with 3D structure in the PDB database was identified. The manual comparison showed that there were at least three proteins that are both essential and virulent, viz. 4RLH, 4CFI, 5X9Q. Pocket druggability prediction of all nine proteins, 2XBL, 4RLH, 4CFI, 5X9Q, 5WNN, 4JGB, 4HCN, 4UTI, and 4USH, revealed that all druggability scores above 0.5 and hence were druggable. Subcellular localization prediction was made, after which we selected outer membrane proteins, extracellular or periplasmic. Antigenicity prediction using the vaxijen server also led to shortlisting four antigenic proteins and must be taken for further analysis.
After this, the prediction of MHC I, MHC II, and B-cell epitope was made using various servers. Our focus was to identify antigenic, non-allergic, and non-toxic epitopes. All the selected epitopes were joined using amino acid linkers HEYGAEALERAG and GGGS linkers. EAAAK linkers attached adjuvants at both N and C terminus to enhance the immunogenicity of the vaccine construct. A non-natural pan DR (PADRE) sequence was combined with adjuvants, which induce CD4+ Tcell and improve the vaccine efficacy and potency.4 vaccine constructs (V1, V2, V3, V4) were made. Thereafter, antigenicity, allergenicity, and solubility prediction of vaccine constructs were made, and V4 was dropped from further analysis as it was an allergen and also had an instability score of more than 40 in physiochemical analysis by the protparam server. V1, V2, and V3 were taken for further analysis. Docking of all three vaccine constructs with 4 HLA alleles 1A6A, 1XR8, 2Q6W, and 6J1W was done. V1 showed the lowest global binding energy values for all four alleles and the best binding score among the three vaccines and, hence, was chosen for further analysis. Interaction of final vaccineV1 with TLR/MD2 complex was done, followed by molecular dynamics simulation showing that the complex was stable after 20 ns. V1 was further cloned in silico into the pET28a vector for its heterologous expression in E. coli using EcoR1 and BMT1 restriction enzymes. We added adjuvants, Pan-DR epitopes, and linkers with the multiepitope sequence in the vaccine construct. The multiepitope sequence mixes MHC I, MHC II, and B cell epitopes. We have carefully selected components that will be significant in inducing. B. pseudomallei-specific immune response. Therefore, we have included all possible factors that might induce the immunogenicity and feasibility of vaccine constructs V1. Additional in vitro and in vivo study is required to demonstrate the effectiveness of the proposed vaccine.
CONCLUSION
The availability of the proteome of B.pseudomallei has made this study possible through the usage of various in silico approaches. We could shortlist vaccine targets using subtractive proteomics and then construct chimeric vaccines using reverse vaccinology and immunoinformatics approaches. Subtractive proteomics led to identifying antigenic outer membrane, extracellular, and periplasmic proteins, which can be suitable vaccine candidates. MHC I, MHC II, and B-cell epitope prediction of antigenic proteins were made thereafter. Constructing a chimeric vaccine by merging the epitopes with different adjuvants and linkers was done to enhance the immune response and improve the effectiveness of chimeric vaccine V1. In silico validation of the vaccine construct was also done. This research has opened opportunities for experimental research on B. Pseudomallei vaccine production. This also provides a systematic pipeline for researchers to design chimeric vaccine constructs against other pathogens. The final vaccine V1 construct needs to be validated in an animal model before use against B.pseudomallei.
LIST OF ABBREVIATIONS
BLAST | = Basic Local Alignment Search Tool |
PDB | = Protein Data Bank |
PADRE | = Pan DR-binding Epitope |
HLA | = Human Leucocyte Antigen |
TLR4 | = Toll Like Receptor 4 |
CDC | = Centers for Disease Control and Prevention |
ATCSA | = Anti-terrorism, Crime and Security Act 2001 |
OMP | = Outer Membrane Protein |
CD-HIT | = Cluster Database at High Identity with Tolerance |
DEG | = Databse of Essential Genes |
TID | = Target iDentification |
VFDB | = Virulence Factor Database |
IEDB-AR | = Immune Epitope Database- Analysis Resource |
BCPRED | = B-cell Epitope Prediction |
GRAVY | = Grand Average of Hydropathy |
HBHA | = Heparin-Binding Hemagglutinin Adhesin |
ETHICS APPROVAL AND CONSENT TO PARTICIPATE
Not applicable.
HUMAN AND ANIMAL RIGHTS
No animals/humans were used in this research.
CONSENT FOR PUBLICATION
Not applicable.
AVAILABILITY OF DATA AND MATERIALS
The authors confirm that the data supporting the findings of this research are available within the article.
FUNDING
SM received a fellowship from DBT INDIA.
CONFLICT OF INTEREST
Dr. Salman Akhtar is Associate editorial board member of The Open Bioinformatics Journal.
ACKNOWLEDEGMENTS
The author would like to thank DBT INDIA for the fellowship to SM. The authors are grateful to the research and development committee of Integral University for providing the necessary infrastructure and support. Manuscript Communication number: IU/R&D/2022-MCN0001604.