All published articles of this journal are available on ScienceDirect.
Design and Modeling of 4-Anilinoquinazoline Derivatives as Small Molecule Inhibitors of T790M/C797S EGFR Mutations to Abandon the Phenomenon of Tumor Angiogenesis
Abstract
Introduction:
In most types of cancers, specifically, lung cancer, glioblastoma, and breast cancer, the EGFR tyrosine kinase mostly remains in an overactivation state due to the developed mutations in a few specific residues of the kinase domain of protein EGFR.
Methods:
The overexpression of EGFR results in the activation of signaling pathways responsible for the proliferation, growth, metastasis, and neo-angiogenesis in different types of cancers. The different mutations found in cancers expressing the EGFR include L858R, T790, and C797S and other uncommon mutations like S786R, C761X, and L861Q mutations. In the treatment with first-generation EGFR tyrosine kinase inhibitors like erlotinib and gefitinib, cancers have developed secondary resistance due to the development of secondary mutations like T790M.
Results:
It was first proposed that T790M mutations do not block the binding of the inhibitors, but later on, it was found that T790M mutations restore the ATP binding affinity of the kinase domain of EGFR monomers. Therefore, the first-generation inhibitors are not able to bind to T790M mutated EGFR. The second-generation 4-anilino quinazoline-based EGFR inhibitors like dacomitinib and afatinib have shown excellent binding potency with EGFR (T790M) but suffer serious side effects, which urges us to search for new small molecules, which may have the potential to inhibit the tumor angiogenic signals associated with over-activated EGFR.
Conclusion:
In this study, new 4-anilino quinazoline inhibitors as small molecule inhibitors were discovered to target T790M/C797S mutations in EGFR using structure-based virtual screening, docking, and metabolic reactivity studies against the phenomenon of tumor angiogenesis.
1. INTRODUCTION
Tumor angiogenesis, an important mechanism for tumor growth, involves the formation of new blood vessels from pre-existing blood vessels due to the cascading of various pro-angiogenic factors [1]. Various proangiogenic factors include epidermal growth factor receptor, vascular endothelial growth factor, fibroblast growth factor, epidermal growth factor, placental growth factor, matrix metalloproteins (MMPs), and angiopoietin-1 and angiopoietin-2 and their respective receptors known as receptor tyrosine kinases (RTKs) [1]. The other family of biomarkers includes Rho’s family of receptors that are involved in the regulation of proliferation, invasion, and survival. The RhoB has an essential and critical role in governing cell signalling. In signal transduction or cell signalling, which controls the actin cytoskeleton and phagocyte NADPH oxidase, the Rho protein family is essential. Moreover, RhoA, RhoB, and RhoD have been identified to affect membrane trafficking. However, the molecular processes underlying these effects of Rho proteins are not well understood [2, 3]. Furthermore, the implementation of support vector machines and AI-based approaches has validated in many studies that RhoB is a well-known biomarker expressed in rectal cancer [4]. The AI-based approaches have further revealed that the RhoB biomarkers are expressed on NATs in rectal cancer patients [5]. Epidermal growth factor receptor (EGFR) belongs to a family of tyrosine kinases, which play a crucial role in the upregulation of various tumor angiogenic pathways in cancers like Non-small cell lung cancer, glioblastoma, head and neck cancer, bladder cancer, etc [6]. EGFR (Epidermal growth factor receptor) is a receptor tyrosine kinase that is involved in altering the signalling associated with cell progression, apoptosis, neoangiogenesis, and proliferation [7]. If any type of alteration occurs in EGFR-mediated signalling pathways, this will result in the change of the cell from normal to abnormal [8]. The over-expression of EGFR and TGF-α in many carcinomas is correlated with poor prognosis, cancer metastasis, and resistance to chemotherapy [9-11]. The activated EGFR in lung cancer is associated with up-regulation of various signalling molecules like IL-8, HIF, VEGF, bFGF, etc. These signalling molecules, upon binding to their respective receptors, give rise to signalling pathways responsible for the process of angiogenesis [12]. The binding of signalling factors to EGFR receptors initiates different downstream signalling cascades like mitogen-activated protein kinase (MAPK), PI3-AKT/PKB pathway, PLC-gamma pathway, etc. In human cancers, the overexpression of EGFR is the main cause of proliferation, survival, and metastasis of the tumor [13]. The major challenge in NSCLC is the resistance developed by the EGFR due to the recurrence of the mutations that occurred during treatment in cancer patients. The most commonly found mutations in EGFR include L858R (a point mutation in exon 21 of the EGFR kinase domain) and deletions in exon-19. Besides such mutations, many uncommon mutations like S786I, C719S, and L861Q have been observed in NSCLC patients within exon 18-20 of EGFR. Such mutations play a crucial role in growth, proliferation, metastasis, and resistance to apoptotic signalling [13]. In EGFR, the mutation arises due to the alterations mostly in the kinase domain, which comprises tyrosine residue L858R (exon-21 mutation), and exon-19 deletion in the EGFR gene reduces the binding affinity of L858R mutated EGFR with ATP. Erlotinib and gefitinib, the first-generation tyrosine kinase inhibitors, have the potential to inhibit signal transduction pathways initiated by L858R mutant EGFR. Due to the development of secondary mutation T790M in EGFR, the tumor cells develop secondary resistance to first-generation TK inhibitors [14, 15]. T790M EGFR mutation restores the binding affinity of L858R mutant with ATP molecule, which, in turn, results in the failure of first-generation tyrosine kinase inhibitors to bind L858R-T790M EGFR [16, 17]. The second generation inhibitors like dacomitinib and afatinib (4-anilinoquinazoline based second-generation EGFR mutant irreversible inhibitors) have been proven to modify irreversibly the ATP binding site of the kinase domain of EGFR at the Cys797 site. Dacomitinib and afatinib have shown excellent potential against L858R-T790M double EGFR mutants but exhibit many side effects like diarrhoea, mucositis, and ocular toxicity [18, 19].
Furthermore, osimertinib, a third-generation inhibitor, has been developed against the T790M mutation and has minimized off-target effects to a great extent. This characteristic is due to the increasing pharmacodynamics and pharmacokinetics profile of the osimertinib drug. EGFR protein has suffered from another mutation, namely, C797S, a point mutation showing resistance to the third-generation inhibitor, osimertinib. C797S mutation modifies the exact location in the kinase domain of EGFR required for the binding of first and second-generation inhibitors covalently [20, 21]. C797S mutation, which exists within the tyrosine kinase domain of EGFR, is considered to establish a resistance mechanism against osimertinib (A2D9291), rociletinib (CO-1686), and HM61713 (B1142694) that are third generation inhibitors. EA1045 is considered to be the first allosteric TKI to overcome resistance due to T790M and C797S mutations in EGFR tyrosine kinase. EA1045, an allosteric inhibitor, is supposed to be ineffective alone due to receptor dimerization; however, when administered along with cetuximab, it remains effective fully against T790M and C797S mutational resistance. As EA1045 is effective only in combination with cetuximab, this opens up new avenues for researchers to investigate and discover new potential tyrosine kinase inhibitors to overcome the resistance of EGFR [20].
Overcoming these mutations responsible for the drug resistance phenomenon in EGFR, the subject area presents a next-level challenge for researchers to discover the novel broad-line 4- aniline-quinazoline based EGFR inhibitors to combat carcinomas, by especially targeting the expression of EGFR and related tumor angiogenesis in cancers [22].
2. MATERIALS AND METHODS
2.1. Retrieval of Target Receptor and Investigational Phytochemicals
With the aid of structure-based virtual screening, 1000 investigational 4-anilinoquinazoline derivatives were obtained from the PubChem database (https://pubchem.ncbi.nlm. nih.gov/), which were used to prepare a library. The compounds were further allowed to undergo Lipinski’s RO5 filtration to screen out 563 4-anilinoquinazoline compounds possessing drug-likeness. The 2D and 3D structures of the filtered compounds were obtained from the PubChem database in sdf format for future use. Moreover, the crystal structure of the kinase domain of target receptor EGFR (PDB ID: 4I24) having a resolution of 1.80 Å in complex with dacomitinib was retrieved from PDB (protein data bank) database (https://www.rcsb.org/.)
2.2. Target Receptor Preparation
The target of interest was downloaded from the protein data bank in its co-crystallized form having PDB ID: 4I24 (Figs. 1 and 2). The retrieved structure represents the kinase domain of T790M mutated EGFR protein in a complex with dacomitinib drug and water molecules. The ligand and other hetero atoms were removed from the complex using the academic version (v21.1.0.20298) of Biovia Discovery Studio. Furthermore, the polar hydrogens were added to nullify the overall charges on the protein for docking purposes. The 3D structures of the kinase domain of EGFR protein with ligand binding site residues are shown below.
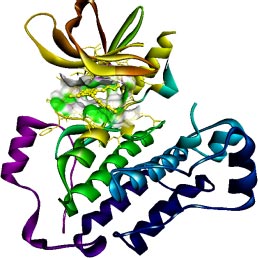
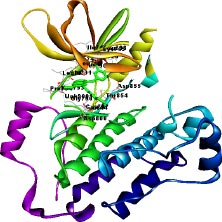
2.3. Ligand Library Preparation
The library of compounds includes all filtered 4-anilino quinazoline derivatives retrieved from the PubChem database in 3D sdf format. The library comprises all 563 4-anilino quinazoline compounds filtered via using Lipinski’s rule of five and ADME filters. The ADME and toxicity profile represents the absorption, distribution, metabolism, excretion, and toxicity of the drug molecules or chemical compounds within the animal body. The ADME profile includes parameters like blood-brain barrier (BBB), HIA (human intestinal absorption), CaCo2, and MDCK cells. The blood-brain barrier is the network of blood vessels and tissues that keeps the brain safe from harmful substances. If the drug is crossing the BBB in excessive concentration then it will be very critical and lethal for the human body to survive. Human intestinal absorption (HIA) is the property that indicates that orally administered drugs are absorbed via the gastrointestinal tract into the bloodstream. The administered drug must possess an HIA feature for its therapeutic effectiveness inside the human body. MDCK cells refer to Madin-Darby canine kidney cells that are used as a model mammalian cell line in various biological and research studies like cell polarity, cell adhesion, and toxicity studies. After performing filtration, we obtained 28 compounds with good ADME and toxicity profiles. All structures were energetically minimized and optimized using an energy minimization program involving AMBER force fields of PyRx virtual screening and USCF Chimera tools while performing the molecular docking with the selected targets.
2.4. Lipinski’s Filters (drug-likeness)
The compounds retrieved using a structure-based virtual screening approach from the database were allowed to undergo Lipinski’s filtration, a rule of 5 given by Christopher. Using Lipinski's rule of five on 1000 4-anilino quinazoline compounds, we obtained 563 compounds that possess drug-likeness and were further selected for ADME and docking studies [23].
2.5. ADME and Toxicity Predictions
After applying the Lipinski filter, all 563 compounds obtained were then allowed to undergo ADME and toxicity filtration. The preADMET tool (https://labworm.com/tool/ preadmet) was used to predict the ADMET properties of all 563 4-anilino quinazoline derivatives and only potential compounds, which favour the value of BBB, CaCo2, HIA, and MDCK ADME parameters, were selected. Following the ADMET filter, we obtained 28 compounds out of 563 compounds with valuable ADMET profiles. The toxicity profile of the selected 28 compounds was also predicted using the same preADMET online server.
2.6. Molecular Docking based Virtual Screening
The virtual screening tool, PyRx v0.8, was utilized to identify the potential compounds based on the binding affinity of the complexes [24]. We performed multiple ligand site-specific docking of all 28 4-anilino quinazoline compounds and control drug, dacomitinib, to the ligand binding site residues of the kinase domain of target protein, 4I24. The binding site residues of the kinase domain include Leu718, Ala743, Lys745, Glu762, Met766, Leu788,Thr790, Gln791, Leu792, Met793, Pro794, Gly796, Leu844, and Thr854. The Autodock vina module of the PyRx virtual screening tool was employed to perform molecular docking studies to filter out the potential compounds. The previously filtered 28 compounds and dacomitinib were docked to the ligand binding site of kinase domain of the target protein, and the docking score was obtained and then analysed. After analyzing the docking score, we obtained only 7 potential compounds possessing binding energy greater than the binding energy of the control drug complex.
2.7. Molecular Docking using AutoDock Vina Module of UCSF Chimera
AutoDock vina module of UCSF Chimera (Academic version 1.15) was employed to perform molecular docking of 7 selected 4-anilino quinazoline derivatives and a control drug, dacomitinib, with the ligand binding site of kinase domain of the target protein. UCSF Chimera is an extensible molecular docking tool; by using its inbuilt Autodock vina module, we perform molecular docking, and it is the fastest and most accurate computational docking tool [25]. The selected compounds and control, dacomitinib, were prepared using the Dock Prep module of the Chimera software. The hydrogen atoms were added to both the species and Gasteiger charges were assigned to nullify the overall charge of the target protein and that of the selected compounds. All standard residues were subjected to AMBER ff14SB to generate different poses for the ligands to interact. The net charges were also specified for both the target proteins and ligands. For most of the proteins, the specified net charge remained zero. The grid box was set up around the ligand binding site of the kinase domain of target protein. The grid box dimensions chosen have values for x= -12.41, y = 41.81, and z = -2.96, and the optimized box size for x =y=z = 25. After generating the grid box size, the docking process was executed and the binding energy of all selected potential compounds and the control drug, dacomitinib, was calculated. Comparing the binding scores, it was found that the three compounds: CID: 12241700 (AQ245), CID: 22417730 (AQ236), and CID: 122417707 (AQ242) had a binding energy greater than that of the control drug, dacomitinib (Figs. 3 and 4).
2.8. Metabolic Reactivity Prediction
SmartCyp 3.0 web server is a sophisticated online web tool to predict the metabolic reactivity of chemical drugs with Cytochrome P450 isoforms while being administered into the body. We used the SMARTCyp 3.0 tool to predict the metabolic reactivity of the top lead and control drug, dacomitinib, as they are assumed to interact with the isoforms of cytochrome enzymes like Cyp3A4, Cyp2D6, and Cyp2C9. The online SMARTCyp 3.0 tool uses the density functional theory to calculate the energies of transition states of the reactive atoms of the compounds. The tool accepts the chemical data in SDF or Mol2 or SMILE format and the output is given for the parameters like score, energy, relative span, 2DSASA (Solvent accessible surface area), span2end, similarity, N+ dist, and COO-dist. The score is the only parameter that is used to rank the atomic sites of the molecule and determine the reactivity of the atoms of the molecule for Cytochrome P450 isoforms. If the value of the energy parameter is 999, then there is no probability of the atomic site being the site of metabolism for CypP450 isoforms. The atoms of the molecule possessing the lowest score are more reactive, so are kept at the top of the table and the atoms having the highest score are kept at the bottom of the table for being the least reactive atomic site. The lowest score is the probability of that atom being the site of metabolism for cytochrome p450 isoforms. The value range from 0 to 1 indicates the high probability of metabolic reactivity. If the value of similarity is equal to 1, it means that the similarity is perfect and the atomic site is reactive, thus showing the metabolic reactivity towards the cytochrome complexes.
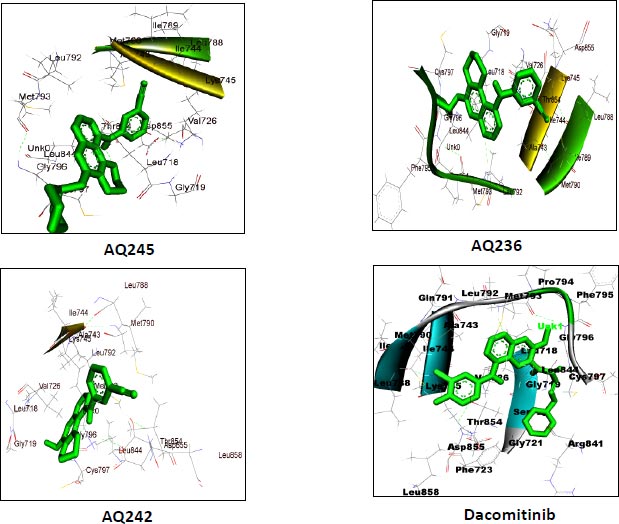
S.No. | Compound | BBB | CaCo2 | HIA | MDCK | logP value |
---|---|---|---|---|---|---|
1 | AQ245 (CID:122417700) | 0.13502 | 42.1628 | 96.320266 | 40.3191 | 3.91049 |
2 | AQ236 (CID:122417730) | 0.0718194 | 26.6161 | 96.282552 | 118.736 | 3.68724 |
3 | AQ242 (CID:122417707) | 0.129112 | 39.6897 | 96.069931 | 56.9512 | 3.72515 |
4 | AQ221 (CID:122417840) | 0.302867 | 23.9717 | 96.210175 | 51.8506 | 4.46783 |
5 | AQ229 (CID:122417770) | 0.0613568 | 26.6533 | 96.320266 | 74.4659 | 4.10129 |
6 | AQ231 (CID:122417767) | 0.193497 | 28.2072 | 96.135421 | 66.1103 | 4.09649 |
7 | AQ46 (CID:71543088) | 0.0524418 | 19.2094 | 96.058509 | 94.1493 | 2.70318 |
8 | Dacomitinib Control | 1.75771 | 39.5278 | 96.060994 | 0.0460513 | -3.54067 |
S.No. | Code | Ames Test | Carcinoma Mouse | Carcinoma Rat | hERG |
---|---|---|---|---|---|
1 | AQ245 (CID:122417700) | Mutagen | Negative | Negative | Medium risk |
2 | AQ236 (CID: 122417730) |
Mutagen | Negative | Negative | Medium risk |
3 | AQ242 (CID:122417707) |
Mutagen | Negative | Negative | Medium risk |
4 | AQ221 (CID:122417840) |
Mutagen | Negative | Negative | Medium risk |
5 | AQ229 (CID:122417770) |
Mutagen | Negative | Negative | Medium risk |
6 | AQ231 (CID:122417767) |
Mutagen | Negative | Negative | Medium risk |
7 | AQ46 (CID:71543088) |
Mutagen | Negative | Negative | Medium risk |
8 | Dacomitinib control | Non-mutagen | Positive | Negative | Medium risk |
3. RESULTS
3.1. Structure-based Virtual Screening
We utilized the structure-based virtual screening approach to screen the PubChem database to retrieve 1000 4-anilinoquinazoline derivatives. The 3D conformers of the retrieved structures were downloaded in sdf (structure data file) format to create a library of compounds. Furthermore, the retrieved compounds were allowed to undergo Lipinski’s rule of five filtrations, and the energy minimization was done during the preparatory phase of the molecular docking process. Moreover, the details of all compounds retrieved were saved by downloading CSV files of the compounds.
3.2. Drug-likeness of Compounds
Lipinski’s RO5 provides a way to filter out the 563 potential compounds possessing drug-likeness. Lipinski’s rule of five includes the parameters like molecular weight <500, hydrogen bond donor <5, hydrogen bond acceptor <10, and logP value <5. Utilizing the rule of five, we obtained only those compounds which possess drug-likeness out of 1000 4-anilino quinazoline derivatives obtained from the PubChem database. By applying Lipinski’s filter on the large library of 1000 compounds, we filtered out 563 compounds possessing drug-likeness properties, which were further screened using the ADME filter approach.
3.3. ADME and Toxicity Prediction
The newly obtained library of 563 filtered compounds was further subjected to undergo ADME filtration. The ADME profile was checked for parameters like BBB, CaCo2, HIA, and MDCK. Based on the value for each ADME parameter, we obtained only 28 compounds out of 563 compounds. Furthermore, the toxicity profiles for the Ames test, carcinoma mice, carcinoma rat, and hERG for 28 potential compounds were obtained using the same preADMET online server 2.0. The ADME and toxicity profiles for the top 7 compounds are given below in Tables 1 and 2.
3.4. Virtual Screening using PyRx Virtual Screening Tool
The AutoDock vina module of PyRx v0.8, a virtual screening tool, was utilized to screen the library of 28 compounds against the drug target, 4I24 (T790M mutated kinase domain of EGFR) to search for potential compounds based on binding affinity. We performed site-specific docking of 28 compounds with the ligand binding site of the kinase domain of EGFR (4I24) tyrosine kinase. The ligands were minimized using the energy minimization program of the PyrX tool to optimize the ligands for docking purposes. The binding site residues chosen for ligand docking include Leu718, Ala743, Lys745, Glu762, Met766, Leu788, Thr790, Gln791, Leu792, Met793, Pro794, Gly796, Leu844, and Thr854. The site-specific multiple ligand docking was performed to dock all 28 4-anilinoquinazoline derivatives and control, dacomitinib, with the specific site of kinase domain of the target protein, and the binding energies of all ligands were obtained. The best potential compounds possessing binding energy greater than that of the control, dacomitinib, and having an RMSD value equal to zero, were selected for future studies. While comparing the binding energies of the complexes, we found only 7 potential compounds with a binding affinity greater than that of the control, dacomitinib. These compounds were further allowed to undergo molecular docking using the Autodock vina module of the UCSF Chimera molecular modelling system to identify the top three potential lead-like compounds. The list of the top 7 compounds is mentioned in the table, along with their binding energies obtained by using PyRx and Autodock vina module of Chimera (Table 3).
3.5. Molecular Docking Studies
We utilized the AutoDock vina module of UCSF Chimera to perform molecular docking of the top 7 best 4-anilinoquinozoline derivatives and the standard control dacomitinib with the ligand-specific site of the kinase domain of mutant T790M EFGR (4I24). The AMBERff14SB force field was utilized to generate ligand interaction poses of the protein during the process of a molecular docking simulation. For each ligand complex, 10 different conformations were generated and the one which possessed the highest binding energy and zero rmsd value was selected. Using this approach, we analysed the binding energy of the docking complex and found three top leads with binding energy greater than that of the standard control, dacomitinib. The selected three top potential leads include 122417707 (AQ245), 122417730 (AQ236), and 122417707 (AQ242) with the binding energy of -9.8 kcal/mol, -9.8 kcal/mol, and -9.6 kcal/mol, respectively. Whereas, the binding energy of the control drug, dacomitinib, was found to be -9.5kcal/mol, which was less than that of the selected top leads. It is noteworthy that compound AQ242 established a hydrogen bond with Cys797 residue, which is involved in EGFR resistance to third-generation tyrosine kinase inhibitors. Therefore, this compound has the potential to be used as the fourth-generation EGFR inhibitor to target EGFRC797S activity. Hence, based on the achieved binding energies, it can be concluded that the selected leads may have the potential to inhibit the activity of the mutant T790MEGFR and C797S, which, in turn, may have the ability to target tumor angiogenesis phenomenon. The binding score of the top three ligands and control dacomitinib are mentioned in Table 4.
S.No. | Compounds | PyRx Docking Score | **UCSF Chimera Docking Score |
---|---|---|---|
1 | AQ245 (CID:122417700) |
-9.9 | -9.8 |
2 | AQ229 (CID:122417770) |
-9.9 | -9.4 |
3 | AQ242 (CID:122417707) |
-9.7 | -9.6 |
4 | AQ236 (CID:122417730) |
-9.7 | -9.8 |
5 | AQ231 (CID:122417767) |
-9.5 | -9.4 |
6 | AQ221 (CID:122417840) |
-9.5 | -9.5 |
7 | AQ46 (CID:71543088) |
-9.4 | -9.1 |
8 | Dacomitinib | -9.2 | -9.5 |
S.No. | Compound | *Binding Energy (kcal/mol) | **Hydrogen Bond Interactions Involved | ***Binding Site Residues |
---|---|---|---|---|
1 | AQ245 (CID:122417700) |
-9.8 | A:LYS745:H - A:LEU788:O A:LYS745:HZ1 - A:ASP855:OD1 A:MET790:H - A:ALA743:O A:GLY796:H - A:MET793:O A:ASN842:HD22 - A:ASP855:OD2 |
Unk0, Leu718, Gly719, Val726 Ala743, Ile744, Lys745, Leu788 Ile789, Met790,Leu792, et793, Gly796, Cys797, Asp800, Leu844, Thr854 Asp855 |
2 | AQ236 (CID:122417730) |
-9.8 | A:LYS745:H - A:LEU788:O A:LYS745:HZ1 -A:ASP855:OD1 A:MET790:H - A:ALA743:O A:MET793:H -:UNK0:N5 A:GLY796:H - A:MET793:O A:ASN842:HD22 A:ASP855:OD2 A:ASP855:H - A:THR854:OG1 |
Leu718,Gly719,Val726,Ala743 Ile744,Lys745,Leu788,Ile789 Met790,Leu792,Met793, Pro794,Phe795,Gly796,Cys797 Leu844,Thr854,Asp855,Unk0 |
3 | AQ242 (CID:122417707) |
-9.6 | A:LYS745:H - A:LEU788:O A:LYS745:HZ1 - A:ASP855:OD1 A:MET790:H - A:ALA743:O A:GLY796:H - A:MET793:O A:CYS797:H -:UNK0:O3 A:ASP855:H - A:THR854:OG1 |
Leu718,Gly719,Val726,Ala743 Ile744,Lys745,Leu788,Met790 Leu792,Met793,Gly796,Cys797 Leu844,Thr854,Asp855,Leu858 Unk0 |
Rreference drug | Dacomitinib | -9.5 | A:LYS745:H - A:LEU788:O A:LYS745:HZ1 - A:ASP855:OD1 A:MET790:H - A:ALA743:O A:GLY796:H - A:MET793:O A:CYS797:H -:UNK0:O3 A:ASP855:H - A:THR854:OG1 |
Leu718, Gly719,Val726, Ala743,Ile744,Lys745, Leu788,Met790,Leu792 Met793,Gly796,Cys797 Leu844,Thr854,Asp855 Leu858,Unk0 |
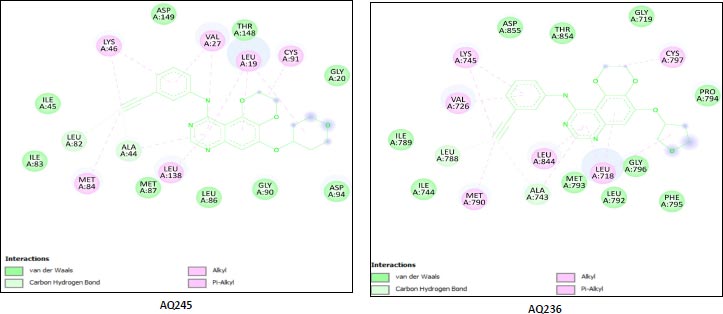
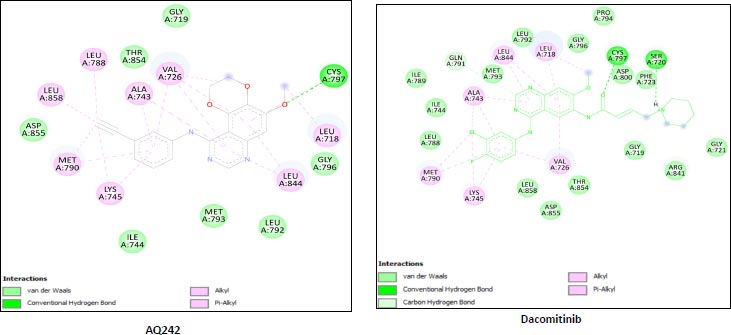
3.6. Cardio Toxicity Prediction using predhERG
We used the predhERG online server to predict and compare the cardiotoxicity profile of the top three potential leads to the control drug dacomitinib [26]. It is important to establish the safety of the drug before it can be administered [27]. The predicted cardiotoxicity results of our top leads in relation to dacomitinib showed that our top leads exhibited high potency towards the inhibition of the hERG enzyme than the control drug dacomitinib. This high level of toxicity was due to the presence of atomic sites possessing high affinity towards hERG blockage, which afterward could be modified to generate compounds that might have null or least cardiotoxicity profiles (Table 5).
3.7. Metabolic Reactivity Prediction via SMARTCyp 3.0 Server
SMARTCyp 3.0 is a sophisticated server that is deployed to predict the metabolic reactivity of the atoms of chemical compounds with cytochrome p450 drug-metabolizing enzymes. It is important to predict the drug metabolism of the compounds so that a safe drug can be developed by reducing its adverse side effects if present. We deployed the SMARTCyp server to predict the drug metabolism of p450 cytochrome isoforms like 3A4, 2D6, and 2C9 towards our selected compounds. We used SMILES notation of the compounds as an input to the server and the output was given in the tabulated form as a result. From the tabular data, we observed that the selected leads and the reference drug have energies <999, and for the selected compounds, almost for all Cyp p450 isoforms, the value for span2end was less than 4 and the similarity was found to be between 0 to 1, indicating that the selected compounds have the acceptable probability of having the metabolic reactivity towards cytochrome isoforms. In the control drug, dacomitinib, atomic sites C.29, C.27, and C.26 have a higher probability for the sites of metabolism for the 3A4 cytochrome isoform. The atomic sites C.1, C.18, and N.13 were the high metabolic reactive sites for the 2D6 isoform, whereas the atomic sites C.29, C.27, and C.31 were found to possess a higher probability of being metabolized by the 2C9 isoforms of p450 cytochrome. In compound AQ245, the reactive atomic sites for 3A4 isoforms include C.27, C.22, and C.21. The atomic sites C.27, C.8, and C.25 have the probability of being metabolized by isoforms 2D6 and 2C9 of cytochrome p450. In the compound AQ236, C.27, C.29, and C.21 atomic sites have a higher probability of being metabolized by the 3A4 isoform of p450 cytochrome. The atomic sites, C.27, C.29, and C.8, have a higher probability of being metabolized by cytochrome 2D6 and 2C9 isoforms. Similarly, in compound AQ242, the atoms C.1, C.24, C.23, and C.17 (by 2D6 only) have a higher probability of being metabolized by cytochrome isoforms 3A4, 2D6, and 2C9. By analyzing the above data about the metabolic reactivity, it can be concluded that C.27, C.29, C.8, C.1, C.23, and C.24 are the atomic sites present in all selected compounds that are most likely to be metabolized by three isoforms of cytochrome p450 (Table 6).
Compound Name | Prediction/Potency | Confidence | Applicability Domain | Probability Map |
---|---|---|---|---|
AQ245 122417707 |
Potential cardiotoxic (+) | 60% | No Value=0.25 and Limit = 0.26 |
![]() |
Weak or moderate | 70% | |||
AQ236 122417730 |
Potential cardiotoxic (+) | 60% | Yes Value = 0.26 and Limit = 0.26 |
![]() |
Weak or moderate | 70% | |||
AQ242 122417707 |
Potential cardiotoxic (+) | 60% | Yes Value=0.27 and Limit = 0.26 |
![]() |
Weak or moderate | 70% | |||
Dacomitinib | Non-cardiotoxic | 50% | Yes Value=0.32 and Limit = 0.26 |
![]() |
Not applicable | Not applicable |
Compound Name | Cyp Type | Atoms | Score | Energy | 2D SASA | Span2end | Relative Span | Similarity |
---|---|---|---|---|---|---|---|---|
CONTROL (Dacomitinib) | 3A4 | C.29 | 32.7 | 41.1 | 32.9 | 2 | 0.9 | 0.7 |
C.27 | 33.9 | 41.1 | 23.9 | 4 | 0.8 | 0.4 | ||
C.26 | 45.9 | 52.4 | 18.9 | 5 | 0.7 | 0.3 | ||
2D6 | C.1 | 86.5 | 62.2 | 64.3 | 6 | 9 | 1.0 | |
C.18 | 96.3 | 84.1 | 30.0 | 2 | 13 | 0.7 | ||
N.13 | 98.4 | 72.0 | 10.4 | 5 | 10 | 0.7 | ||
2C9 | C.29 | 51.6 | 41.1 | 32.9 | 2 | 0 | 0.7 | |
C.27 | 63.8 | 41.1 | 23.9 | 4 | 0 | 0.4 | ||
C.31 | 74.4 | 75.9 | 37.7 | 0 | 0 | 1.0 | ||
AQ245 122417707 |
3A4 | C.27 | 53.2 | 62.2 | 37.8 | 1 | 0.9 | 0.3 |
C.22 | 54.8 | 62.2 | 38.6 | 4 | 0.7 | 0.3 | ||
C.21 | 55.3 | 62.2 | 38.6 | 5 | 0.7 | 0.3 | ||
2D6 | C.27 | 67.4 | 62.2 | 37.8 | 1 | 0 | 0.3 | |
C.8 | 73.6 | 68.2 | 32.0 | 1 | 0 | 1.0 | ||
C.25 | 81.9 | 62.2 | 10.1 | 3 | 0 | 0.3 | ||
2C9 | C.27 | 66.6 | 62.2 | 37.8 | 1 | 0 | 0.3 | |
C.8 | 72.8 | 68.2 | 32.0 | 1 | 0 | 1.0 | ||
C.25 | 79.5 | 62.2 | 10.1 | 3 | 0 | 0.3 | ||
AQ236 122417730 |
3A4 | C.27 | 52.5 | 62.2 | 41.7 | 0 | 1.0 | 0.3 |
C.29 | 53.3 | 62.2 | 37.9 | 1 | 0.9 | 0.3 | ||
C.22 | 54.4 | 62.2 | 38.6 | 3 | 0.8 | 0.3 | ||
2D6 | C.27 | 60.5 | 62.2 | 41.7 | 0 | 0 | 0.3 | |
C.29 | 67.4 | 62.2 | 37.9 | 1 | 0 | 0.3 | ||
C.8 | 73.6 | 68.2 | 32.0 | 1 | 0 | 1.0 | ||
2C9 | C.27 | 60.5 | 62.2 | 41.7 | 0 | 0 | 0.3 | |
C.29 | 66.6 | 62.2 | 37.9 | 1 | 0 | 0.3 | ||
C.8 | 68.2 | 68.2 | 3.0 | 1 | 0 | 1.0 | ||
AQ242 122417707 |
3A4 | C.1 | 51.6 | 62.2 | 64.3 | 0 | 1.0 | 1.0 |
C.24 | 53.3 | 62.2 | 38.6 | 1 | 0.9 | 0.3 | ||
C.23 | 54.0 | 62.2 | 38.6 | 2 | 0.8 | 0.3 | ||
2D6 | C.1 | 59.6 | 62.2 | 64.3 | 0 | 0 | 1.0 | |
C.24 | 67.4 | 62.2 | 38.6 | 1 | 0 | 0.3 | ||
C.17 | 73.6 | 68.2 | 32.0 | 1 | 0 | 1.0 | ||
2C9 | C.1 | 59.6 | 62.2 | 64.3 | 0 | 0 | 1.0 | |
C24 | 66.6 | 62.2 | 38.6 | 1 | 0 | 0.3 | ||
C.23 | 72.5 | 62.2 | 38.6 | 2 | 0 | 0.3 |
4. DISCUSSION
The EGFR has a critical role in the process of tumor angiogenesis, a phenomenon responsible for the growth, proliferation, and development of various cancers like NSCLC, glioblastoma, head and neck cancer, cervical cancer, and breast cancer. The kinase domain of EGFR tyrosine kinase is a sensitive region that is susceptible to many mutations like L858R, T790M, C797S, etc. In the treatment with first-generation EGFR inhibitors like erlotinib and gefitinib, cancer has developed secondary resistance due to the development of secondary T790M mutations. It was proposed that T790M mutations do not block the binding of the inhibitors, but later on, it was found that the T790M mutation restores ATP binding affinity of the kinase domain of EGFR monomers. Therefore, the first-generation tyrosine kinase inhibitors were not able to bind to T790M mutated EGFR. The second-generation 4-anilinoquinazoline-based EGFR inhibitors like dacomitinib and afatinib have shown excellent binding potency with mutant EGFRT790M but exhibit serious side effects that demand an urgent solution in terms of a new therapeutic strategy. Therefore, our research objective was to identify novel 4-anilinoquinazoline derivatives that may have a good therapeutic tendency and the least side effects. In the present era, structure-based virtual screening, pharmacokinetics, drug-likeness, molecular docking, and metabolic reactivity-based drug designing strategies are widely used to identify novel chemical compounds that can provide cancer treatment options by inhibiting the activity of the kinase domain of EGFR tyrosine kinase. To identify a new 4-anilinoquinazoline derivative, we screened out the PubChem database to retrieve 1000 such derivatives that were further allowed to pass through Lipinski’s and ADMET filters. Then, a total of 28 drug-like compounds possessing good pharmacokinetic profiles were identified. The screened 28 compounds and control dacomitinib were then docked with the active site residues of the kinase domain of EGFRT790M by using PyRx virtual screening tool. We selected the top seven compounds possessing higher binding affinity than that of control, dacomitinib, and utilized the UCSF Chimera tool to perform docking to cross-check the docking results. The top three compounds, 122417707 (AQ245), 122417730 (AQ236), and 122417707 (AQ242), were selected for further study as they possess higher binding affinity than the control, dacomitinib. Moreover, we performed metabolic reactivity prediction and cardiotoxicity prediction of the selected top three leads, which is an important step in drug discovery and development. The results we obtained for the selected compounds demonstrated that the selected compounds possess good binding affinity, pharmacokinetic profile, and drug-likeness features. Such features indicate that our selected compounds may possess the ability to target and inhibit the activity of the kinase domain of T790M/C797S mutant EGFR (PDB ID: 4I24), thereby inhibiting tumor angiogenesis.
CONCLUSION
The inhibition of the biological activity of mutant EGFR (T790M) is essential to inhibit the tumor angiogenic signaling pathways responsible for tumor growth, development, progression, and metastasis [28]. With the use of ADME, toxicity prediction, and molecular docking tools, we were capable of identifying the 4-anilinoquinazoline plant phytochemical derivatives from PubChem-screened compounds. Based on the predicted ADME toxicity profile and molecular docking results, we may suggest that the top three identified potential 4-anilinoquinazoline leads may possess the potential to inhibit the activities of T790M mutant EGFR tyrosine kinase and thereby can be used to inhibit the phenomenon of tumor angiogenesis to treat different kinds of cancers [29, 30]. Furthermore, the anti-angiogenic effect of the top three compounds, 122417707 (AQ245), 122417730 (AQ236), and 122417707 (AQ242), can be validated through in vitro and in vivo studies in the future. The wet lab validation will provide us with detailed information regarding the therapeutic efficacy and safety of the selected lead phytochemicals. Moreover, our selected 4-anilinoquinazoline derivatives may or may not work without cetuximab to inhibit T790M/C797S mutant EGFR, which can also be validated in the future via in vitro and in vivo studies.
LIST OF ABBREVIATIONS
MMPs | = Matrix Metalloproteins |
RTKs | = Receptor Tyrosine Kinases |
EGFR | = Epidermal Growth Factor Receptor |
ETHICS APPROVAL AND CONSENT TO PARTICIPATE
Not applicable.
HUMAN AND ANIMAL RIGHTS
In our research work, no humans or animals were used.
CONSENT FOR PUBLICATION
Not applicable.
AVAILABILITY OF DATA AND MATERIALS
The data and supportive information are available within the article.
FUNDING
None.
CONFLICT OF INTEREST
Salman Akhtar is the associate editorial board member of The Open Bioinformatics Journal.
ACKNOWLEDGEMENTS
The authors would like to thank Integral University, Lucknow, for providing the facilities to carry out this research work, with Manuscript Communication Number: IU/R&D/2022-MCN0001425.