Aims and Scope
Editor's Choice
Network Biology Approaches to Identify the Drug Lead Molecule for Neurodevelopmental Disorders in Human
Archana Verma, Shweta Singh Chauhan, Vaishali Pankaj, Neha Srivastva, Prachi Srivastava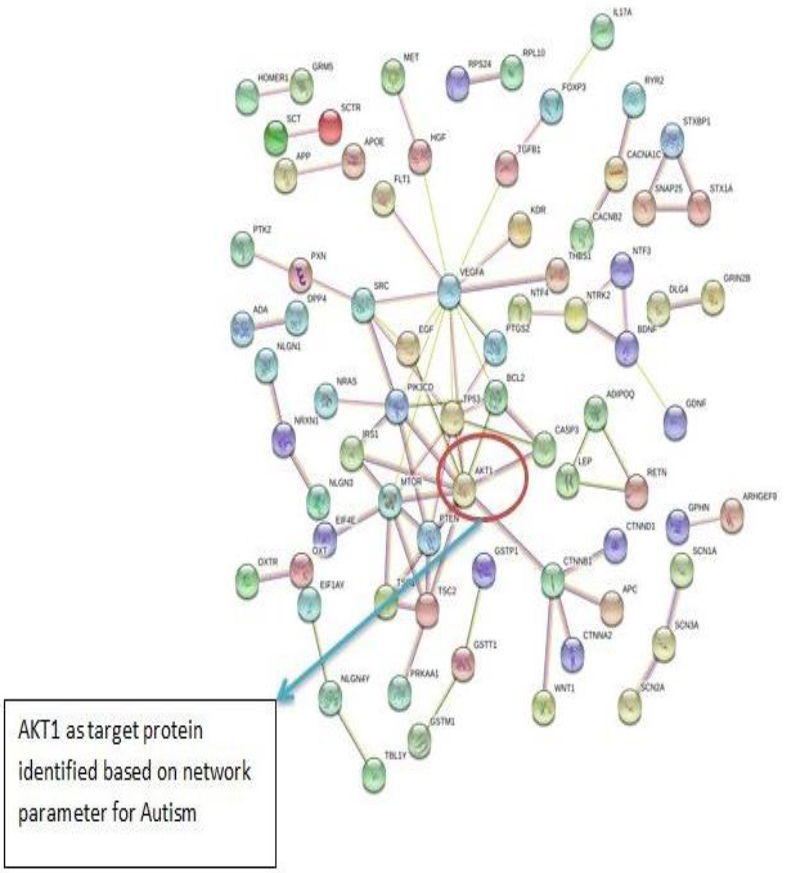
Aims:
To identify most novel drug target and lead molecule for neurodevelopmental disorder Autism, Intellectual Disability (ID) and Attention Deficit Hyperactivity Disorder (ADHD) diseases through system biology approaches
Background:
Neurodevelopmental disorders (NNDs) are disabilities associated chiefly with the functioning of the neurological system and brain. Children with neurodevelopmental disorders have difficulties with speech, behaviour, learning and other neurological functions. Systems biology is a holistic approach to enciphering the complexity of biological systems and their interactions. It opens the way to a more successful discovery of novel therapeutics.
Objective:
To identify most novel drug target and lead molecule for neurodevelopmental disorder Autism, Intellectual Disability (ID) and Attention Deficit Hyperactivity Disorder (ADHD) diseases through system biology approaches.
Methods:
A list of genes was collected from NCBI database for Autism, Intellectual Disability (ID) and Attention Deficit Hyperactivity Disorder (ADHD) diseases. STRING database and Cytoscape software was used for construction and interpreting molecular interaction in the network. 3D structure of target protein, was build and validated.The phytochemicals were identified through various research articles and filtered out by virtual screening through Molinspiration. Molecular docking analyses of known phytochemical with target proteins were performed usingAutoDock tool.
Result:
AKT1 for Autism, SNAP25 for Intellectual Disability (ID) and DRD4 for Attention Deficit Hyperactivity Disorder (ADHD) were identified as most potential drug target through network study. further the modelled structure of obtained target were undergo molecular docking study with kown phytochemicals. Based on lowest binding energy, Huperzine A for Autism and ID, Valerenic acid for ADHD found to be the most potential therapeutic molecules.
Conclusion:
Huperzine A against Autism and ID, Valerenic acid against ADHD found to be the most potential therapeutic molecules and expected to be effective in the treatment of NNDs. Phytochemicals do not have side effects so extract of these can be taken in preventive form too as these disorders occur during developmental stages of the child. Further the obtained molecule if experimentally validated would play promising role for the treatment of NDDs in human.
March 20, 2020
Other Post
- December 12, 2017
Data Mining Approach to Identify Disease Cohorts from Primary Care Electronic Medical Records: A Case of Diabetes Mellitus
January 23, 2015Protein-Protein Interaction Prediction using PCA and SVR-PHCS
December 31, 2014Performances of Bioinformatics Pipelines for the Identification of Pathogens in Clinical Samples with the De Novo Assembly Approaches: Focus on 2009 Pandemic Influenza A (H1N1)
December 31, 2014Identification of the Factors Responsible for the Interaction of Hsp90α and its Client Proteins
January 31, 2013Comparison of Sequencing Utility Programs
July 26, 2013Community Annotation and the Evolution of Cooperation: How Patience Matters